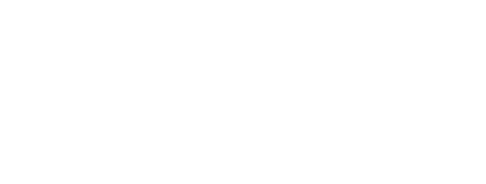
Taming data chaos in digital advertising
Tinuiti’s VP of Data Services Lakshmi Ramesh on modernizing its data stack to provide world-class marketing services to some of the biggest brands.
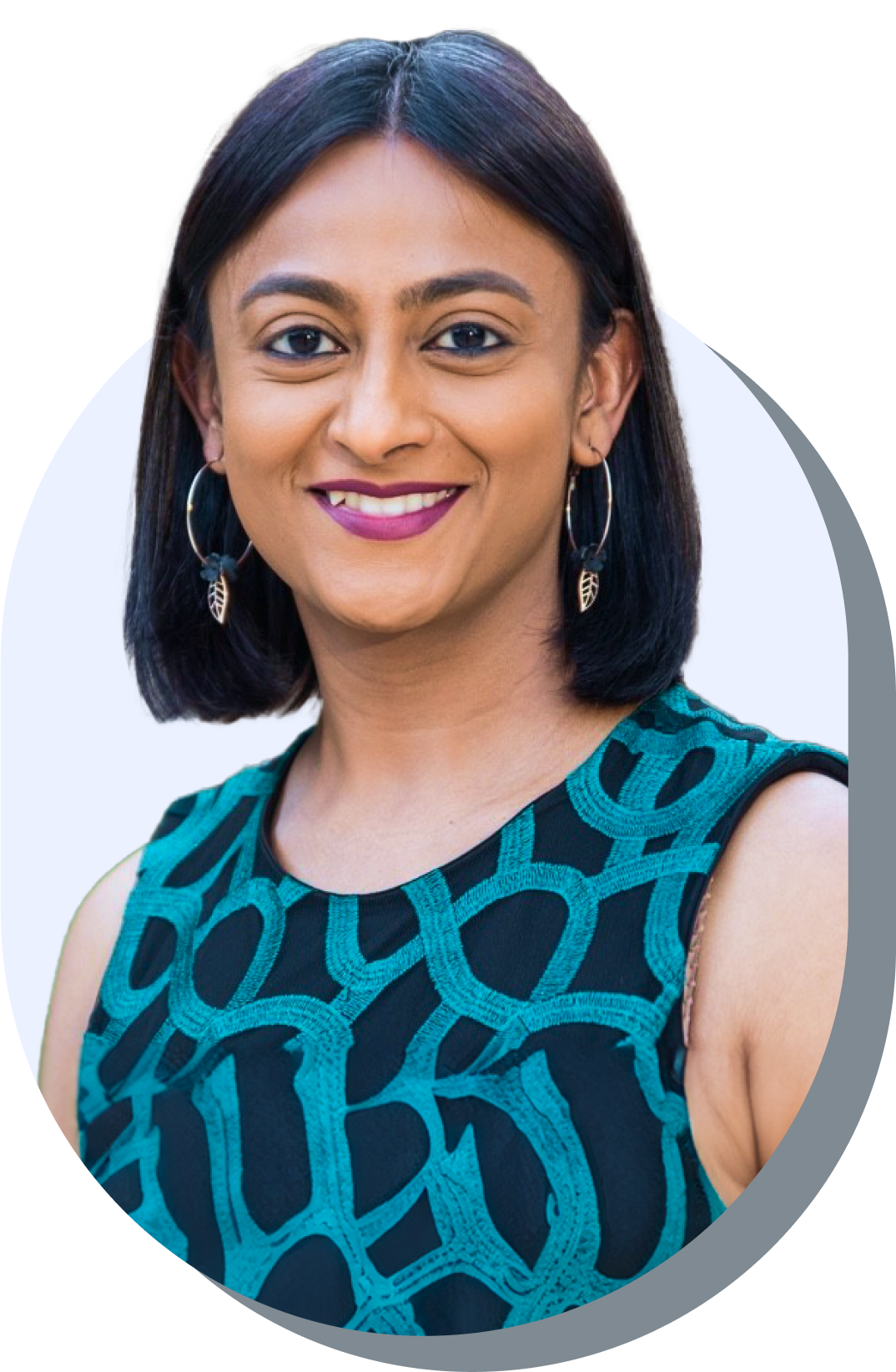
