Why everything doesn’t need to be generative AI
Parag Shah, Senior Director of Data and Analytics at Rocket Software, joins us to discuss the possibilities of taking a hybrid approach to AI, as well as the importance of choosing modern, scalable data tools.
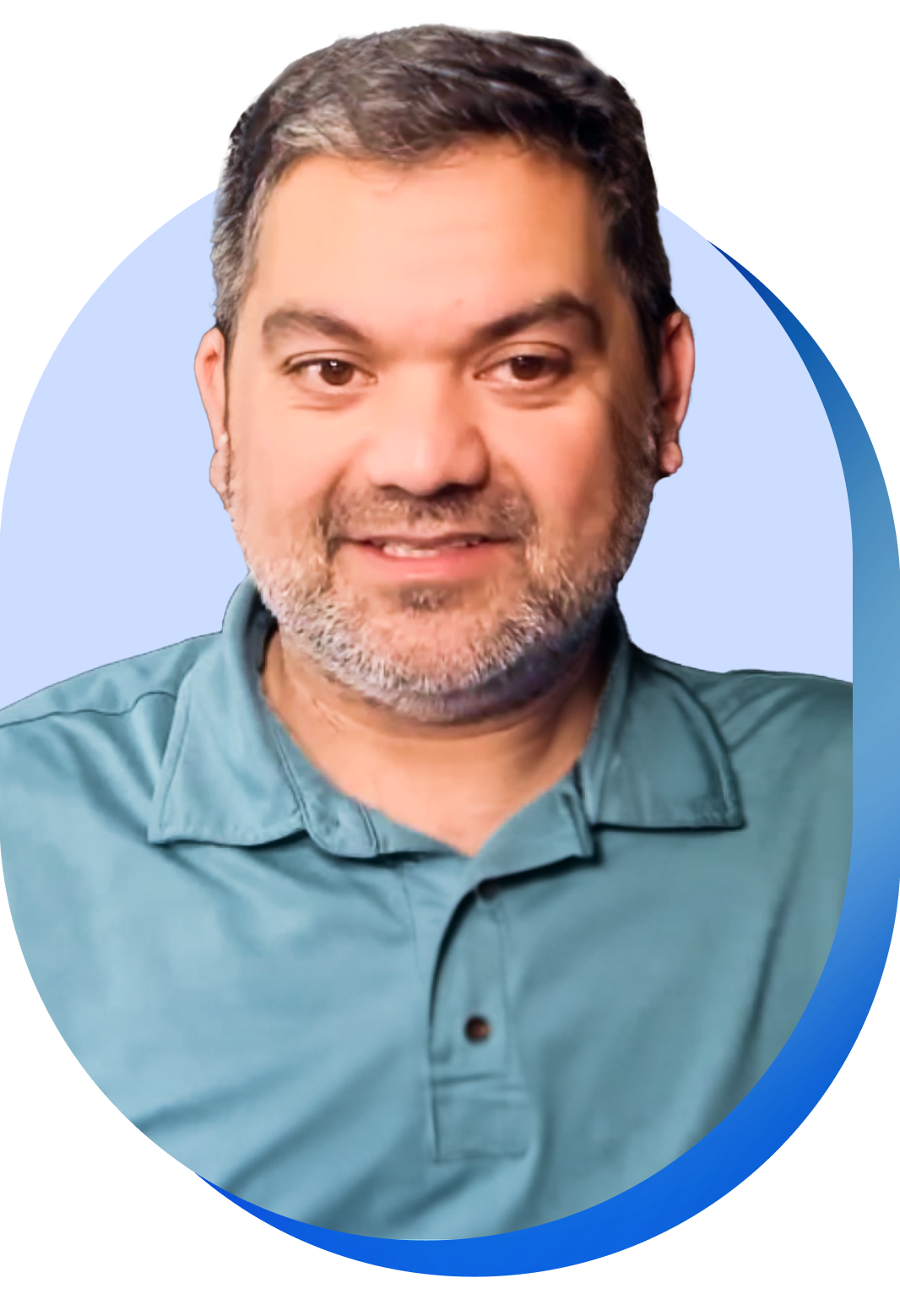
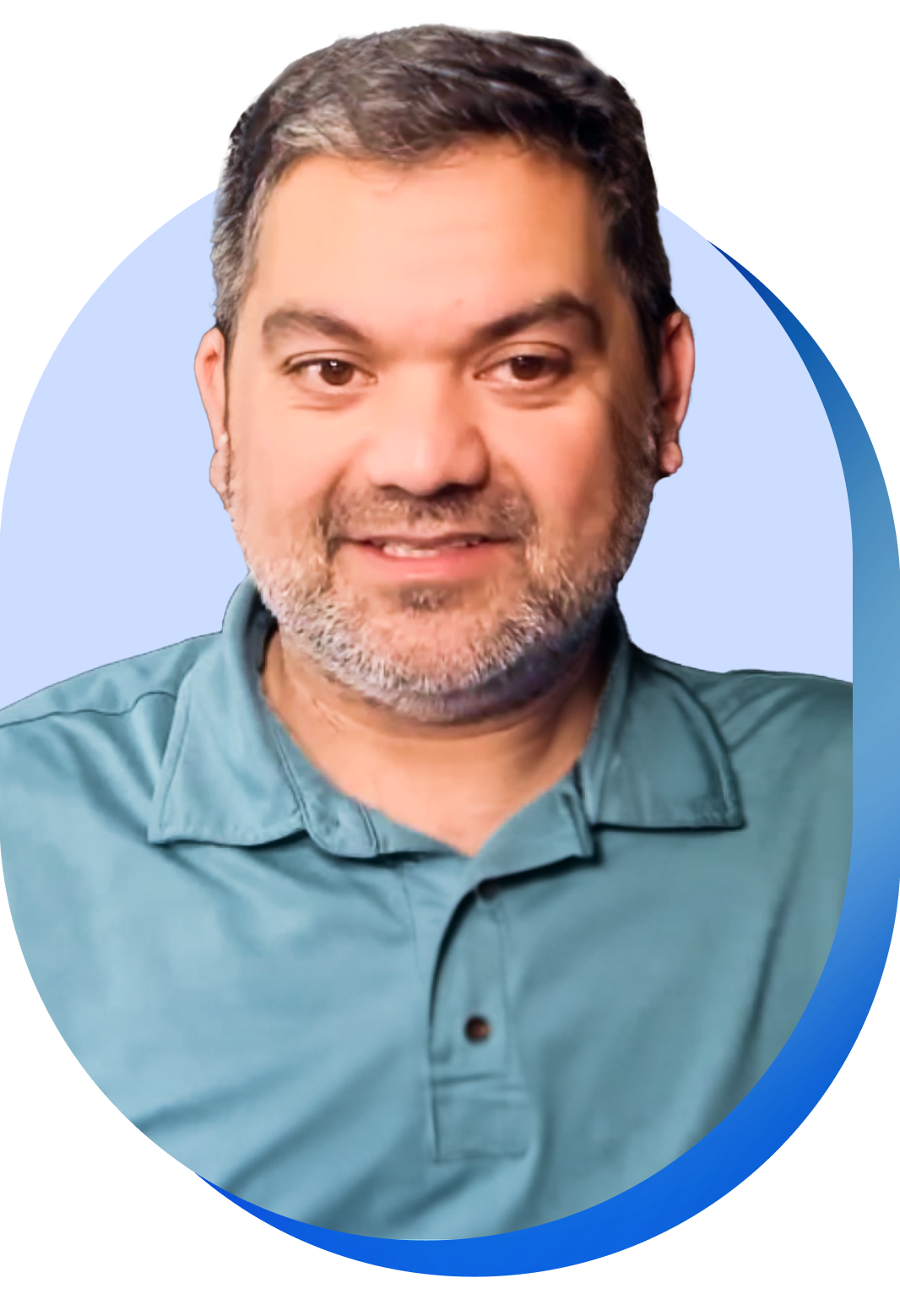
More about the episode
Businesses have been fixated on generative AI, but is the buzz detracting from the possibilities around predictive AI? And where do the technologies fit into your data stack?
