Data movement: The ultimate guide
Data movement: The ultimate guide
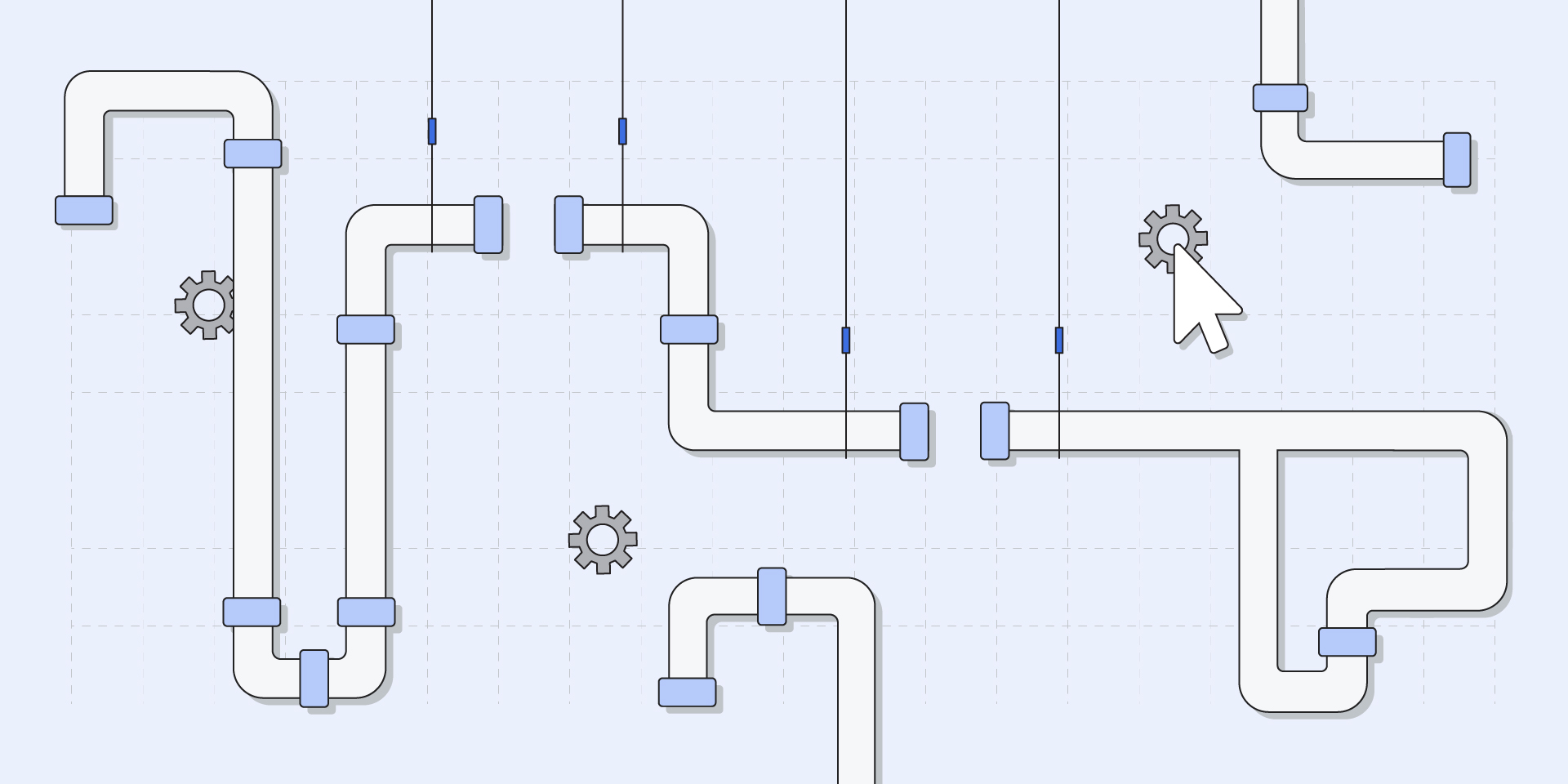
The IT landscape and application landscape of your company is always evolving with a variety of databases and data warehouses being leveraged in your organization. This means that in order to move data between your systems without affecting the performance of your sources, you need effective and secure data movement solutions. Due to its enormous power and myriad top benefits, data mobility is currently an essential core capability for any given organization. Data movement refers to the ability to transfer data through a variety of methods from one source or system in your company to another destination.
