Contents
What is a transactional database?
Who uses a transactional database?
Workloads in a transactional database
Transactional database vs. analytical database
Benefits of using a transactional database
Connect your transactional databases with Fivetran
Transactional databases work hard under the hood in every industry. But what makes them different from analytical databases?
Transactional databases are one of two major database paradigms, the other being analytical databases.
Where analytical databases are designed for data analysis, transactional databases are optimized for day-to-day operations such as running production systems. Though the difference might seem clear-cut, understanding the trade-offs and differences between them is key to realizing the value of transactional databases.
In this post, we’ll demystify transactional databases and explore their key differences from analytical databases, as well as some use cases and benefits.
What is a transactional database?
A transactional database is a type of database management system (DBMS) that supports transaction processing, specifically ACID transactions.
Here, a database transaction is a unit of work or logic performed by the database or DBMS. Though we often associate the word “transaction” with finance, database transactions aren’t necessarily financial or business transactions. Instead, they’re any number of operations, such as modifying an airline reservation or adding a sum to a bank balance.
By definition, transactions must satisfy ACID properties: atomic, consistent, isolated and durable.
- Atomic: The transaction must complete entirely to have an effect.
- Consistent: The transaction must conform to the database’s existing constraints.
- Isolated: The transaction cannot affect another transaction.
- Durable: The transaction results must be written to some form of persistent storage.
In short, ACID guarantees that every transaction will keep the database consistent. While inconsistencies might occur while a transaction is in progress, they’re valid as long as the database regains its original consistency after a successful transaction.
As you can probably imagine by now, a transactional database is simply one that supports this dynamic. Though that’s still a very broad definition, that’s also the point — transactional databases can satisfy a very broad range of applications and workloads.
But before we get into that, let’s explore who uses them in the first place.
Who uses a transactional database?
When we think about the difference between analytical and transactional databases, we should consider the two different types of people who use them.
Data scientists and analysts spend most of their time working with data, but in a very different way than software engineers and application developers.
Data scientists and analysts work analytically, executing queries against a database and using the data to build reports, dashboards and predictive models. They care a lot about how the data is stored and how to access it efficiently.
That’s very different from what software engineers experience. Software engineers think about app stability, app maintainability, questions about throughput and how fast the app responds to certain queries.
The difference between these two types of database users — specifically their workloads — will inform how we think about these different paradigms.
Workloads in a transactional database
Now that you know who uses transactional databases and why, you might begin to imagine what transactional workloads look like.

Transactional workloads tend to focus more on individual objects and records than aggregate data. While processing large amounts of data at once is great for data scientists, it’s not so useful for software engineers who are more concerned with manipulating objects on an individual level.
Analytical workloads
As we mentioned above, analysts and data scientists are interested in processing large amounts of information in order to calculate summary statistics.
These database operations are called aggregate functions and involve grouping together and performing computations on values from many rows. For example, an analyst might be interested in calculating the average value of all orders placed last quarter in California.
In order to produce more sophisticated insights, analytical workloads may require complex queries involving multiple steps of joining, filtering and other data processing steps. Sometimes, these analyses are performed on an ad hoc basis and never repeated.
Since analysts and data scientists only analyze data from business operations and do not build systems to perform the operations themselves, analytical workloads only require read-only queries and batch-write data loads.
Transactional workloads
By contrast, transactional workloads generally don’t involve aggregates over multiple objects. Instead, software engineers are usually most concerned with managing the state of one object at a time. Objects include any entity represented by a row in a table, such as “a user” or “an order.”
In a transactional workload, we’re managing one object — such as a user, order or patient — at a time. These database operations are often referred to as CRUD (Create, Read, Update, Delete).
A system that performs transactional workloads must be able to accommodate huge volumes of these CRUD operations (transactions) per second. Moreover, the system must also be able to ensure the integrity of every transaction so that they satisfy ACID.
Transactional database vs. analytical database
With their workloads in mind, we can summarize the differences between analytical and transactional systems in the following way.
