In the previous two posts of this series, we discussed the data team, including data analysts, analytics engineers and data engineers. We proposed a framework that aligned these roles from operational to informational, and we covered a variety of metrics to evaluate the success of each role within a data team. The other roles we’ll be covering in this series aren’t focused on operational or informational work but on alignment.
In this post we’ll be tackling the role of the manager in the data team, and in the next post we’ll be talking about data product owners. Both of these roles are foundational in assuring your team is aligned with the larger organization and vision, as well as internally.
Managers come in many different forms and with many different titles: team leads can be managers, directors can be managers and individual contributors can even double as managers. The common thread is that these people lead a team of contributors and its resources, whether it’s a team of one or dozens.
The goal of a manager can be divided in two:
- Ensure that your team’s skills are well used and that the organization is satisfied.
- Ensure that your team member’s skills are developed and appreciated and that the team is satisfied.
Organization alignment
The first goal is to ensure that the data team is well-aligned with the organization. In other words, are the team’s resources being used well?

In the previous post on data practitioners, we proposed various metrics that are relevant also for managers, albeit from a team-wide perspective.
- Department metrics: department-specific metrics (e.g. conversion rates, retention rates, efficiency metrics like those for ad spend or scheduling) can also be useful for measuring the success of a team; the data team isn’t wholly responsible for these metrics, but putting this metric front and center will help the team understand how their efforts are enabling others across the larger organization.
- Lead time: number of days between identification of bug or creation of request, and fulfillment of work for that bug or request; this time can then be grouped into ad hoc needs (i.e. bugs and minor requests) and planned needs (i.e. major requests).
- Data adoption: of all the resources being created and maintained by this team, how many are being used, by how many unique users and how often by those users; these three variations can be represented individually to compare between resources or they can be summarized into an overall adoption metric (i.e. how many weekly active data consumers are there?).
- Trustworthiness: internal surveys can be useful for assessing trustworthiness and usefulness of data across departments; this can be achieved by sending short surveys to your team on a semi-frequent basis (e.g. quarterly).
These metrics provide measurable ways of understanding how the data team is providing value to your organization, and how efficiently and effectively. However, as with practitioners, there are many important things that are much harder to measure.
- Empower data-driven decisions: It is imperative to increase the use of data throughout the entire organization. This can be done indirectly through office hours and enablement, or directly through managers being involved in decision making. Either way, the manager’s goal is to get data involved in decision making. The manager should personally advocate for people to use data products and allocate resources for their team accordingly.
- Standardize processes and improve efficiency: Efficient and standardized work is another objective of data teams and their managers. This is an iterative process wherein the team aims to become more efficient through new, repeatable processes and standards. Similarly to empowering data-driven decisions, this is something that the data manager needs to advocate for but also something they need to coordinate using their team’s resources. After all, even if the manager is defining and testing the process, its success is tied to its implementation by the team of data professionals.
- Stakeholder satisfaction: Alignment with the larger organization is difficult to measure. You can refer to other departmental metrics, adoption, and trustworthiness scores, but another strong indicator is simply whether or not your internal stakeholders are satisfied with your data pipeline and team. Metrics like CSAT (customer satisfaction) and NPS (net promoter score) can be useful for measuring this, but a manager should also look out for anecdotal and actionable feedback.
A successful manager ensures that their team is well-aligned with the larger organization and is making progress on its goals. Of course, there are other factors that can affect these metrics, so it’s important to account for outside changes: sometimes the goal is to improve all measures, other times it is to focus on improving just a critical few.
Team alignment
The second goal is to ensure that your individual data team members are well-aligned with the broader data team and its current and future needs. This type of alignment is also very challenging to measure, but there are some tools available.
