ETL (Extract, Transform and Load) and ELT (Extract, Load and Transform) are data integration methods that dictate how data is transferred from the source to storage.
While ETL is an older method, it is still widely used today and can be ideal in specific scenarios. On the other hand, ELT is a newer method that is focused on flexibility and automation.
Both processes have three main operations:
- Extraction: Pulling data from the original source (such as an app or SaaS platform).
- Transform: Changing the data’s structure so it can be integrated into the target data system.
- Load: Depositing data into a storage system.
The differences arise in the order of these operations. ETL focuses on transformation right after extraction, while ELT extracts and loads data before transformation.
In this article, we cover ELT and ETL in detail, so you can understand how they work and choose the right data integration method. We’ve included a handy table explaining the differences between the two processes.
[CTA_MODULE]
What is ETL?
The acronym “ETL” stands for Extract, Transform, Load. It is a popular data integration method that consists of three main operations:
- Gathering and extracting data
- Loading it into a destination
- Transforming it into models that analysts can use
ETL is the traditional approach to data integration. It was invented in the 1970s and is so ubiquitous that “ETL” is often used interchangeably with data integration.
Under ETL, data pipelines extract data from sources, transform data into data models for analysts to turn into reports and dashboards and then load data into a data warehouse.
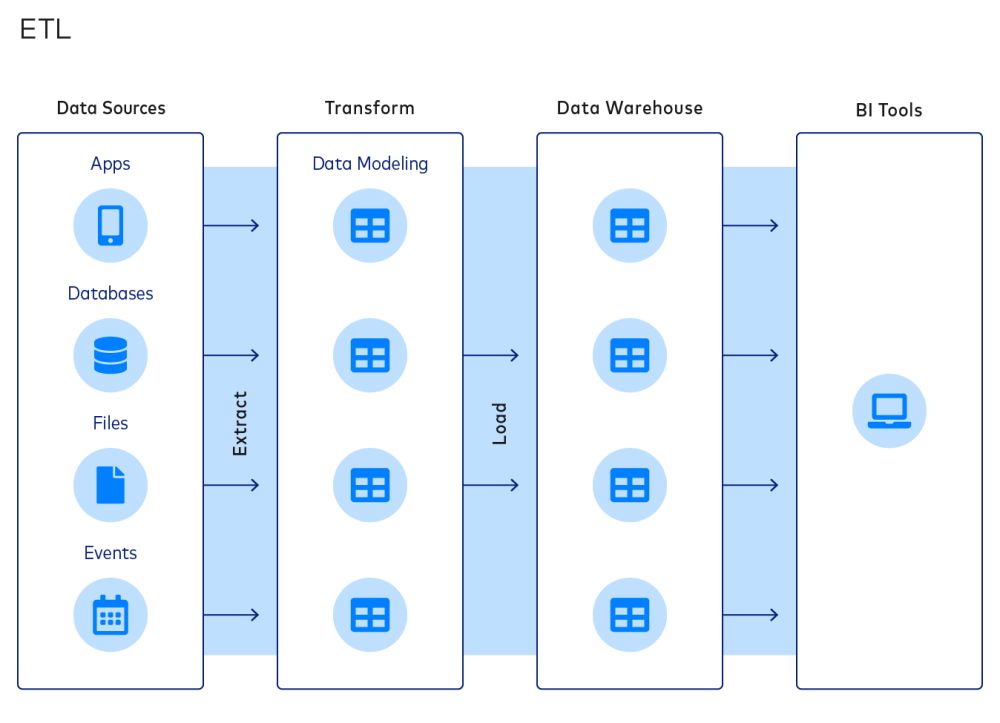
Data transformations typically aggregate or summarize data, shrinking its overall volume. This was crucial when ETL was first devised and most organizations operated under very stringent technological constraints.
Storage, computation and bandwidth were extremely scarce. By transforming before loading, ETL decreases the volume of data stored in the warehouse. This process preserves resources throughout the entire workflow.