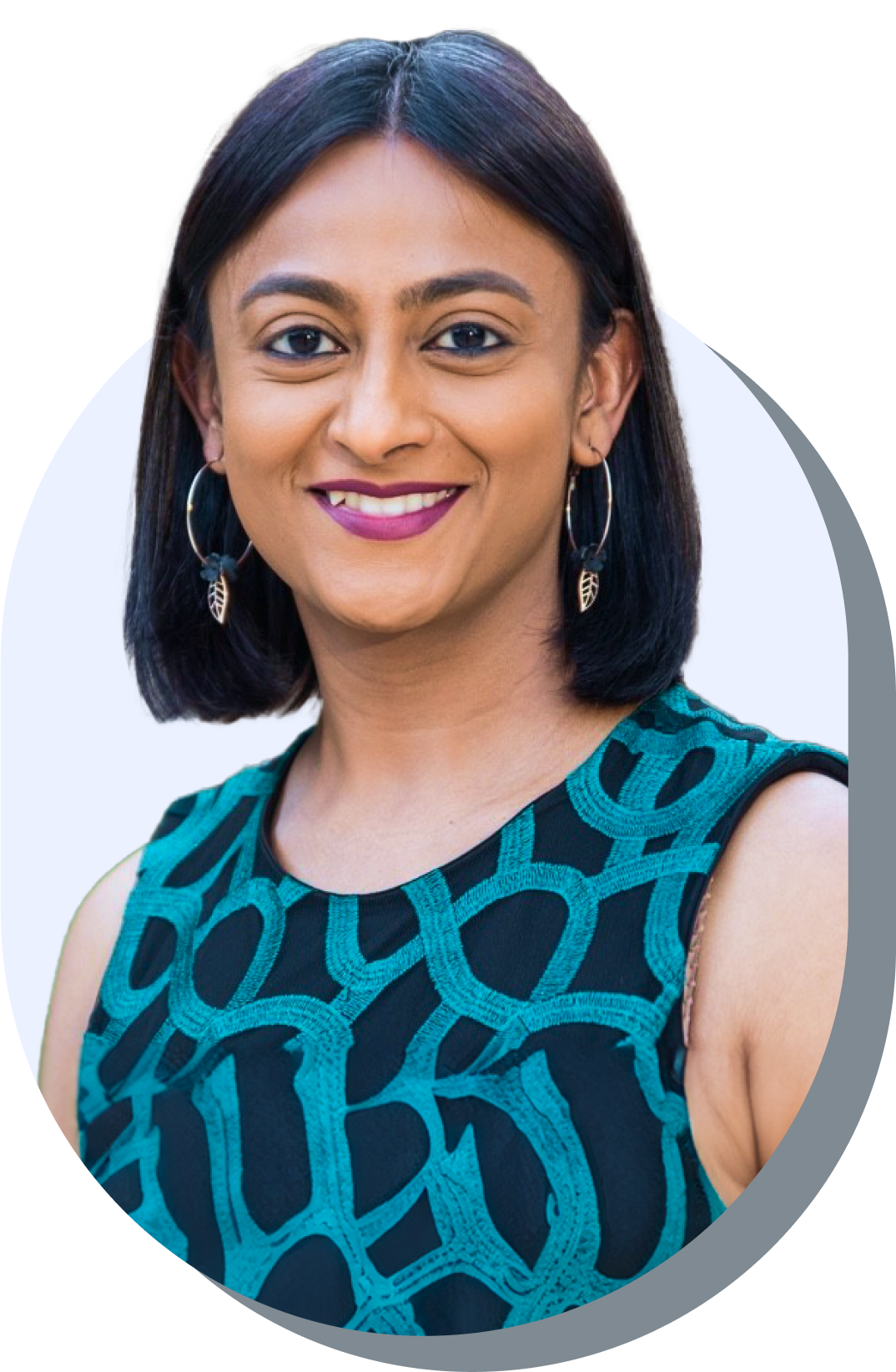
More about the episode
Modern marketing is constantly growing in complexity due to new technology, privacy requirements and analytics opportunities. That’s why the right data integration tools are essential to all of today’s digital marketing endeavors.
