The Essentials of AI Data Preparation
The Essentials of AI Data Preparation
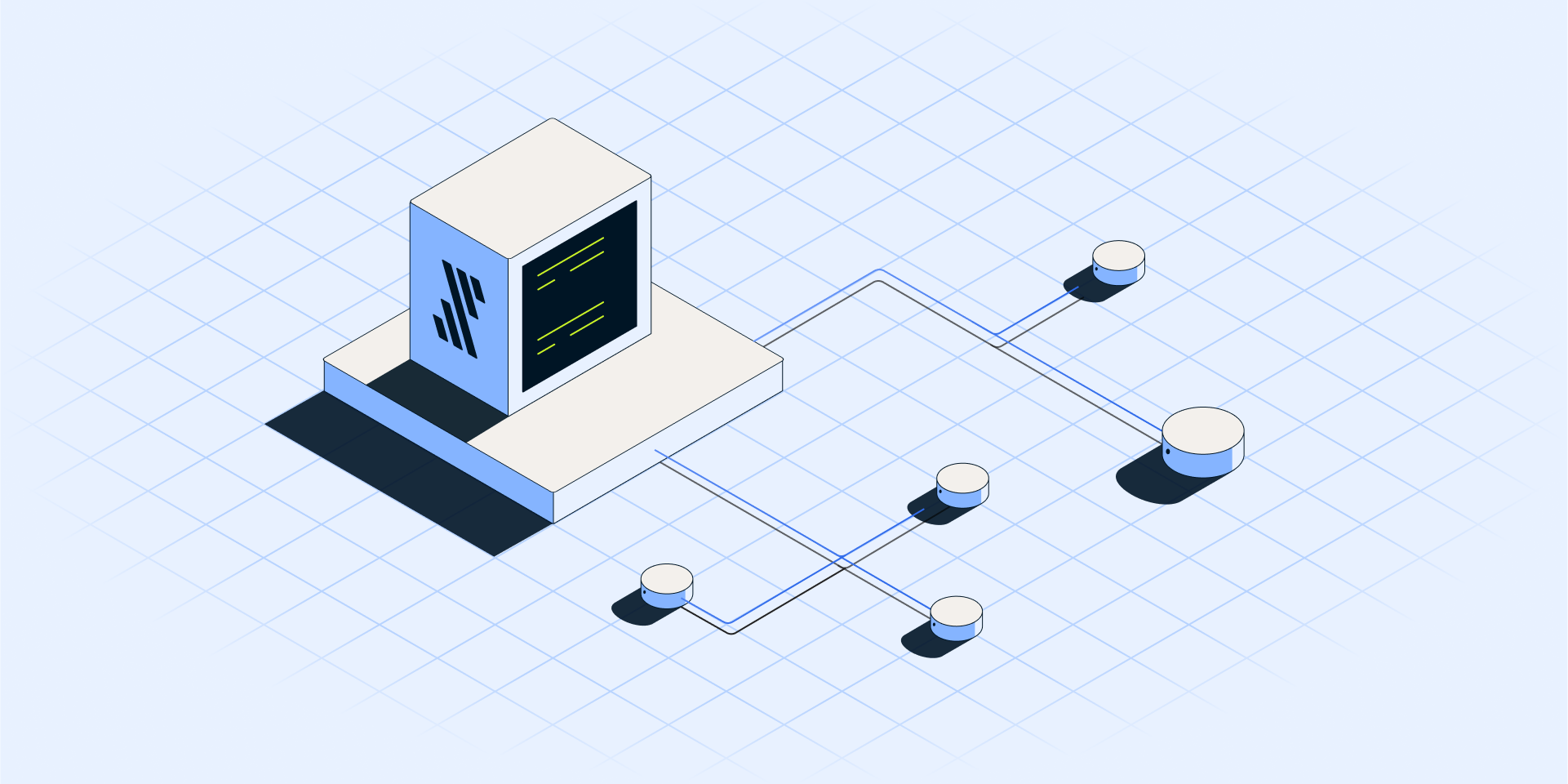
A successful AI strategy requires integrating diverse data from many first- and third-party data sources. It’s a challenging task due to variations in data formats, quality, and volumes. Imagine a complex puzzle with each piece representing a different type of data. While an AI system doesn’t NEED all the pieces assembled correctly to function, it's much harder to work without all that data in the right place and format/orientation.