The ETL workflow has the following steps:
- Identify data sources
- Scope the exact data analytics needs the project is meant to solve
- Define the data model/schema that the analysts and other end users need
- Build the pipeline
- Conduct analytics work and extract insights
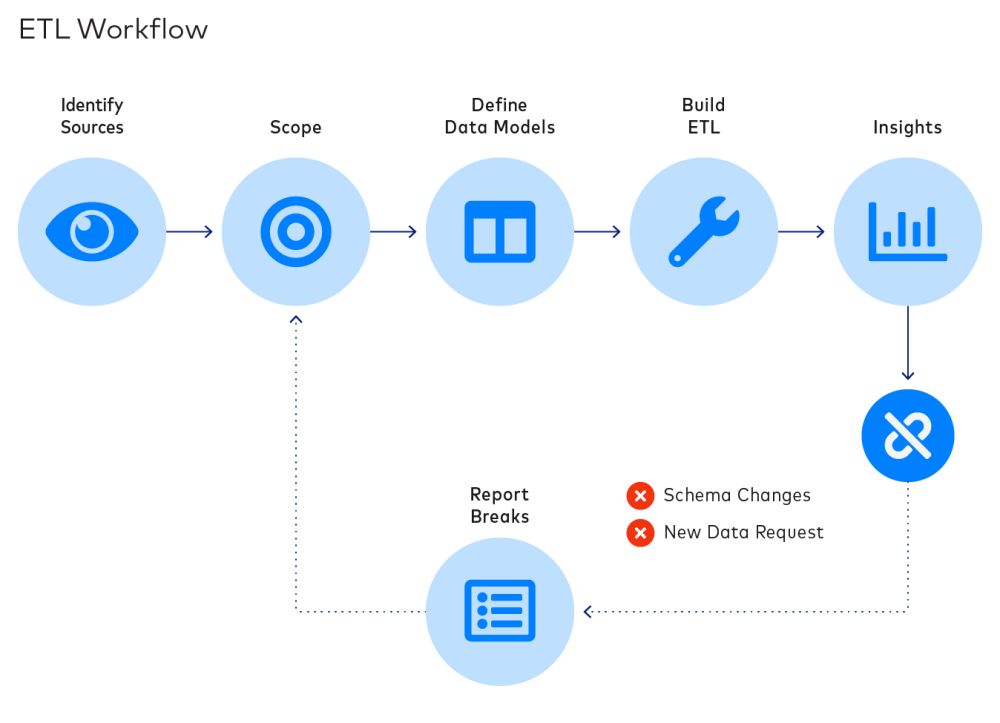
In ETL, extraction and transformation are performed before any data is loaded to a destination. So, both these processes are tied together. Also, because transformations are dictated by the specific needs of analysts, every ETL pipeline is a complicated, custom-built solution.
The complex nature of these pipelines makes scaling very difficult, particularly when adding data sources and models.
Your ETL pipeline’s workflow must be repeated and altered every time these two common conditions occur:
- Changes at the source: When fields are added, deleted or edited at the source, the upstream schemas change and invalidate the code used to transform the raw data into the desired data models.
- New data configurations: When an analyst wants to build a dashboard or report that requires data in a new configuration, the downstream analytics must change. The transformation code must be rewritten to produce new data models.
Any organization that is constantly improving its data literacy will regularly encounter these two conditions.
Since extraction and transformation are codependent, any transformation stoppages will also prevent data from being loaded to the destination, creating downtime.
Using ETL tools for data integration involves the following challenges:
- Constant maintenance – Every time there is a change in the upstream or downstream schemas, the pipeline breaks and an often extensive revision of the ETL software’s code base is required.
- Customization and complexity – Data pipelines not only extract data but perform sophisticated transformations tailored to the specific analytics needs of the end users. This means a great deal of custom code.
- Labor-intensive and expensive – Because the system runs on a complex code base, it requires a team of dedicated data engineers to build and maintain.
These challenges result from the critical tradeoff made under ETL, which is to conserve computation and storage resources at the expense of labor.