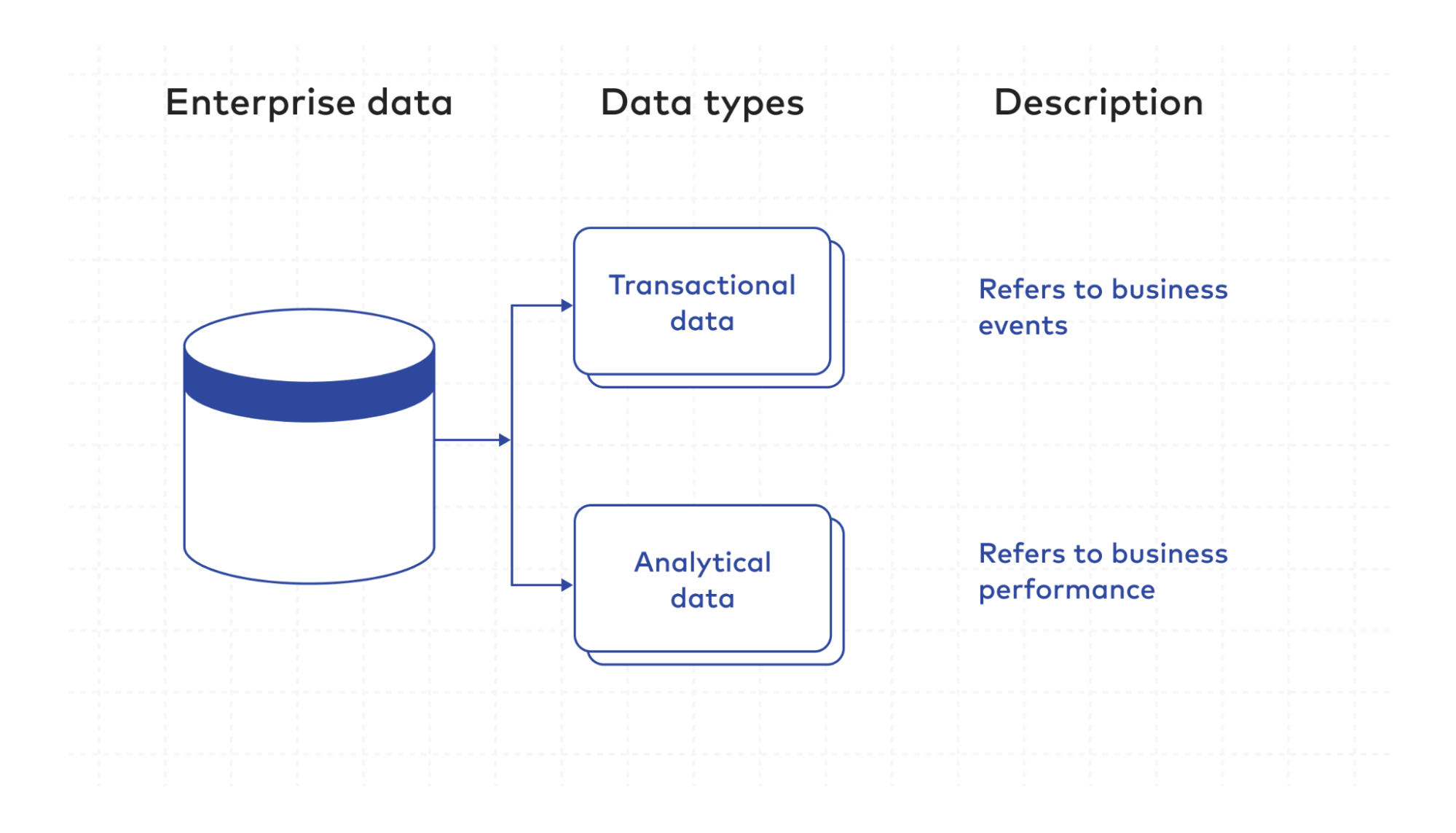
Analytical databases involve:
- Column store
- Calculating complex aggregate functions
- Read-only queries and batch-write loads
- Complex queries
- Ad-hoc, non-routine analyses
Transactional databases involve:
- Row store
- Operating on one “object” at a time
- CRUD operations
- Precisely managing the state of a database
- Supporting many operations per second with high throughput
One of the most fundamental differences between transactional and analytical databases is how they store data. Where analytical databases store data in columns, transactional databases store data in rows.