The data must also be meticulously prepared to be accurate, relevant, and compliant. It’s an ongoing process that ensures AI systems remain accurate and effective over time, adapting to new data and evolving requirements. It's a demanding task, but essential. Developing an effective data preparation process is key to staying abreast of the ever-increasing complexity and volume of data.
What is data preparation?
Meticulous data preparation allows AI systems to gain the clarity and precision necessary to excel in their tasks. Undertaking this process involves cleaning, structuring, and enriching raw data to make it suitable for analysis. Just as a chef carefully prepares ingredients before cooking, data must be meticulously prepared to ensure the best results in AI applications.
The initial phase of data preparation revolves around identifying and rectifying any inaccuracies or inconsistencies present in the raw data. Ensuring the data is cleansed of such errors is crucial, as even minor inaccuracies can significantly impact the performance of AI models.
Another essential aspect of data preparation is ensuring that the data is in a format that is readily accessible and usable by AI systems. Often, this process requires converting data into standardized formats and arranging it to meet the specific requirements of AI algorithms.
Automated tools like Fivetran streamline the data preparation process. They ensure data consistency regardless of the variety and complexity of data sources. Plus, they drastically reduce the time and effort needed to prepare data. Faster and simpler data preparation allows businesses to derive insights and value from their data. The journey from raw data to AI-ready information then becomes faster and more efficient.
Data preparation for AI
While the process of data preparation is complex and multifaceted, it's the foundation upon which the success of AI hinges. High-quality input data ensures that the resulting models are accurate and reliable.
H3: Consequences of inadequate data preparation in AI
Inadequate data preparation can have far-reaching consequences. For instance, in February 2024 Air Canada's GenAI chatbot misled customers about discounts for bereaved families, contradicting the company's actual policy. This incident led to customer distress and legal challenges, highlighting the critical need for accurate data and programming in AI systems, especially in sensitive customer service areas.
The recall of Cruise's entire fleet of autonomous vehicles in November 2023, following a serious accident attributed to a flaw in the autonomous driving software, serves as a critical case study. This incident not only highlights the devastating consequences of insufficient data preparation and quality assurance in AI but also stresses the heightened stakes in sectors like autonomous transportation, where public safety is directly impacted.
The impact of data quality on AI outcomes
Business consequences can range from customer dissatisfaction to severe safety risks. Focusing on data preparation is not just a technical necessity but a critical factor in ensuring responsible and reliable AI deployment. Moreover, securing high-quality data is a multi-step focus:
- Data aggregation: The method of gathering data from various sources. The data can come from internal systems, external databases, social media, and more.
- Data cleaning: The practice of removing inaccuracies and inconsistencies before input. Techniques used here include correcting errors, filling in missing values, and removing duplicates.
- Data transformation: The act of converting data into a format suitable for analysis. It typically entails normalization, aggregation, and applying techniques like scaling or encoding to suit AI algorithm requirements.
- Data integration: The method of combining data from different sources. Involves the alignment of data formats and consolidation of datasets, it resolves conflicts to ensure seamless integration.
- Data reduction: The technique of simplifying data without losing its essence. Techniques like dimensionality reduction and feature selection focus on the most relevant data aspects for AI.
The objective is to create a refined, AI-ready dataset, which is crucial for the efficient and effective functioning of AI algorithms.
Challenges in data preparation
Preparing data for AI is a critical process that directly influences the effectiveness of the outcomes. Missteps in this phase often result in AI systems producing unreliable or skewed results, which can seriously hamper their real-world usefulness and accuracy. Key challenges include:
- Data cleanliness: Data often comes with errors, inconsistencies, or missing values. In a retail setting, this could mean having sales records with incorrect prices or incomplete customer information. Failing to address this data cleanliness issue can lead to faulty AI analyses, resulting in misleading sales forecasts and flawed customer segmentation – ultimately impacting critical business decisions.
- Data relevance and completeness: If data completeness is not maintained, the AI's ability to understand and predict accurately is compromised. In the field of financial market predictions, using a dataset that lacks recent market trends or excludes certain economic indicators can lead to inaccurate or misleading AI-driven forecasts.
