Building a modern data infrastructure is one of the most important first steps in the development of an organization. For early-stage startups, it’s a step toward maturity that enables them to make data-driven decisions.
We spoke to Kat Gardiner, a Marketing, Growth and Analytics Consultant who frequently works with Series A startups to get a glimpse of what her clients like Ava have encountered in their quest toward creating a modern data stack and a mature data-driven practice.
Can you tell us a little bit about yourself and the type of organizations you work with?
Sure! I’m Kat Gardiner and I work as a Marketing, Growth, and Analytics Consultant. While I wear many hats, I work with many growth-stage companies looking to build out their data infrastructure.
I enjoy my work with Series A companies specifically because I gravitate towards helping tackle the complex challenges those organizations are facing at that point in their growth. They finally have the resources to hire the right people and start addressing some of the operational tech debt they’re encountering.
Getting started
What advice would you give to startups that are just commencing their data journey?
There are two issues I typically see amongst my clients as they get started:
The first is a lack of impulse control. Many of the companies I work with have binged data across a variety of sources. Unfortunately, with the lack of structure in place — they’ve struggled to leverage that data efficiently. While there’s always an impulse to collect as much data as possible, it’s important to understand why you’re doing so and what types of data provide the most value.
The root of all data practices start with the intent to answer questions about your business model. You need to have a clear understanding of what questions you’re trying to answer to select the right data sources and types. Identifying goals within the context of your business model will ensure you’re focusing on gathering key data points that contribute towards your objectives.
The second is an anxiety of choice. Many companies have dozens of data sources now. If you lack the people and processes to manage and QA that data — you’ll have a hard time interpreting it and trusting its accuracy. Many of the companies I work with have difficulty discerning what data is actionable, accurate and up-to-date — which can cause a lack of strategic focus during analysis.
It’s like having a full fridge, but a lack of recipes to turn it into a dish that can fuel your decisions.
So for organizations at this stage, where should they start?
It all starts with proper planning. You need to understand how your business is operating and the ideal state you’re looking to achieve. What are the goals for your business right now and in the next 1-2 years, and how can data support your decision-making on that journey?
- Reducing costs?
- Increasing customer conversion?
- Improving customer lifetime value?
- Making the business more efficient?
- Finding product market fit?
- Understanding your most valuable features, products, or markets to enter?
Think of these goals as ascending a mountain. (I love hiking, so bear with me!).
When you’re hiking, your ultimate goal is the summit. In this context, the summit is your long-term growth goal — the north star that’s discussed in each All-Hands meeting.
In order to reach that summit, you’ll have to climb switchback after switchback. In the context of your long-term growth goals — each switchback is a quarterly OKR or global hypothesis, which when validated or achieved, helps your team continue your journey.
This approach helps break down a large (perhaps intimidating goal) into bite-sized chunks. It’s also flexible enough to allow for meaningful learning along the way, while still maintaining an eye on the ultimate north star metric.
While you’re not climbing all the way to the summit today, you need the right plan and equipment to start scaling those switchbacks — while still considering the height and length of the journey ahead.
In the final parallel of this metaphor, that means building a system and strategy that fits your budget and needs today — but is still scalable for the future.
Knowing this, you should understand what your current data sources are, but also consider what sources you’ll likely need in the future.
The next critical component is making centralization part of that strategy.
Maturing your data process requires breaking down siloed data sources. You can’t get a holistic view of your business, in real-time, if you’re working out of spreadsheets and going to each individual source to run an analysis. It’s inefficient and could lead to lost insights buried behind time cost or complexity.
Having one centralized source of information not only makes it easier for a data analyst to leverage your stream of data, but it also democratizes access to that data now — and especially in the future as your business grows.
