Back in 2012, “data scientist” was labeled the “sexiest job of the 21st century.” The tech world was abuzz with the revolutionary potential of machine learning, artificial intelligence and big data. More than a decade later, this potential remains largely unrealized. This is to a large degree because organizations have not yet built the foundational tools, processes and practices that support machine learning and artificial intelligence projects.
Data teams run a real risk of rushing headlong into prototyping powerful, intricate systems before they have the capabilities to fully leverage or sustain those efforts. This especially poses a danger to tempt technically-minded data scientists and engineers to pursue technologically advanced and intellectually interesting projects — while losing sight of practical problems that directly concern the needs of the organization. Specifically, there are two major pitfalls involved in the premature pursuit of machine learning:
- A lack of fit between problem and solution – it’s tempting to pursue a solution that’s overkill for the problem it’s meant to solve. Many analytics problems can be solved with simpler methods, such as heuristics, rather than machine learning or predictive modeling of any kind.
- Lack of supporting infrastructure – many organizations hire data scientists before establishing robust data operations, forcing data scientists to assume duties more commonly associated with data engineers and analysts (namely building data pipelines, dashboards and reports).
To avoid these two major pitfalls an organization must meet two major requirements to support machine learning and artificial intelligence:
- There’s a specific problem machine learning can solve that’s not adequately addressed through simpler analytics methods, such as heuristics.