We talked with Parag Shah, Senior Director of Data and Analytics at Rocket Software, about the company’s three-year roadmap for predictive AI and how its scalable data stack dramatically reduces the time to acquire data.
“We did our largest acquisition at Rocket in 2021 and acquired all the data in two weeks. That was a direct result of the modern data stack that we implemented.”
Here are key highlights from the conversation:
- AI use cases in the enterprise, such as using predictive analytics to improve operational efficiency
- Tips for building a unified team of data experts
- How to scale your data stack while meeting complex data integration needs
Watch the episode
Transcript
Kelly Kohlleffel (0:00)
Hi folks, welcome to the Fivetran Data Podcast. I'm Kelly Kohlleffel, your host. Every other week, we're gonna bring you insightful interviews with some of the brightest minds across the data community. We'll cover hot topics such as AI, ML, of course, GenAI, enterprise data and analytics, various data workloads, use cases, data culture and a lot more.
Today, I am really pleased to be joined by Parag Shah. He is the Senior Director of Data and Analytics at Rocket Software. He currently leads the company's data engineering, data analytics, master data management and data science teams. Parag has more than 15 years of experience building data teams across industries, specializing in modern data and BI stacks. Prior to Rocket, he spent time with both Staples and Bank of America in a number of capacities across engineering compliance and BI. Parag, welcome to the show.
Parag Shah (0:52)
Hey Kelly, thanks for having me. I'm excited to be here.
Kelly Kohlleffel (0:56)
It’s great to have you. I would love to dive into Rocket Software's data journey and sift through the hype around AI/ML/GenAI we're hearing about all the time now. Before we get into that, could you share a little bit about Rocket Software and your current role?
Parag Shah (1:16)
Sure. I've been at Rocket for four years now. We make modern software for legacy applications and legacy mainframes. We help companies scale and move towards that hybrid cloud architecture that more and more companies are moving towards.
Kelly Kohlleffel (1:35)
As much as we talk about modern, there are a lot of mainframes still out in the market today.
Parag Shah (1:41)
I mean, if you look at the Fortune 50 companies, I can guarantee you that at least 90% of them are running on mainframes.
Kelly Kohlleffel (1:48)
You've got a varied background. What originally caught your interest with Rocket Software?
Parag Shah (1:56)
When they reached out to me at Rocket Software, it was actually a really interesting conversation. What they essentially told me over the phone is: “Listen, Parag, if you can come in here and we can talk to you — we have this unique opportunity for you to have a blank slate and to start from scratch.” For someone like me, who is essentially a born change agent, it was music to my ears. As soon as they said that, that hooked me.
Kelly Kohlleffel (2:26)
That’s really unusual, especially for a company that’s been around for a while, to say “Hey, here is a blank slate, let’s get this done right.” Rocket has gone through a lot of acquisitions over the years. How does it influence how you think about your data program, delivering data products and delivering data services? How do you select your technology stack and some of the processes and approaches?
Parag Shah (3:00)
It played a large role. When you think about acquisitions, you're acquiring a lot of data. You're acquiring data from CRMs, ERPs, from all these different systems that exist across all these organizations, big and small. When you're doing an acquisition integration, first you acquire all the data, analyze the data and then you figure out how that data is gonna fit into our CRM and ERP.
You need to build for scale and cost efficiency. The way we look at cost efficiency is, for instance, having distributed storage and compute, so you know where your dollars are being spent. That's how it influenced our decision. We're going to have a lot of different data sources, so we need a lot of flexibility and we need scalability.