In this article, you will understand the need for data movement and explore the different methods that are widely used to move your data. Furthermore, you will gain insights into one of the best data movement tools and why it is so popular in the market. Before you jump onto that part, let’s get acquainted with the basics of data movement.
[CTA_MODULE]
What is data movement?
Transferring data from one place to another is referred to as data movement. For the purposes of data migration and data warehousing, this can be accomplished via techniques like extract, transformation, load (ETL), extract, load, transform (ELT), data replication, and change data capture (CDC). The following section will go into more detail about these techniques.
Data movement, in all of its forms, is an enabling technology rather than a standalone solution. For example, it is used to populate data warehouses, exchange data with business partners and between applications, provide high availability, assist data preparation, and, in the case of streaming platforms, serve as the foundation for implementing machine learning and analytics in-stream.
What are the types of data movement?
Data movement is made feasible by a number of different strategies, and the one you use will depend on how you plan to store and use the data. The following are some of these methods:
1) Extract, transform, load (ETL)
With this method, data is extracted from the source, modified to fit the structure of the destination, and loaded into the destination. Relational data warehouses need data transformations to maintain rigorous schema and data quality before loading to the data destination such as data warehouse, which makes ETL a perfect choice for them.
This approach is often used when the datasets are small and the metrics that matter to the business are clear. Before the data reaches its final destination, ETL transforms it. ETL enables businesses to ensure compliance when they are subject to data privacy laws like GDPR by removing, masking, or encrypting sensitive data before it is loaded into the data warehouse. Since transformation takes time, ETL is not recommended for processing large amounts of data. The data storage does not provide access to information as rapidly as ELT as data must be transformed in a staging area before it is loaded.
2) Extract, load, transform (ELT)
The different order of processes is the most prominent differentiator between ETL and ELT. ELT (Extract, Load, Transform) exports or copies the data from the sources, but instead of loading the raw data to a staging area for transformation, it loads the data straight into the destination data storage to undergo any necessary transformations. A vast historical archive for creating business intelligence is created by the ELT's raw data retention. In order to create new transformations using extensive datasets when goals and tactics change, BI teams can re-query raw data.
ELT is especially helpful for large, unstructured datasets since it allows for the direct loading of data in the storage. This approach works best when you're feeding data to a data lake, which collects massive amounts of data to be sorted later. The data can then be transformed as needed rather than all at once. Although it speeds up loading, access after the transmission is slowed. As ELT requires less advanced planning for data extraction and storage, it can be more suitable for big data management.
The data transformations in ELT, which might be labour and resource-intensive, are handled by the destination system. Systems that are unable to manage such transformations may find this to be a limitation. As the data is not cleaned, altered, or anonymized before loading, ELT may be less secure than ETL and it calls for more rigorous security practices.
3) Reverse ETL
As organizations switch their architecture from ETL to ELT, the data warehouse becomes the only source of truth for all data. Thus, a platform that unifies warehouses with software is important. Reverse ETL acts as a bridge that transfers data from your data warehouse into software applications like CRM, analytics, and marketing.
Reverse ETL enables real-time access to and availability of unused data from data warehouses in CRMs and other SaaS systems. Data silos dissolve as a result, and you are relieved of the constant need to persuade a different team to generate a list or report for you. The required data can be loaded into the application you're using. For example, you can use it to provide an effective solution to the audience at the right moment, enhancing the overall experience. Using a reverse ETL tool allows data teams to focus on tackling more complicated data issues, such as maintaining high data quality, implementing security and privacy policies, and choosing the metrics and information that are most pertinent to your company's objectives and challenges.
4) Replication
Data replication is the process of storing and keeping many copies of your important data on other systems. It allows businesses to maintain high data availability and accessibility at all times, enabling them to retrieve and recover data even in the event of an unplanned disaster or data loss.