- The organization’s data infrastructure is mature enough to support machine learning. Machine learning depends on large volumes of high-quality data, meaning that an organization must have a way to extract and load data from sources into a destination that can cost effectively store it.
Frameworks for judging readiness
You should think of machine learning and artificial intelligence as the pinnacle of data science. There are two models you can use to chart a progression to machine learning, corresponding to how an organization uses data and the tools and processes it uses to manage data. These two models also correspond with the fundamental questions of whether an organization
- Has a problem that machine learning can solve
- Has the technological and organizational capabilities to support such a solution
The data maturity curve is an excellent model for describing how your organization uses data and its overall orientation toward data.
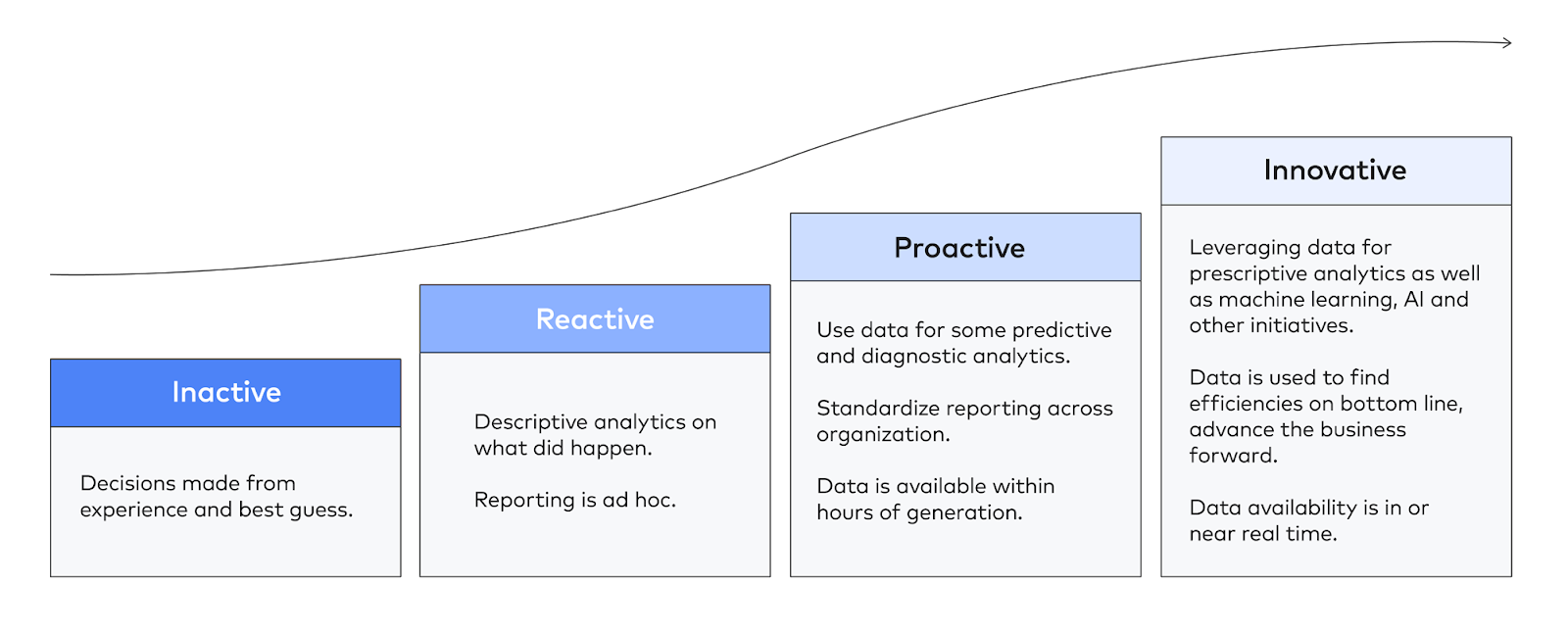
At the bottom of the curve, an organization that is inactive uses data sparingly or not at all, making decisions on hunches and best guesses. This is appropriate for early-stage organizations that are trying to achieve product-market fit.
Organizations that are able to maintain some ad-hoc reporting supported by manual, inefficient data integration are best considered reactive.
An organization that uses data proactively, by contrast, has systematized the production of data assets (i.e. data models, dashboards, reports, etc.), supported by ensuring the timely ingestion of data. Such an organization is well on its way to promoting widespread data literacy.
In order for an organization to pursue innovative uses of data such as machine learning and artificial intelligence, it must have fully realized proactive uses of data.
