A data lakehouse, or governed data lake, is a data platform that combines the cost-effectiveness and flexibility of a data lake with the governance and query capabilities of a data warehouse. With the use of open table formats and data catalogs, data lakehouses replicate the SQL-based functionality of data warehouses while ensuring visibility into and control of the tabular data stored on the data lake.
The data lakehouse is a powerful central repository of data that supports all manner of analytic and operational uses of data, ranging from reporting, business intelligence and streaming to machine learning and artificial intelligence.
Data lakehouse architecture
Five key layers make up a data lakehouse architecture.
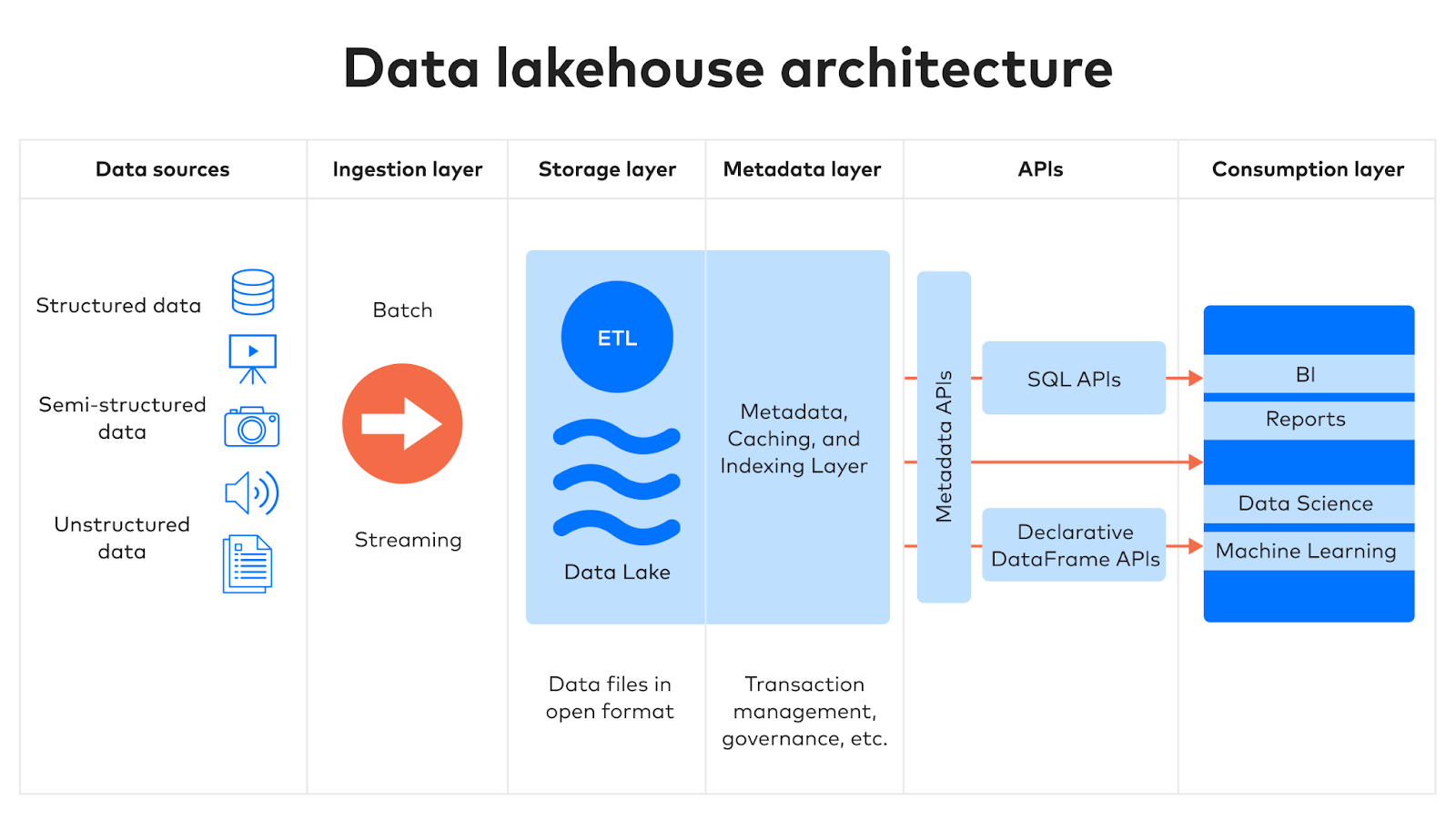
1. Ingestion
In the first layer, data from multiple sources is collected and delivered to the storage layer. This data can be pulled from either internal or external sources, such as:
- Databases, including both relational database management systems (RDBMSs) and semi-structured NoSQL databases
- Enterprise resource planning (ERP), customer relationship management (CRM), and other Software-as-a-Service (SaaS) applications
- Event streams
- Files
The Fivetran Managed Data Lake Service is purpose-designed to facilitate this step. In addition to moving data from source to destination, the Fivetran pipeline normalizes, compacts and deduplicates data while converting it to an open table format.