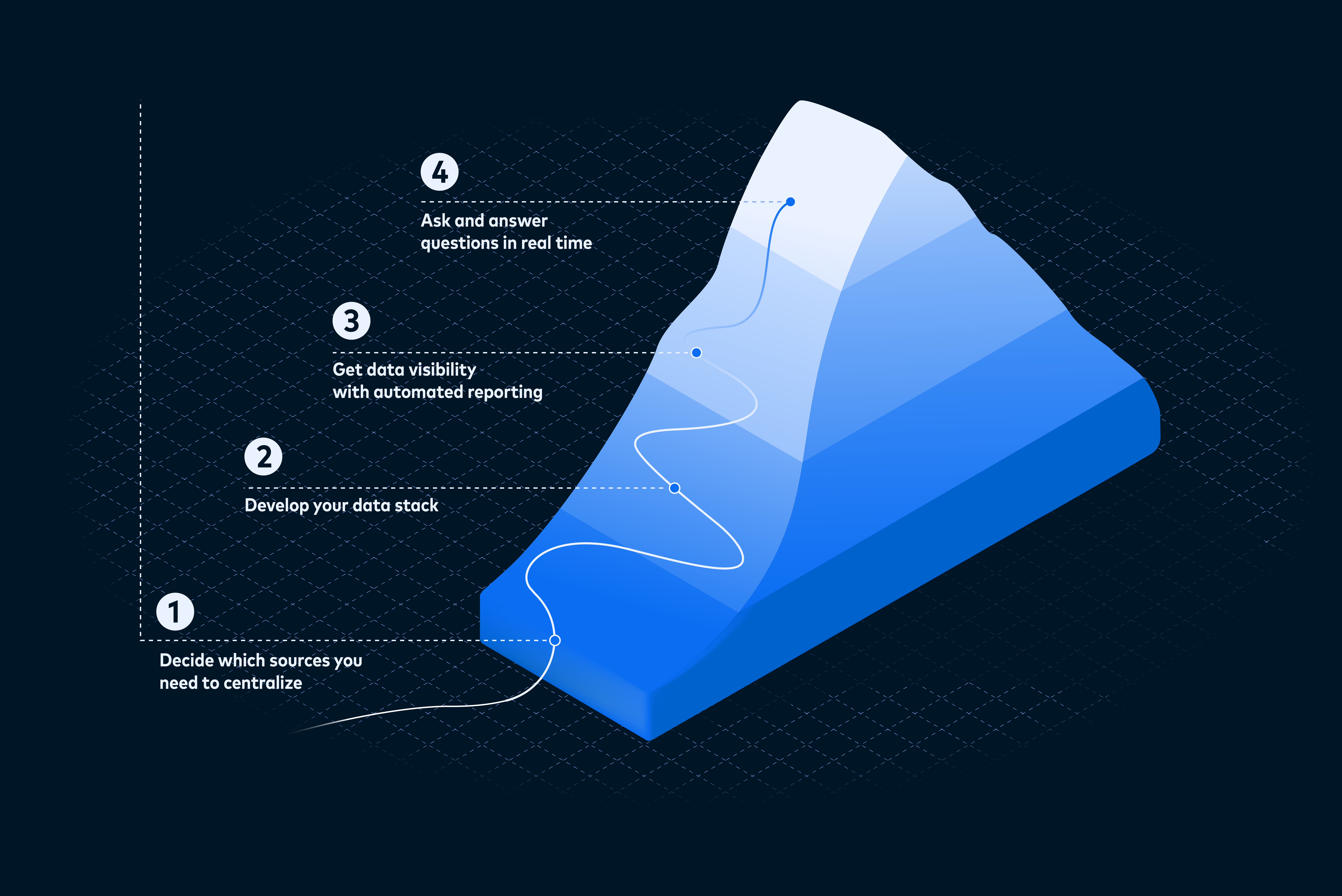
When helping a company develop their strategy, are there any foundational data concepts they need to consider?
For sure! Some of my early work with companies involves developing the base level of data processes and considerations. Tasks like creating a data dictionary with proper taxonomy and establishing a relational dashboard tree with level-specific metrics pays dividends later.
Those considerations help align the data organization with the wider organization and get everyone to speak the same lingo.
What are the top questions that early-stage start-ups should ask themselves and look to answer with reliable data?
Many companies start by looking into financial data because it is accessible and drives core decision-making. But this shows the outcome, not the why. Some questions to help you get started:
1) Who are my ideal customers? What segments exist, and what needs do my product(s) fulfill for each one?
This one is all about searching for and understanding power users as a means of uncovering the why behind potential product-market fit. Using data to demystify this question helps companies hone in on their buyer personas, top segments in terms of annual recurring revenue (ARR), and with whom their product is a slam dunk.
2) What steps are required for purchase/activation? When do they fail to connect to value, and why?
While this is difficult to answer without an efficient and centralized data process, it’s necessary to reduce friction and increase motivation in the customer journey. It helps companies establish what their ideal marketing mix looks like, including understanding what the right type of content looks like and what the ideal touchpoints are.
On the product side, it helps companies understand what’s necessary to get a customer interested, what the customer needs to see to reach their aha moment, what gets in the way, and what level of onboarding is necessary to obtain and retain customers.