The other model, the data hierarchy of needs, describes an organization’s tools, processes and capabilities with respect to data.
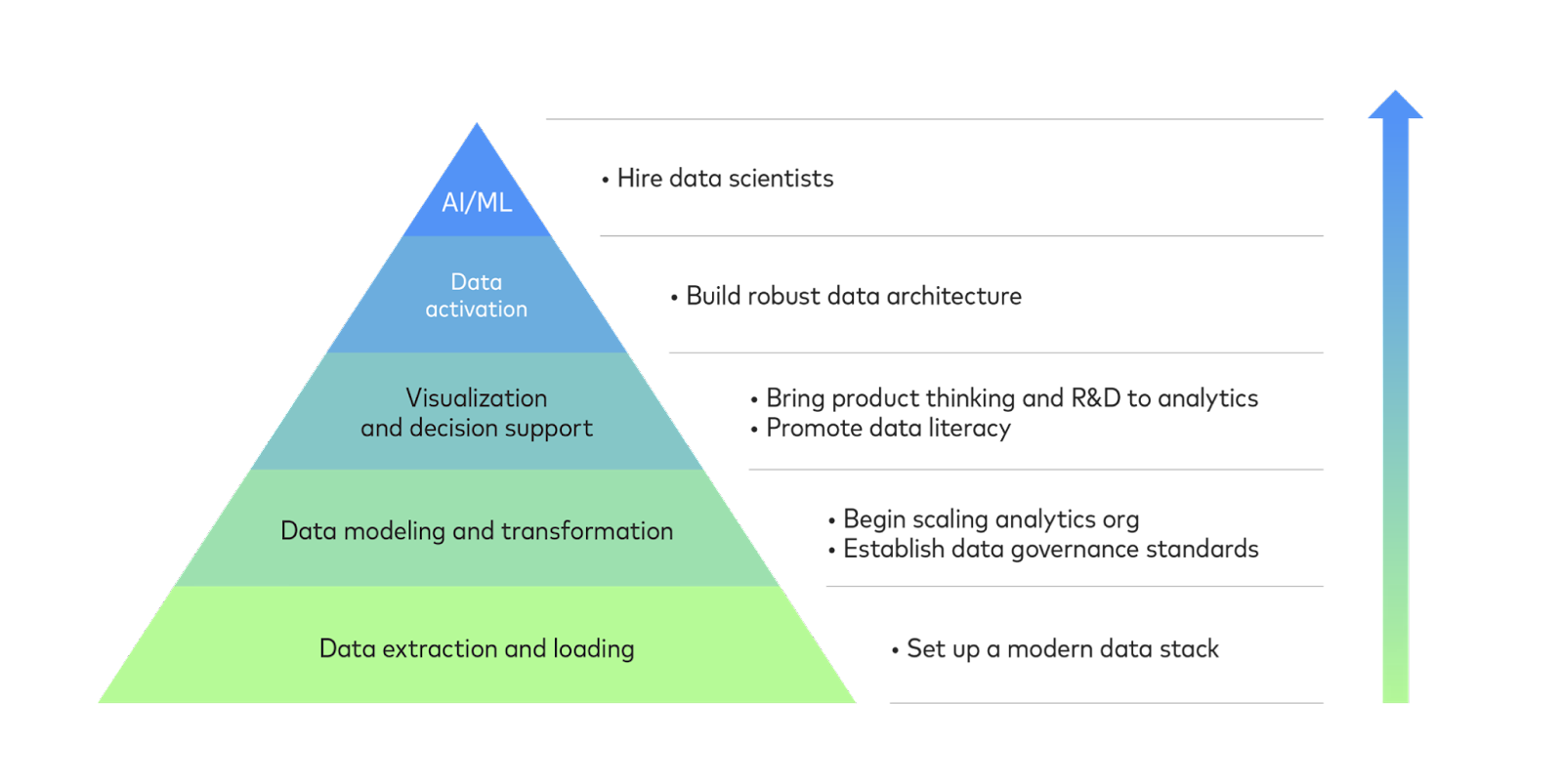
At the bottom of the data hierarchy of needs, the most basic capability an organization must have is the ability to move data from a source to a location. This requires a suite of tools called the modern data stack. Once an organization can reliably extract and load data, it is well situated to model and transform it into usable assets for analytics. This enables an organization to hire additional analysts, scaling their data team. It also necessitates the creation of data governance standards as data assets and their usage both proliferate.
Widespread use of data visualization and decision support, i.e. data democratization, is the obvious next step, accompanied by product thinking and the promotion of data literacy. In turn, this will prepare an organization to “activate” data by moving data models back into production and operational systems in order to supply employees and customers with real-time analytics or to automate important business processes.
The ideal time to pursue machine learning is when you’ve picked all of the low(er)-hanging fruit. These foundational steps not only lay the groundwork for sustaining machine learning long-term but are also, in their own right, lower effort, higher reward activities that serve as proofs of concept, generating credibility and buy-in for more complex and riskier data projects like machine learning.
Getting your organization ready
Per the two frameworks we have discussed, the key to getting ready rests on leveraging the power of technological solutions to build a robust data infrastructure —in turn enabling your organization to develop a more proactive and innovative orientation towards data.