Kelly Kohlleffel (3:53)
When most companies think about acquisitions, you think about the data integration challenge that it is, with two different CRMs, two different ERPs and two different operational systems. Usually, there's at least a one-year, sometimes three-year window to get all of this data in and say “Hey, we’re finally a combined entity.” Is that how Rocket thinks about it?
Parag Shah (4:19)
No matter where you go, whatever organization you work for, when they do an acquisition, you're going to try to compress the timeline to achieve any sort of synergies that you may have. So understanding that timeline and understanding the staffing for that timeline is a big deal that Rocket is getting better and better at. They're learning from every acquisition and that's the key.
Kelly Kohlleffel (4:45)
Do you feel like a modern approach to data gives you an advantage when you're incorporating and combining data sets from acquisition into the overall Rocket portfolio?
Parag Shah (4:55)
Absolutely. Prior to us having implemented a modern data stack at Rocket, it would take, for a small acquisition, anywhere from six to seven months to acquire the data. We did our largest acquisition at Rocket in 2021. We acquired all the data in two weeks.
Kelly Kohlleffel (5:19)
Oh my gosh, that's incredible.
Parag Shah (5:20)
That was a direct result of the modern data stack that we implemented.
Kelly Kohlleffel (5:27)
Wow, months to sometimes years down to weeks. Really incredible. You talk about modern data stack, and it’s always this delicate balance: should I build or should I buy? Which way do I go for what tool? How do you look at it as a data leader and balance out that build versus buy decision?
Parag Shah (5:55)
That’s a good question, it's a tough question. When you're looking at build vs. buy, it depends on your staffing and your budget. A lot of what it comes down to is what you have for either operational budget versus capitalizable budget. With the newer accounting rules, we're seeing that we can capitalize on new capabilities projects, even if they're in the cloud.
Previously, if we could build it on-prem, let's build it on-prem because we can capitalize the whole thing. But now we're able to buy and gain those efficiencies and get semi-managed services that get our lean teams to focus on more difficult and more rewarding tasks for the organization — and for them. So the approach that we're looking to take when we're looking at build versus buy is: What's our efficiency model? What does our staffing look like? What does our budget look like? Based on that, we can make an informed decision.
Kelly Kohlleffel (6:58)
Yeah, and I think as part of that, and you alluded to this, is asking “Can we get differentiation? Is there a level of differentiation that we can get with a build? Or is there something we can buy today that eliminates all the work associated with that?” If I can take advantage of what I have today and if there is a measure of business differentiation that I need that I can't get from a “buy” decision, then that can play into it as well.
Parag Shah (7:29)
Yeah, let me give you a good example here because we did a build and we did a buy. When we were looking at master data management, we could have bought a tool off the shelf. We could have gone with one of the leaders in the MDM space. What we did was we decided to go with an open-source tool and build in-house based on their framework. The reason we did that is because we wanted to have a level of flexibility to add multiple product hierarchies, to add different fields, to sort things in different ways and to be able to control the metadata. Something highly customizable, so we built it. There was nothing that was out there that had the level of customization we were looking for.
Our buy was Fivetran. We decided we were going to buy a tool as opposed to trying to build it in-house. “Do we want to build an enterprise service bus? Let's use Kafka, let's use Hadoop, and let's go all in on it.” Well, that would have cost way more than we spend on Fivetran, so we have efficiency issues, and then it would have taken significantly more time to get spun up. Fivetran, or someone like Fivetran, has done the work to create connectors to data sources that we all use across the industry. Let's take advantage of the work that they did.
Kelly Kohlleffel (9:11)
You’ve gotten so much done, and I know you can never sit still here. Where do you feel like you want to be this time next year, in the next 12 months?
Parag Shah (9:25)
My primary focus is going to be on predictive AI. Let me take you back to day one at Rocket Software. I sat down with the CIO at the time and with the VP of Business Applications and said, “Here's my three-year plan. I'm going to have us build the foundation so that within three years, we have the data pipeline and the data architecture built for us to pursue advanced analytics, predictive analytics.” That was the goal. But now for the next 12 months, we had this little thing that popped up around April of this year that had a lot of buzz: ChatGPT.
