Enthusiasm for AI is at an all-time high in boardrooms and C-suites around the globe.
A new study from independent market research firm Vanson Bourne and Fivetran unveils insights into the latest AI maturity levels, successes and challenges from 550 respondents within organizations with global annual revenues between $25 million to over $50 billion. Responses were collected from companies across the U.S., U.K., Ireland, France and Germany.
The study found that despite a great deal of optimism and confidence in AI technologies, poor data quality is devaluing AI initiatives.
Ninety-seven percent of surveyed respondents acknowledge their organizations are investing in generative AI in the next 1-2 years. However, the survey also found that models trained on inaccurate, incomplete and low-quality data caused misinformed business decisions that impacted an organization's global annual revenue by 6%, or $406 million on average, based on respondents from organizations with an average global annual revenue of $5.6 billion.
The key takeaway: Feeding subpar data into AI models leads to severe financial loss.
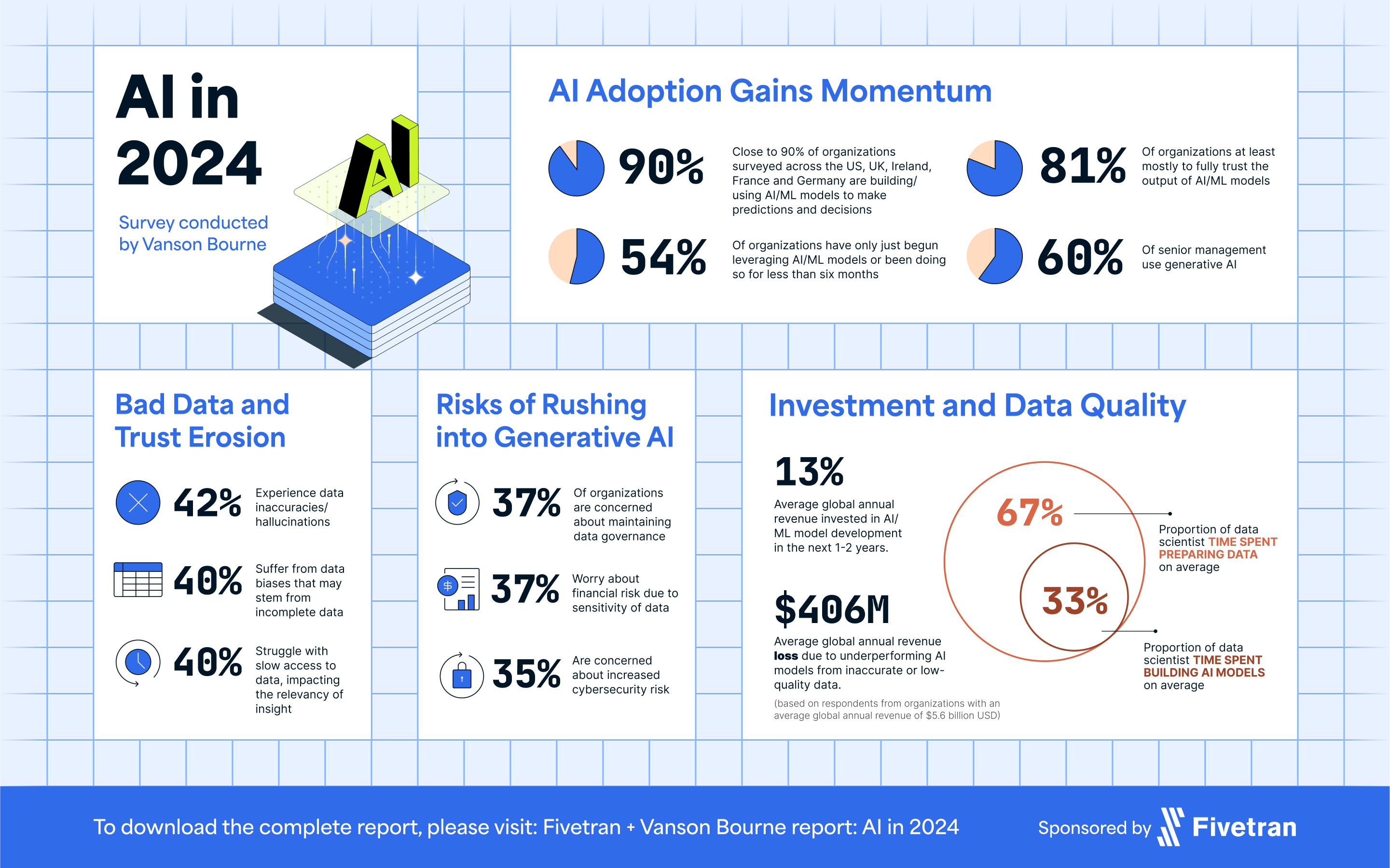
Organizational data maturity has a direct impact on AI advancement
For AI models to make decisions and predictions that can be fully trusted, the data being used to build the models must be complete, accurate and up to date.
Data must move across and in between systems — being enriched in one, pushed into another and across the business — to truly capture all the intelligence needed for data-driven decision-making that drives new ideas and a competitive edge. Companies that can do this such as HubSpot and Nauto are reaping the greatest rewards from machine learning and AI.
But this level of success is uncommon. More than three-quarters (76%) of surveyed respondents say their organization isn't fully utilizing AI and human intervention is still used” More than three-quarters of survey respondents say they struggle to use AI to its full potential, largely because siloed, low-quality and stale data are causing AI models to underperform.
While the inability to access the right information can prevent an AI model from working at all, even more alarming are the measurable losses that can occur when a functioning AI model delivers bad information.
[CTA_MODULE]
Why data integration is key to AI effectiveness
Just over half (51%) of senior decision-makers say the top barrier to building effective AI models is people within their organization who have the skills are focusing on other projects.
Organizations admit that their data scientists spend most of their time (67% on average) preparing data, rather than building and refining AI models. This leaves data teams between a rock and a hard place. They’re losing AI innovation time but they can’t risk costing their organizations money because of bad data.
Enterprise data teams have a strong case for investing in tools that strengthen data movement, governance and security. A full two-thirds of survey respondents overall, and three-quarters of respondents in the U.S., are planning to deploy data movement technologies to accelerate and fortify their AI work. Doing so will free up data scientists’ time to fully utilize their skills, likely enhancing job satisfaction and attracting more talent needed to support AI initiatives. Forty percent of surveyed companies say that hiring more people with the right skills is a top priority in the next one to two years.
Similarly, solid data governance foundations will be increasingly important for overcoming concerns about generative AI. Thirty-seven percent of respondents cited “maintaining data governance” and “financial risk due to the sensitivity of data” as top concerns.
Data integration makes a strong data foundation for AI
A strong data foundation is the result of strong data integration. When data pipelines are at their best, a business can move data wherever it needs to go and serve many purposes. This gives the entire organization the best chance at building high-quality AI models and applications.
Many enterprises are overwhelmed by the volume of data from disparate sources. Without a strong data foundation, it gets siloed and sprawled out across multiple clouds, locations, data stores and more.
Businesses need to seamlessly and securely centralize governed data from across every enterprise system to build high-quality, relevant AI models and applications. Getting to AI-driven insights requires that there is less human intervention in the collection, processing and understanding of data. The less time humans spend preparing data, the more time they can spend building new models, training tests and implementing the results of experiments.
Companies that adopt Fivetran as part of the modern data stack can quickly unlock access to near real-time, reliable data to innovate with AI. Discover how leading organizations use Fivetran to harness the full potential of AI to make the best decisions possible.
[CTA_MODULE]