On the technological side, you will need data infrastructure in the form of the following suite of tools:
- A destination that can accommodate large volumes of data, such as a data lake or similar cloud data platform
- A data movement tool to move data from your sources to destination that ideally requires minimal human intervention and engineering time
- A tool to transform data into usable models while using collaboration, version control and other engineering best practices
- A business intelligence platform to produce dashboards and help support the decisions of people across your organization
- A data activation tool to help operationalize and productionize data models by routing them back into operational and production systems
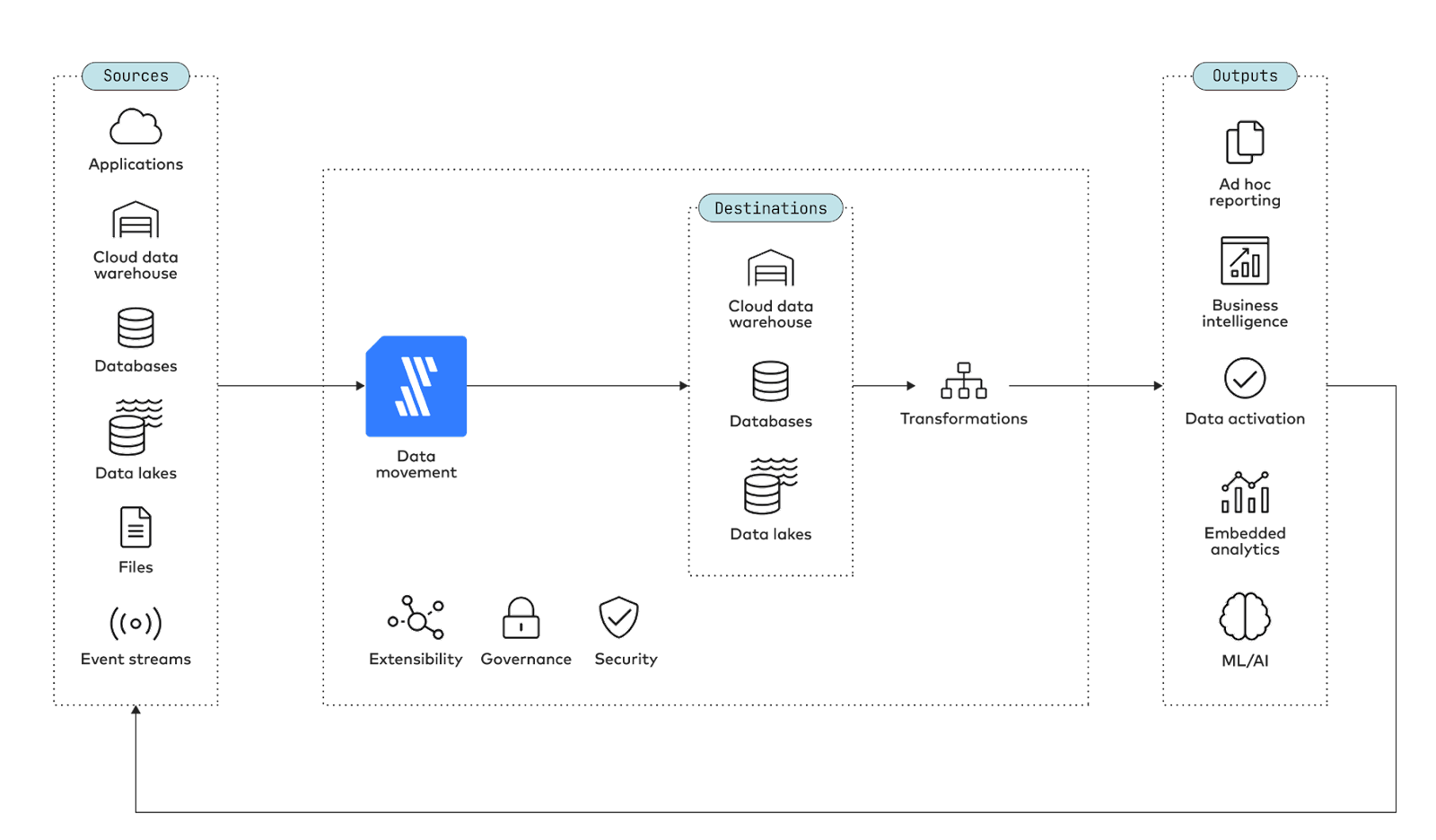
On the organizational side of things, you will need:
- A robust analytics organization including analysts who specialize in understanding the needs of specific departments
- A mature, repeatable process for producing data assets
- Widespread data literacy and the routine use of data to support decisions
- Data engineers who have the bandwidth (and expertise) to support machine learning by bringing models into production
Once these criteria are met, you’re (finally) ready to hire data scientists in earnest and realize the potential of machine learning, artificial intelligence and big data.
To experience for yourself how easy it can be to start building a strong foundation for data science, schedule a demo or consider a trial.
Good luck!