- Data diversity: AI systems learn patterns and make decisions based on the data they're fed. Bias or lack of data diversity leads to skewed AI's decisions and predictions. For example, in developing facial recognition software, if the training data is predominantly composed of images from a narrow demographic group, the AI is likely to perform poorly in recognizing faces from other demographic groups. Regularly reviewing and updating the data helps to maintain its diversity and relevance.
- Data structure and format: Data from various sources is typically structured differently, presenting challenges in merging and analyzing it collectively. Data formats like YAML or XML, while standardized, can exhibit significant variations, requiring careful alignment to achieve consistency. Properly aligning disparate data into a common format is crucial to fully realize its potential.
Poor data quality in any of these areas can lead to AI systems that are unreliable, biased, or simply ineffective. A recent Fivetran study revealed that neglecting data correction can have substantial financial consequences. Organizations reported a 6% loss in global annual revenue due to underperforming AI models stemming from inaccurate data.
Clean data, powerful AI. It's that simple.
Data quality and management
Data quality is like high-grade fuel for a car's optimal performance. Superior quality data results in enhanced business decision-making, operational efficiency, and overall competitive advantage. To achieve this level of data quality, especially for AI readiness, the following steps are designed not just to maintain but to elevate data standards:
- Set clear standards: Start by defining what good data looks like for your specific AI applications. For example, a city planning AI application might require access to comprehensive and current city demographic statistics, traffic patterns, and infrastructure data, allowing for precise modeling and forecasting. Review for quality on a continuous basis to ensure the data remains accurate, relevant, and consistent over time.
- Employ audits and updates: Develop robust systems and processes to maintain your data's integrity, including regular audits and updates. In a healthcare setting, regular audits often include cross-checking patient data across various systems, such as electronic health records and medical imaging databases. The goal is to identify and rectify discrepancies, thereby ensuring that AI algorithms used in diagnostic tools or treatment recommendations are based on accurate and current patient information.
- Establish robust data governance policies: Create guidelines for data usage and security. Once these policies are in place, they will help maintain the integrity and confidentiality of your business data. A financial institution implementing stringent data governance can better monitor and control data access, ensuring sensitive financial information remains secure. Regular audits and adherence to international data protection regulations, such as GDPR or HIPAA, further reinforce the integrity and confidentiality of the data, reducing the risk of data breaches and misuse.
- Don’t overlook technology: Modern tools and software automate many aspects of data quality control, from error detection to data cleansing. Consider a marketing analytics company utilizing machine learning tools for data quality control. Its automated systems efficiently detect and correct inconsistencies in customer interaction datasets, enhancing precision and efficiency in data processing. Where appropriate, incorporate these technologies to enhance the precision of your data quality efforts.
Data quality and management in AI requires commitment and the right tools to ensure that the data driving your AI is as good as it can be.
For a healthcare company leveraging AI in patient outcome predictions, the focus on data quality is non-negotiable. Advanced data management tools are employed to meticulously clean and standardize patient data from diverse sources like electronic health records, lab results, and wearable technology. Inaccuracies or inconsistencies in this data could lead to incorrect predictions, potentially affecting patient care. Consequently, a strong commitment to data quality is key to enhancing the accuracy of AI predictions and improving healthcare outcomes.
Empowering AI success with Fivetran
Effective AI implementation hinges on robust processes for data integration and preparation, which guarantees accuracy and consistency across various data sources. The way data is handled and integrated significantly influences how precise and relevant the outcomes of AI are, highlighting the importance of making smart data management practices.
Fivetran has the expertise in data integration that can significantly streamline and optimize the data preparation process. They help businesses create a strong and reliable data foundation, essential for AI initiatives. Their support is invaluable in achieving sophisticated data preparation, which is fundamental in realizing the benefits of AI in today's digital business landscape.
Businesses may find understanding and implementing these concepts can be a challenge. To dive deeper into this topic and unlock actionable insights, we invite you to download our comprehensive ebook, "A Primer for Data Readiness for AI”. This essential guide provides detailed strategies, real-world examples, and practical tips to streamline your data preparation process using AI technologies. Don't miss this opportunity to elevate your data strategies to the next level.
Related posts
Start for free
Join the thousands of companies using Fivetran to centralize and transform their data.