Kelly Kohlleffel (10:07)
It's hard to find somebody that hasn't tried it out at this point, right?
Parag Shah (10:11)
Absolutely. It's a great tool, but it created so much buzz. And that buzz was both good and bad. The buzz was great because it got people thinking of AI. The bad is that they were thinking only of generative AI. They were only thinking of ChatGPT, Bard. , asking bots questions and how can you utilize that? Completely skipping over the operational efficiencies that come with predictive AI. That's where my team wants to focus in the next 12 months, in that predictive AI space.
Kelly Kohlleffel (10:51)
Can you do a quick comparison on building a predictive analytics app or building a GenAI app? How do I think about those differently?
Parag Shah (11:05)
When you think about predictive AI, you're looking at past data to predict future outcomes. Based on historical data on a customer, based on all of the conversations that they've had with support, on their buying patterns and how long they've been a customer. Can we predict with a reasonable degree of accuracy and lead time, whether or not they're gonna churn and leave us within the next year?
That's a predictive AI and operational efficiency use case because studies have shown that it costs about 30% more to acquire a new customer than to retain one that already exists. That's what I'm talking about when I say operational efficiencies.
When you look at generative AI, you're looking at creating content. There's a use case where you might have a predictive cross-sell/upsell model that tells the sales rep, “This customer you're talking to about product A, based on this customer’s prospect size, their industry, employees, revenue, all of these different factors, might benefit from a cross-sell product C and D as well.”
Generative AI could come in to synthesize and create a script for that sales rep to read to help them cross-sell these other products to the potential customer. That's where I see Generative AI having a huge use case when it comes to the sales cycle.
I also see use cases for generative AI in documenting code. Feed it all of my code that my team hasn’t had time to create documentation for, let it synthesize that code and spit out some English documentation. That would complete 80% of the most tedious tasks in development.
Kelly Kohlleffel (13:03)
If you can get 80% on something like that, that is a huge gain. In the next 12 months, are you going down a predictive analytics path right now? Are you going down to GenAI? Is it some sort of combo of that? And secondly, is there some pressure coming from either upstairs or from the grassroots saying, “Hey, let's go do some of these things?”
Parag Shah (13:28)
Yeah, there is. There's a lot of pressure coming down saying, “How do we utilize large language models? How do we utilize generative AI?”
From an operational standpoint, we're going to look more at predictive AI. How can we help our sales reps cross-sell and upsell? How can we predict customer churn? But we're a software company, we have software products. So the question is going to be, “How do we build generative AI and chatbots into our products to make them more user-friendly? How do we enable them to generate content when they need to generate content?
We're going to be taking a hybrid approach. Where in our product suite, we're going to look at a little bit of both predictive AI and generative AI, but internally, we're going to focus a lot on the predictive AI piece.
Kelly Kohlleffel (14:18)
Love it. I think another dimension too, potentially, from a product engineering standpoint is: “Can I use GenAI to maybe build my new products better, faster, easier and more efficiently?” So there are so many aspects to this. I love the example that you gave of not diving in too deep and taking a step back.
Anytime you do that, regardless of what that data workload is, or the application, you want to have a solid business problem. You talked about a couple you were going to go after. Is there anything else you see over the next year that stands out to you from a kind of a business use case perspective?
Parag Shah (15:03)
I generally move on a “prove it” kind of pattern. Where I'm going to go in, I'm going to prove the usefulness of what we can do. In some cases, I need staff to do that and “prove it”. But once I “prove it”, my staff grows. So that “prove it” mentality is something that's helped me be very successful in everything that I've done in the data space.
I have modern data stack ideas, we go out and prove that we can reduce the time to acquire data by 92%. That's a huge reduction. So we get that “prove it” mentality. I want to prove that we can build this customer churn model, that we can build this cross-sell upsell model, and that we can impact both revenue and retention.