2. Storage
Data lakehouses use open table formats to store structured and semi-structured data, as well as raw files for unstructured data. Like conventional data lakes, data lakehouses offer scalability and flexibility, decoupling storage from compute and enabling a high degree of modularity.
Lakehouses keep schemas of structured and semi-structured data sets in the metadata layer, allowing data teams to observe and control the contents of the lakehouse, making governance easier.
3. Metadata
Metadata is data that contains information about other pieces of data. A data lakehouse’s metadata layer is the key advantage it has over ungoverned data lakes.
The metadata layer houses a catalog that provides metadata for every object in the lake storage. It also enables users to implement features such as ACID transactions, indexing, caching and data versioning.
The metadata layer also allows users to implement data warehouse schema architectures, like snowflake or star schemas and improve schema management. Auditing and data governance can be done directly on the data lake, enhancing data integrity and building trust in the data and its derivative products.
4. APIs
SQL and DataFrame APIs enable analysts, data scientists and other users to access and query data using their preferred languages. Data professionals are likely to prefer SQL for simpler transformations and reporting while using Python, R, Scala or other languages for more complex manipulations of data.
5. Consumption
Business intelligence platforms and data science applications of all kinds sit in the consumption layer, drawing transformed, analytics-ready data from the lake via the API to produce reports, dashboards and data products, like machine learning and artificial intelligence, of all kinds.
Key features and advantages of a data lakehouse
A data lakehouse combines the capabilities of both data lakes and data warehouses, including the following key features:
- ACID transactions support: Data lakehouses enable ACID transactions, normally associated with data warehouses, to ensure consistency as multiple parties concurrently read and write data.
- BI support: With ACID support and SQL query engines, analysts can directly connect data lakehouses with business intelligence platforms.
- Open storage formats: Data lakehouses use open storage formats, which a data team can combine with their compute engine of choice. You’re not locked into one vendor’s monolithic data analytics architecture.
- Schema and governance capabilities: Data lakehouses support schema-on-read, in which the software accessing the data determines its structure on the fly. A data lakehouse supports schemas for structured data and implements schema enforcement to ensure that data uploaded to a table matches the schema.
- Support for diverse data types and workloads: Data lakehouses can hold both structured and unstructured data, so aside from handling relational data you can use them to store, transform and analyze images, video, audio and text, as well as semi-structured data like JSON files.
- Decoupled storage and compute: Storage resources are decoupled from compute resources. This gives you the modularity to control costs and scale either one separately to meet the needs of your workloads, whether they be for machine learning, business intelligence and analytics, or data science.
These features offer data teams the following benefits and advantages:
- Scalability: Data lakehouses are underpinned by commodity cloud storage, which means they have high scalability.
- Improved data management: By storing diverse data, data lakehouses can support all data use cases, ranging from reporting to predictive modeling to generative AI.
- Streamlined data architecture: The data lakehouse simplifies data architecture by eliminating the need to use a data lake as a staging area for a data warehouse, as well as eliminating the need to maintain a separate data warehouse for analytics and data lake for streaming operations.
- Lower costs: The streamlined architecture also lowers costs.
- Eliminate redundant data: Since data is unified in a lakehouse, redundant copies of data are no longer necessary. This reduces storage requirements and makes governance easier.
Data lakehouses have a bright future
The data lakehouse was first announced by Databricks in 2017, eventually becoming an open-source project aimed at bringing reliability to data lakes. In the years since, other major cloud platform providers have begun to offer the same architecture.
Data lakehouses are ideal for the following use cases:
- An organization’s data needs are anticipated to continue growing in scale, volume and complexity, making the cost advantages and flexibility offered by using a data lake for storage more meaningful.
- An organization wants to simplify its data architecture and eliminate redundancies for cost control and better governance.
- Innovative use cases, such as those involving generative AI, make it ever more valuable to have all of an organization’s data in one place.
The Fivetran Managed Data Lake Service makes data integration and data movement into the data lakehouse simple and reliable. Sign up today for a free trial.