Here, row stores are better optimized for transactional (CRUD) operations since they allow for more individual manipulation of data points. By contrast, analytical databases benefit from column stores, as these make it easier for aggregate functions to work on multiple data points at a time.
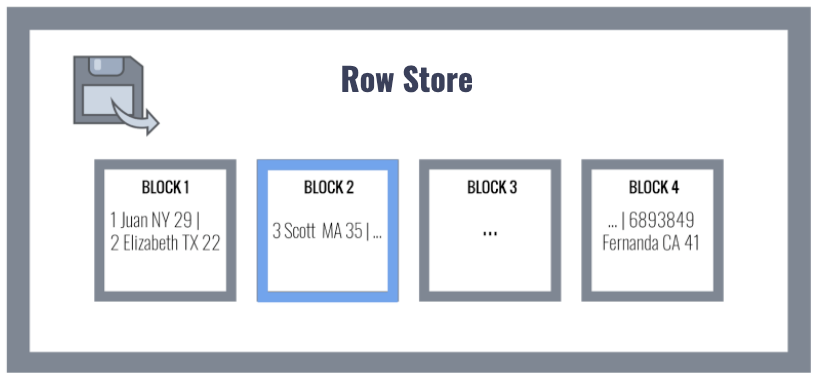
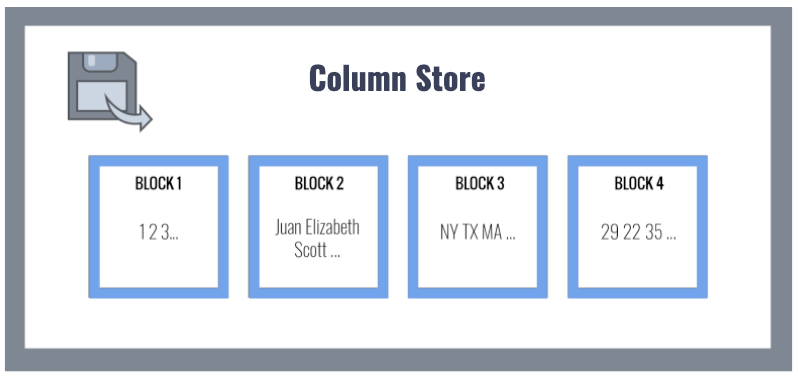
Some very common transactional databases include open-source systems such as PostgreSQL and MySQL and proprietary systems such as Microsoft SQL Database Server and Oracle Database. This is by no means an exhaustive list of databases within these paradigms, but they’re major players you’ve likely encountered before.
Benefits of using a transactional database
Though transactional and analytical databases can perform similar operations, transactional databases offer unique benefits for certain applications.

The biggest benefit of transactional databases is the ability to easily access and modify individual rows. From this capability alone, transactional databases are radically different from analytical databases.
Read and modify individual records
Since transactional databases treat individual rows as the unit of analysis, it’s much easier to read and modify individual data points. This is especially invaluable when supporting software and applications that handle data individually rather than in bulk quantities.
For example, many reservation apps use transactional databases to hold customer and booking data. Since many of the associated fields need regular, individual updates, a transactional database is an ideal solution.
Data integrity and stability
ACID guarantees that data integrity and system stability are inherently preserved throughout the entire transaction. Since data must also be written to persistent storage, data is preserved even in the event of a power failure or system outage.
Ultimately, all of this means reduced transaction failures and, as a result, improved database performance for any connected transactional applications or software. By contrast, analytical databases working in bulk quantities are rarely concerned with this and rarely provide the capability.
Connect your transactional databases with Fivetran
With Fivetran, it’s never been easier to connect transactional databases to your data pipelines and warehouses.
Your production database contains your most valuable transactional data. That’s why our database connectors are our most tested and battled-hardened. Designed with security and correctness in mind, you can rest assured that your database replications are accurate and problem-free — no matter what.
For more information and to start your free trial, sign up today or contact one of our database experts.