Data replication enables extensive data sharing among systems and divides the network burden among multisite systems by making data accessible on several hosts or data centers. It empowers remote analytics teams to collaborate on business intelligence projects. Data replication can be done in a number of ways, such as Full Replication, which allows users to keep a copy of the entire database across several sites, and Partial Replication, which allows users to replicate only a piece of the database to a designated destination.
Replication of data is a technically challenging operation. It offers advantages for making decisions, but the rewards could come at a cost. Certain datasets may become out of sync with one another as a result of replicating data from multiple sources at various time periods. Any roadblocks can be avoided by selecting a replication method that meets your demands.
5) Synchronization (CDC)
Data synchronization is a continuous process that updates changes automatically between two or more devices in order to preserve consistency within systems. With growing access to mobile devices and cloud-based data, the significance of data synchronization increases as well. Updates may take place in real-time by pushing data from the source to the replica, or they may occur at predetermined intervals by pulling data from the source. Replicated data should be updated so that users and applications can access the most recent information. The replicated database can be updated either live (push) or in batches (pull).
You can use the Change Data Capture tool to immediately synchronize fresh data for numerous relational databases. With the help of Change Data Capture (CDC), only the source data that has been updated is located, captured, and transferred to the target system. CDC can be used to cut down on the number of resources needed for the ETL "extract" step. Undoubtedly, the use case has a significant impact on the complexity of sync and the type of synchronization chosen. The amount of data, data changes, synchronous or asynchronous sync, the number of devices, and the choice of client-server or peer-to-peer architecture are all factors that affect it.
What is the purpose of data movement?
As your organization's application landscape and IT architecture are always evolving, your firm needs more pertinent and accurate data from many data sources. In other words, to move data seamlessly and safely between your existing systems without interfering with business activities, your data-driven business needs secure and effective data movement solutions.
The majority of modern enterprises are driven by big data, which operates around the clock. Hence, whether data is moving from inputs to a data lake, from one repository to another, from a data warehouse to a data mart, or in or through the cloud, these processes must be well-established and smooth. Without a solid plan for data migration, firms risk going over budget, creating overbearing data processes, or discovering that their data operations aren't performing up to par. Hence, the success of your company depends on having complete data transformation and data movement abilities. Your entire IT operation will benefit from the expansion and modernization of these capabilities.
There are numerous benefits to moving your data, including improved accuracy and safety. Companies should move their data for these and other reasons, as listed below:
- Data archiving: You need proactive solutions to ensure that your progress continues as your databases scale. Data movement solutions give you access to sophisticated scheduling tools so you can actively manage the scaling databases while guaranteeing the smooth operation of your business. They can also make it possible for future audits and traceability concerning compliance with regulatory standards for data capture.
- Database replication: Data movement can assist in achieving the objectives quickly and effectively if you need to make better use of distributed resources, perform faster analytics at several locations, or replicate data from a database for disaster recovery.
- Cloud data warehousing: Businesses in the data-driven world need to ensure that their data warehouses have the most current, relevant data from all areas of their organization, including legacy databases and conventional platforms. Data movement techniques can assist a company in transitioning its traditional data sources into a cloud data warehousing environment and moving data to the cloud.
- Hybrid data movement: By transferring on-premises data to the cloud, your company can take advantage of on-demand agile services to gain more useful insights and enhance decision-making. Also, it makes it simple for them to move data from cloud applications to the mainframe, giving their system access to more comprehensive data.
Why do you need a data movement tool?
Businesses are depending on data movement tools and technologies to meet all of the data consumption requirements for critical business applications as data volumes continue to climb. Your business analysts, marketing experts, salespeople, and data scientists can all use a variety of innovative methods and tools to evaluate and use data. To get the most value from your data, you must find a method to guarantee that data can transfer between systems in real-time. Data can be transferred across storage systems using data movement tools. They achieve this by collecting, preparing, extracting, and modifying data in order to make sure that its format is appropriate for its new storage place.
Businesses have a wide range of data movement tool alternatives. While building and manually coding data movement tools are expensive and time-consuming, many businesses rely on point solutions from their cloud provider, which can move the data quickly. When moving data, enterprises have 4 main options:
- Even though hand coding is the least efficient and cost-effective method of moving data, it is still employed. Teams are unable to keep up with the real-time data demands of today.