Technology trends toward cloud data integration
Intensive labor was acceptable when resources were limited and expensive and the volume and variety of data were insubstantial.
ETL was a product of its time. But, a lot of these constraints are no longer present.
Specifically, the cost of storage has plummeted from nearly $1 million to a matter of cents per gigabyte (a factor of 50 million) over four decades.
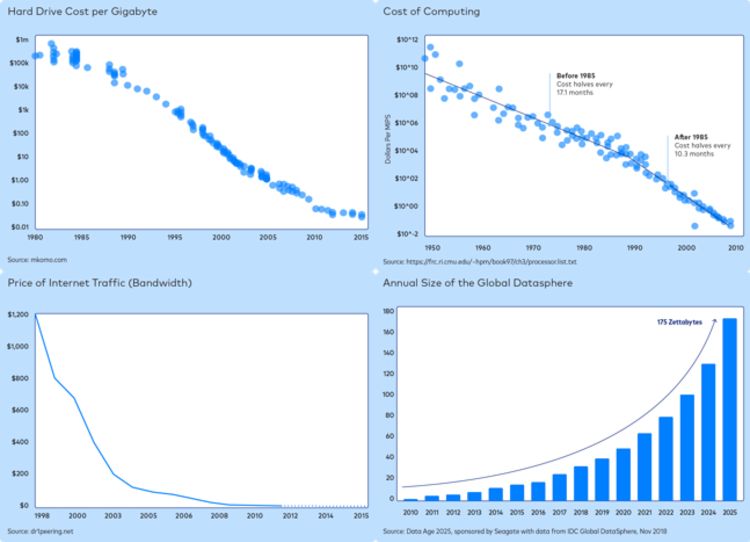
Likewise, computation costs have shrunken by a factor of millions and the cost of internet transit has fallen by a factor of thousands.
These trends have made ETL obsolete for most purposes in two ways:
- The affordability of these critical elements has led to the explosive growth of cloud-based services. As the cloud has grown, the volume, variety and complexity of data have also increased. A brittle, complicated pipeline that integrates a limited volume and data granularity is no longer sufficient.
- Modern data integration technologies have fewer restrictions on the volume of data to be stored and the frequency of queries performed within a warehouse.
These two factors have made it practical to reorder the data integration workflow. Most importantly, organizations can now afford to store untransformed data in data warehouses.
What is ELT?
Extract, Load andTransform (ELT) is a newer data integration process where data is immediately loaded from the source(s) to a destination upon extraction and the transformation step is moved to the end of the workflow.
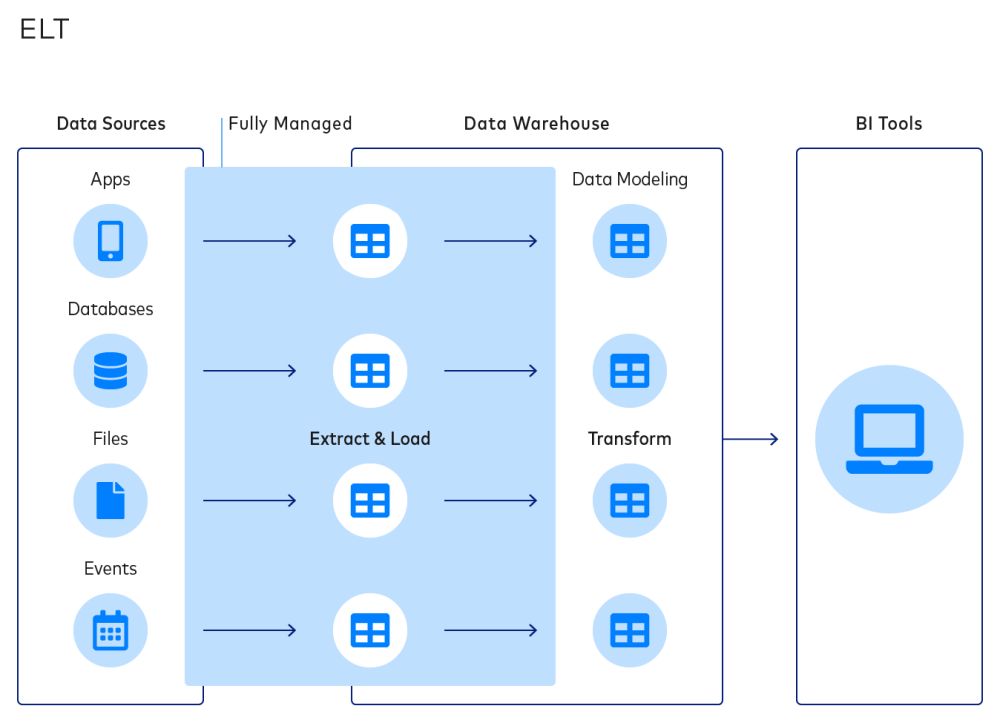
This is a fundamentally different process from ELT. Over the past couple of years, companies in a wide range of industries have made the switch over from ETL due to ELT’s benefits. For one, it uncouples the extraction and transformation processes.