3) What are the elements of ongoing value that drive repeat purchase/retention?
Similar to the last one, but critically important to drive repeat purchases and reduce churn. (It’s always cheaper to get repeat or renewed purchases). Digging into data from sources such as a loyalty program or understanding what key network effects drive continued engagement requires connecting the dots across your data landscape.
4) What channels/growth loops drive the most customer acquisition and return on ad spend?
This is nearly impossible to answer without centralized data. Understanding how customers are interacting with your marketing and acquisition efforts across the full scope of your efforts — from brand awareness, inbound, outbound and once they’ve converted will give you a full picture of what contributes towards acquisition. Then, you can make decisions on where to invest more - and where to invest less.
These questions will set the stage for your growth strategy, and help you determine what data you need. Often, this requires some combination of financial, product, customer and marketing relational data centralized and analyzed together.
Getting more done with fewer resources
You work with startups, who often have tight budgets. What are some ways to manage the initial implementation with limited resources?
Automation is key.
If your business is complex, you’re likely looking to gain insights from a multitude of sources. Most of the organizations I work with lack the headcount to devote resources to build and upkeep DIY solutions to gather that data.
But that approach is unnecessary — especially when there are inexpensive or free, automated tools available that accomplish the same job. By utilizing automation to connect disparate data sources into one centralized destination, you’ll gain major time and resource savings now — and in the future.
Of course, there are the go-to tools, such as Google Suite and Google Analytics. But the fact that solutions like Fivetran have free plans provides great value for these small teams with tight budgets.
Seems like an easy transition to asking how you’ve used Fivetran to help your clients, can you tell us a little about that?
Yes! So one example I’ll use was with a company called Ava. They’re a live captioning application that uses AI to transcribe what people say, empowering Deaf and hard-of-hearing people in the digital workplace.
They weren’t big enough to justify something truly complex, but they needed all of their marketing data in one place. Their volume wasn’t going to break the bank and investing in a complicated solution with a large learning curve wasn’t feasible. It was important to find a solution that wasn’t going to cost a lot of money and didn’t require maintenance.
Many of my friends in the data space recommended Fivetran. Your Free Plan was perfect for Ava’s needs and size. They could easily connect all of their marketing sources and send that data to BigQuery - all without impacting their budget. I also liked that you can choose which tables you want to use versus other companies which want you to push way more data.
Ultimately, they didn’t have to worry about upkeep or maintenance, and the visualizations and automated dashboards it enabled made it easy for them to understand the full landscape of their data without having to log in to a bunch of different places.
That touches on what I was speaking about earlier, both regarding understanding what’s financially feasible now — while not ignoring scalability in the future. When an industry-standard solution like Fivetran has a free version that doesn’t impact their budget, it’s sensible to leverage it now — while knowing that they can easily upgrade to a paid version in the future if their data volume substantially increases.
Leveraging Fivetran’s Free Plan
What positive business outcomes have your clients experienced from utilizing Fivetran?
The first is the improvement in resource allocation. Automating data movement helps free up time for the limited data engineering and analyst headcounts these organizations have.
I’ve worked with clients before whose analysts spent around 85 percent of their time aggregating data, leaving the most important part of their role — presenting insights — limited to around 15 percent of their bandwidth.
In that same vein, automation also improves the overall morale and job satisfaction of the analysts at these companies. Suddenly, they could focus on more diverse, high-impact projects that were more gratifying and improved their careers. It helped transform their role from execution to strategy.
Lastly, the data process across the company is democratized. When data is less difficult to utilize and easier to access, employees feel confident leaning on and leveraging data individually to make critical decisions.
In the case of Ava, reliable reporting unlocked marketing channel-specific optimization for their growth team. Before they were largely running on intuition and best practices. Now, leadership could make data-driven hypotheses, enabling more quantitative growth marketing.
Buy-in across the company vastly improves once you start seeing the results. It’s easy to assign value to data and your data team when they’re able to support decisions that encourage growth.
[CTA_MODULE]
Thank you for speaking with us! Where can readers find you and what’s a fun fact they can learn about you?
Feel free to connect with me on LinkedIn or reach out to me through Levers Labs if you’re looking for someone to help guide you through the process of developing and optimizing a modern data stack.
In terms of a fun fact, I backpacked in Southern Asia and Patagonia for 7 months!
Thanks for speaking with me!
[CTA_MODULE]