- A database licence frequently comes with built-in database replication tools, which are user-friendly. Nevertheless, they frequently don't feature transformation or visibility and are only capable of one-way data replication.
- Organizations can copy data, often exactly as it is, from one database or other data store to another using data replication software. This is helpful for backup and failover, but it is severely constrained when data is being transferred to a new system with different architectural requirements and usage patterns than the old system.
- Data integration platforms are in charge of continuously ingesting and integrating data for exploitation in analytical and operational applications. They enable the data to be streamlined and transformed for consumption in the target system.
Continue reading this guide, to discover the best alternative to manual data movement tools, that can streamline your data workflows and enhance the productivity of your team.
Best data movement tools ( Fivetran )
Developing data movement tools from scratch and manually coding them takes a lot of effort and time. This is where automated data movement tools help streamline data transmission while being more efficient and economical. One such popular tool is Fivetran which helps businesses in automating the extraction and loading of data into their data warehouses in a cloud. Fivetran significantly reduces the development and administration tasks that data engineering teams would typically have to perform in order to integrate their data sources to their numerous destinations, freeing them up to concentrate on priority tasks for the company.
As an ETL provider, Fivetran provides transformation functionality using dbt Core transformation packages as well as fundamental SQL transformations. It loads data into a variety of data warehouses, including Redshift, BigQuery, Azure, Databricks, and Snowflake, and it links to 150+ data sources that span across a wide range of diverse business use cases. In addition, their "Function connector" enables programmers to build specific data connectors for REST APIs that aren't included in their list of pre-existing connectors.
Data can be easily organized and accessed thanks to Fivetran's automated schema maintenance and speed optimization tools. As a result, small-scale activities can process data as soon as it loads. For common analytical needs like those in banking and online marketing, it offers more than 50 prebuilt data models. It is a perfect solution for companies looking to deploy source-to-target data movement efficiently, therefore keeping your data engineers focused on higher-level tasks rather than managing source-to-destination data flow.
Advantages of data movement using Fivetran
Now that you are aware of Fivetran's features, let's explore what makes it so popular in the market.
- Easily integrate data sources: With the help of Fivetran, you can handle data straight from your browser that has been consolidated from many sources. With strong pre-built connectors, you can seamlessly sync, replicate, and migrate your data from a variety of SaaS sources.
- Real-time data replication: Businesses must be able to maintain effective data movement processes, including the capability to update only the data records that have changed. Organizations can use Fivetran to replicate, process, and gather data from a variety of sources and transfer it to a variety of data destinations, including data warehouses, and databases.
- Synchronize data efficiently: To grant enterprises complete control, Fivetran provides a range of transformation choices. It enables your company to simply collect any modified data packages for more effective incremental updates and scale your data synchronization processes as needed.
- Supports event tracking: In order to load events into your destination, Fivetran interfaces with a number of services that gather events delivered from your website, mobile app, or server. The following event-tracking libraries are supported by it: Segment, Webhooks, Apache Kafka, Snowplow Analytics (open source), Amazon Kinesis Firehose, and Kinesis Firehose.
- Completely secure: Fivetran puts a high value on client confidence. They are aware of how crucial customer data security is to the principles and business models of their clients. They keep everything secure and confidential. High-security requirements are met by Fivetran through the use of data encryption both in transit and at rest including SOC 2 auditing standards, and a support staff that is available round-the-clock.
[CTA_MODULE]
Conclusion
In this comprehensive guide, you gained an overview of data movement and why you need them. You also explored the different types of data movement methods and discovered Fivetran, one of the most popular data movement tools in the market.
In conclusion, data movements' enormous power and myriad top benefits, make it an essential core capability for any given organization. In order to offer the high-performance, secure, and reliable movement of your Big Data, you can consider Fivetran, a one-stop solution for all your data movement needs. Apart from the above-mentioned features and benefits of Fivetran, you can explore more here.
Related posts
Start for free
Join the thousands of companies using Fivetran to centralize and transform their data.