This prevents the two failure states of ETL (i.e., changing upstream schemas and downstream data models) from impacting extraction and loading, leading to a simpler and more robust approach to data integration.
In contrast to ETL, the ELT workflow features a shorter cycle:
- Identify desired data sources
- Perform automated extraction and loading
- Scope the exact analytics needs the project is meant to solve
- Create data models by building transformations
- Conduct actual analytics work and extract insights

The ELT workflow is simpler and more customizable. This is better for analysts that want the flexibility to create tailored data transformations as needed without rebuilds of the data pipeline.
While both ELT and ETL store data in a data warehouse as the end destination, ELT pipelines may also use data lakes to store large-scale unstructured data during the process. These data lakes are managed using a distributed NoSQL data management system or big data platforms.
ELT workflows are easier to customize and are often used for more than business intelligence. Organizations can use them for predictive analytics, real-time data and event streams that drive applications, artificial intelligence and machine learning.
Why ELT is the future
Both ETL and ELT have clear merits, but ELT has six clear advantages that make it future-proof and user-friendly:

1. Simplify data integration
In ELT, the destination can be populated with data directly from the source, with no more than a light cleaning and normalization to ensure data quality and ease of use for analysts.
Analysts and data scientists, whose responsibilities often revolve more around data integration activities than actual analytics, can finally leverage their understanding of business needs and use it for better modeling and analysis.
A simplified data integration solution also streamlines data engineering. It enables engineers to focus on mission-critical projects like optimizing an organization’s data infrastructure or productizing predictive models instead of constructing and maintaining complex data pipelines.
Implementing ELT also means organizations can combine data from different data sets and in various formats. You can also collate structured, unstructured, related or unrelated data.
2. Lower failure rates
ELT workflows move data to its storage destination before it’s transformed. So, extraction and loading processes are independent of transformation. This independence prevents any delays caused during the transformation process.
Although the transformation layer may still fail as upstream schemas or downstream data models change, these failures will not stop data from being loaded into a data warehouse or data lake.
Instead, an organization can continue to extract and load data even as analysts periodically rewrite transformations. Since this data arrives at its destination with minimal alteration, it serves as a comprehensive, up-to-date source of truth.
3. Automate workflows
An organization that combines automation with ELT stands to improve its data integration workflow dramatically.
Since automated extraction and loading returns raw data, it can be used to produce a standardized output. This eliminates the need to constantly build and maintain pipelines featuring custom data models. It also allows derivative products, like templated analytics, to be produced and layered on top of the destination.
The automation of extraction and loading eliminates manual, labor-intensive tasks and frees up analysts to focus on actually analyzing data for insights instead of collecting and preparing it.
4. Easier outsourcing
Since the ELT pipeline can produce standardized outputs and allows for easier changes to the pipeline, it’s easier to outsource your data integration to third parties.
Platforms like Fivetran offer seamless data collection and automatically separate them into columns, rows and tables. Your analysts can get fresh, organized data in minutes. They also have a team of engineers and fully managed connectors to maintain your pipelines for you.