Lakshmi Ramesh, Vice President of Data Services at Tinuiti, which services brands like Rite Aid, Nestle and Instacart, joins us to discuss how the company manages the data from hundreds of platforms to serve both clients and internal teams.
“The data space is constantly evolving. You want to constantly be reassessing and continuing to modernize your stack.”
Ramesh has helped build a data stack that enables Tinuniti to more efficiently collect and synthesize data. This translates to better marketing results for their clients. Here are some key takeaways from the conversation:
- How Tinuiti reduced time to market when sourcing data and making it available to end users
- Why it’s important to focus on end users rather than fixate on specific tools
- How a data lake helps you build specific workflows for known use cases as well as uncover new ideas
Watch the episode
Transcript
Kelly Kohlleffel (1:36)
Hi folks, welcome to the Fivetran Data Podcast. I'm Kelly Kohlleffel, your host. Every other week, we'll bring you insightful interviews with some of the brightest minds across the data community. We will cover hot topics such as AI, ML, Gen AI, enterprise data and analytics, data culture and more. Today, I am pleased to be joined by Lakshmi Ramesh. She is the VP of Data Services at Tinuiti.
She and her team deliver a range of data-centric solutions for marketing and advertising, they’re involved in end-to-end data outcomes. That includes data collection, data management and data consumption. Prior to Tinuiti, Lakshmi spent time with IPG media brands, Treasure Data and Cadrion in various roles. Lakshmi, it is outstanding to have you here today. Welcome to the Data Drip podcast.
Lakshmi Ramesh (0:45)
Thank you so much, Kelly. It's a pleasure to be here.
Kelly Kohlleffel (0:52)
Outstanding, spend just a minute or so, and dive into what you are currently doing at Tinuiti. And what Tinuiti does as an organization, for those who aren’t as familiar.
Lakshmi Ramesh (1:02)
Tinuiti is a full-service performance marketing agency. We run across various channels for our clients. My team is responsible for our underlying data stack. My team is comprised of data engineers, data quality professionals, BI developers and data operations folks, who help build and maintain data stacks that collect data from hundreds of marketing platforms, bring that together and synthesize it to derive data outcomes for our clients.
Kelly Kohlleffel (1.37)
Incredible. The background that you have there and with Treasure Data, IPG media brand, etc, what initially drew you into this career in technology? But then also the marketing side?
Lakshmi Ramesh (1:49)
Growing up, I was heavily influenced by my dad. He was a biomedical engineer by profession, but he used his spare time assembling computers from scratch, playing video games and programming. Very early on in our lives, he taught us basic programming and the fundamentals of computers. It was no surprise that growing up, I chose to major in computer science as I was getting ready for college. That’s how I got interested in the world of computers. Moving from there, I really started playing with data during my master's degree.
I was fortunate to work with one of my database professors at my university, and she invited me to join a project in partnership with the Plant Sciences Department. It was a bioinformatics-based project where we were making it easier to analyze plant genome data.
That was my first chance to see how I could put all the theories that I learned about databases and data structures into practice and generate real value. That had me hooked on data, so I decided to pursue a career in the data space. Marketing and advertising happened by chance for me. In the 2010s, when RTB, programmatic was fairly new, and trading desks were just starting to become a thing.
I landed a role at Cadreon, and they were part of IPG, the Interpublic Group holding company. I was one of the first technology team members there, and we were building our data stack ground up. It was a very steep learning curve for me because those days you didn’t really have a lot of marketing and advertising-related resources. I remember sitting in conference rooms with seasoned marketers and was amazed by the amount of data that was being crunched behind the scenes all to serve up an ad. That was fascinating to me, and ever since — all of my roles have been at this intersection of tech, data and marketing.
Kelly Kohlleffel (4:11)
From bioinformatics and plant genomes to marketing. Very interesting, what have you seen since you’ve been in this space for a while? Have you seen any interesting developments?
Lakshmi Ramesh (4:27)
Marketing and advertising has been one of those spaces that have evolved quickly and rapidly over the last few years. From the rise of social media and the heavy emphasis on influencer marketing, the rise of AI and more emphasis on personalized engagement across all brands. So from a data perspective within the marketing and advertising industry, there are two big shifts. One is the increased emphasis on privacy with regulations like GDPR and CCPA, etc. coming into play. It's redefining how we think about and handle consumer data.