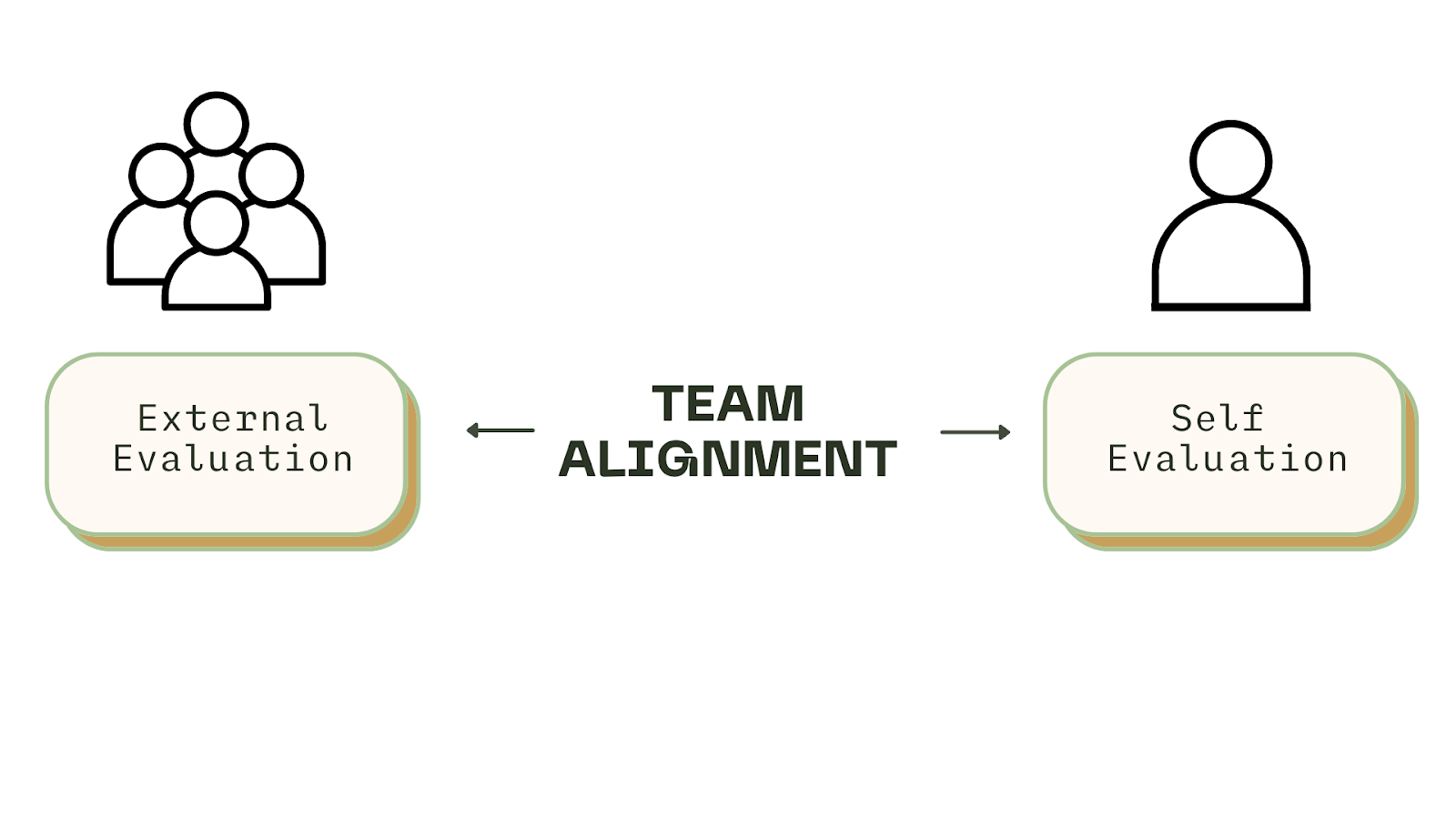
External evaluation
You should be investing in your team and you should be able to measure the progress of that investment. After all, the manager plays a critical role in upskilling and promoting their team.
This can be done by implementing a career progression framework (CPF). A CPF explains to your team how they can progress within the organization and get promoted to the next level. This sets the tone that they’re not just there to produce reports and dashboards but are valued members of the organization with many opportunities to grow. The framework should be transparent and clear on what should be accomplished at each level to get to the next. Removing all ambiguity creates trust in the process and increases motivation.
Once this is in place, it can be used to measure individual performance, but also to measure the success of the broader team and the manager. Success and progress look different for different data professionals. Timelines and ultimate destinations will differ, but in most cases teams should expect and foster progress, and the manager’s ability to facilitate this is a huge part of the role.
Self evaluation
Team members can also self-evaluate with surveys. This feedback can be about one’s own performance, about the data team or about the broader organization.
Platforms such as Officevibe can send out weekly anonymous questionnaires to each member to gauge their satisfaction on all sorts of categories such as happiness, wellness, growth, alignment and relationship with others to name a few. The platform will then aggregate the data and provide data managers reporting with an overall score and the breakdown within each category. This enables managers to assess how well they’re managing the team and areas for improvement. This self-reported information helps balance out the external evaluation of a tool like a CPF.
In the simplest terms, success for a team looks like CPF progress and high internal survey scores. However, as with organization alignment, there may be other external factors at play. Success instead may look like continued excellence in some areas, and improvement in others.
Conclusion
In the previous article, we established a framework for measuring the success of data professionals and in this post we built on top of that. The success of a manager cannot exist without the success of their team.
Given that, the success of a manager can also mean the increased success of their individual team members. By focusing on organizational alignment, you ensure that each team member is able to provide value to the broader team, and by focusing on team alignment, you ensure that each team member has the resources and feedback they need to provide that value.
Stay tuned for the next post on how product ownership can help round out the modern data team.
Montreal Analytics is a Modern Data Stack consulting firm of 45+ people based out of North America. We help our clients on the whole data journey: pipelines, warehousing, modeling, visualization and activation, using technologies like Fivetran, Snowflake, dbt, Sigma, Looker and Census. From strategic advisory to hands-on development and enablement, our agile team can deploy greenfield data platforms, tackle complex migrations and audit & refactor entangled data models.