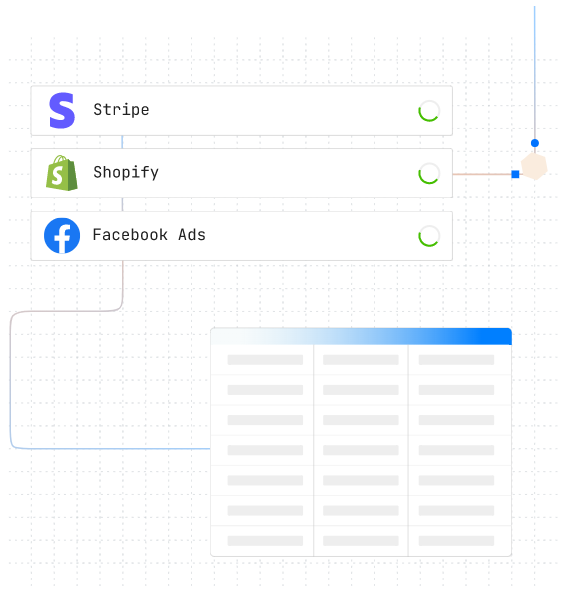
Outsourcing is also a favorable option when it comes to security, privacy and compliance, with Fivetran offering encryption at all stages, the ability to hash sensitive data before it reaches a destination, full control of how data is handled and secure login options.
Using platforms like this to handle your data pipelines saves time and is often more affordable than hiring a team of data engineers.
5. Flexible scaling
Organizational data needs change constantly based on business, market and client relationships. When data processing loads increase, automated platforms that use cloud data warehouses can autoscale within minutes or hours.
In ETL, scaling is much harder and more time-consuming since new hardware needs to be ordered, installed and configured.
Depending on your data needs, you can also scale down your data processing and warehousing. Rather than uninstalling hardware and taking down physical equipment, a cloud-based platform like Fivetran lets you alter your pipelines in minutes.
Scaling is also easier when your data integration platform supports integrations with your data sources.
6. SQL transformation support
In an ELT pipeline, transformations are performed within the data warehouse environment.
There is no longer any need to design transformations through drag-and-drop interfaces, write modifications using scripting languages such as Python or build complex orchestrations between disparate data sources.
Instead, you can write transformations in SQL, the native language of most analysts. This shifts data integration from an IT- or engineer-centric activity to one that analysts can directly and easily own.
Giving analysts the freedom to transform data as they need and gain valuable insights that can drive key business decisions, improve problem-solving and better address customer needs.
ETL vs ELT : Differences and Comparison
The following table summarizes the differences between ETL vs ELT:
There are some cases where ETL may still be preferable over ELT. These specifically include cases where:
- The desired data models are well-known and unlikely to change quickly. This is especially the case when an organization also builds and maintains systems that generate source data.
- There are stringent security and regulatory compliance requirements concerning the data and it absolutely cannot be stored in any location that might be compromised.
These conditions tend to be characteristic of very large enterprises and organizations that specialize in software-as-a-service products.
In such cases, it may make sense to use ELT for data integration with third-party SaaS products while retaining ETL to integrate in-house, proprietary data sources.
Give ELT a try at no cost
ETL and ELT are both solid data integration processes with their own ideal use cases. However, today ELT is clearly a better option for the vast majority of organizations. Want easier and faster access to their business and customer data? ELT is how you achieve that.
ELT enables automation, outsourcing and integrations with third parties. These functionalities save organizations time and money, allowing the analysts to derive the right insights.
A cloud-based data integration platform like Fivetran is the perfect solution to implement secure data pipelines across industries. Our tool helps you gather data from all the databases and apps you use and collect it in a centralized destination.
If you’re still unsure, there’s nothing like experiencing it with your own eyes. Fivetran’s Free Plan, which includes dbt Core-compatible data models, enable you to try ELT completely free.
[CTA_MODULE]