If we can do that, I think what you'll see by 2025, is we'll want to invest in maybe a propensity-to-buy model. We'll have some level of personalization for our customers that we want to implement using data science, AI and ML.
That's where we wanna go, we want to get our stakeholders thinking about how they can benefit from predictive AI because they see the benefits to our sales organization and the benefits to our success organization.
Kelly Kohlleffel (16:20)
As many times as you can “prove it” and then show those success metrics for your organization — that’s gonna help Rocket, but it's also gonna just continue to prove the value of the data team. It's so critical. I really love that approach.
A lot of data leaders have different ways that they go about leading and building a data team. Everybody's got different qualities and characteristics that they're strong at or weak at.
What do you feel are the things that are most valuable to you when you're leading a data team?
Parag Shah (17:15)
I trust the people that I hire. I do that because I hire experts. I let those experts tell me what they think about the field that they're experts in.
When I ask them a question, I may not agree with what they respond with — but then I’ll tell them that and we'll have a dialogue. There will be times when I'll say, “I get it, let's go with that”. But there'll be times when I say, “You didn't convince me, let's move down this path.” Having that give and take with your team builds a very strong team, with a sense of trust and a sense of loyalty.
Kelly Kohlleffel (18:03)
I can tell that you enjoy this role that you're in. What do you enjoy the most? What gives you the most satisfaction about this role?
Parag Shah (18:10)
The most satisfaction that I have is when people reach out to me and say, “Parag, we need access to this thing you guys built”. That is absolutely amazing because we've built some of these things in hours. For example, somebody came to us with a request about our product hierarchy and needing more visibility. We threw a dashboard around it, super detailed, just a tabular format and it had a link to Salesforce to bring them directly to the product, giving them all this visibility.
The next day I had 70 people ask for access to that thing. That to me is just extremely rewarding because you realize that what you've built and the architecture you've put in place allowed us to turn that thing around in two minutes and it has extreme value and impact across the organization.
Kelly Kohlleffel (19:03)
I love that. And now I almost hesitate to ask this question, but what’s most difficult for you?
Parag Shah (19:10)
Convincing people that data governance is something they should care about. This will resonate with any data leader you talk to, across the board. There is a want to do things yourself, to self-serve with impunity — meaning taking data from a federated data set, taking data from a data set that you found on the internet and combining them to come up with insights.
All of those are well-intentioned, but that can create a very messy data governance issue. So understanding that tooling is generally not a problem, data is the problem. Making sure that you have a federated data set, that you use that data set properly and that governance matters — that is a battle that, I have no doubt, I will fight until I retire.
Kelly Kohlleffel (20:13)
We could spend an entire hour on that topic alone. We've got a lot of folks that listen in and are in the same situation. Large enterprises that have been around a long time and are really just now stepping in to modernize their approach to how they deliver that next generation of data outcomes. Any advice based on what you've done, what you've seen really works well, that you'd like to give to somebody that's in that position?
Parag Shah (20:44)
The advice that I would give to somebody who's in that position is to evaluate capabilities and not names. You want a company and a tool that focuses on what you're trying to solve. You want a company or a tool that is gonna innovate in that space. I’ve quoted myself before, and I’ll quote myself “If I wanna buy an electric car, I'm gonna buy a Tesla and I'm not gonna buy a Ford — because that's what they care most about.”
Kelly Kohlleffel (21:16)
Parag, this has been outstanding, and a lot of fun. I really appreciate you joining the show.
Parag Shah (21:22)
I appreciate you having me. This was a fantastic experience.
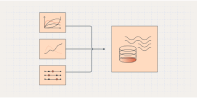
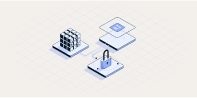
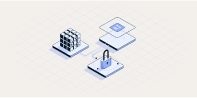