There are also a lot of restrictions with third-party tracking, which puts more emphasis on first-party data for brands. There is an increased need for brands to handle and collect their first-party data more effectively and use that towards driving marketing outcomes.
For example, Treasure Data is a CDP company, so my role involved working with a lot of brands to help them centralize first-party data across all of their different consumer touch points. So at least they had a streamlined data structure to start analyzing and utilizing to drive better marketing decisions.
The second shift is the heavy use of data science and now AI. Particularly for digital marketing and analytics. There have been a lot of good applications of data science, from forecasting to predictive analytics. How do we get better at predicting the next best dollar and where it should go? That's been quite fascinating to see, and I think it will continue to evolve and grow.
Kelly Kohlleffel (6:29)
That’s interesting because when you talk about both of those developments, the privacy side and the expansion of data science or AI-type workloads, all of those have to do with an ever-expanding range of source data systems. At Tinuiti, have you seen more demand for these and does it put pressure on ensuring you've got the privacy side taken care of?
While also ensuring that you deliver these data science and AI workloads? Are those data sources expanding significantly?
Lakshmi Ramesh (7:06)
Yes, absolutely. We probably bring data from hundreds of different platforms today. That's where our primary challenge is, with respect to data — the variety. There are so many different marketing and advertising platforms that we have to bring data from. We also have to bring data from client systems and other third-body data sources so we can provide a more holistic measurement solution.
Kelly Kohlleffel (7:36)
I really agree with that. I say that a lot. I think more so than the volume, it is the variety today. It seems like every single business unit within an organization is getting hit with yet another data source across a different category and it's creating this huge challenge. You alluded earlier that delivering these personalized experiences, obviously for Tinuiti to stay competitive.
What does your data stack look like today? How are you delivering data today across that data stack to the various consumers that you have at Tinuiti?
Lakshmi Ramesh (8:11)
So I think of our data stack sort of in largely three broad components. There's the data collection and the data sourcing side. There is how we manage and store all of that data. Then there is “how do we make data accessible?”, so the data access side. So if I talk about the data collection side, because we've got variety as our challenge and so many different sources to integrate, we've adopted sort of a hybrid approach to data collection.
What I mean by that is, that there are some of our top platforms that we have internally built API connectors so that we have greater control, flexibility and customization capabilities. For a lot of the others, we've leveraged platforms like Fivetran that give us the flexibility to enable self-service connectors and quick out-of-the-box plug-and-play connectors. That helps us reduce the time to market when it comes to data sourcing and making it available to end users. On the data management / housing side of the house, we've largely been an AWS-based stack. So we leverage S3 as our data lake, and then we use Spectrum and Redshift on top of that.
We've also built all of our analytical data preparation transformation workflows using dbt. And that's worked well for us to make our pipelines more modular, more streamlined and more reusable. Lastly, on the data access side of things, we leverage Tableau as our visualization front end for a lot of our client-facing dashboards and our internal dashboards as well.
SQL is used heavily across the organization for more of the ad hoc analytics exploratory use cases. We use R and Python for more advanced analytics. And then we're also starting to explore other solutions to make data available to sort of non-technical users in the company.
Kelly Kohlleffel (10:14)
Very interesting. Delivering the capabilities you have with Tinuiti today would have been exceptionally challenging 10 to 12 years ago given the tools and infrastructure we had at that time.
Lakshmi Ramesh (10:27)
Absolutely, I think that’s part of the challenge of being in the data space, it's constantly evolving. So you want to stay ahead of things. You want to stay on top of “Is there a better way?” And constantly be assessing that to continue modernizing your stack. That’s the big challenge in front of all of us.
Kelly Kohlleffel (10:48)
When you go into a new year, do you look at those top one, two, three areas, and you say, “Look maybe we have these few areas around the tech stack, around process and around skill sets if we want to improve.” And kind of look at it that way to get this constant improvement process going as Tinuiti?
Lakshmi Ramesh (11:10)
Absolutely. I think that modernizing our foundational data layer has been a huge focus for us over the last year or so. That’s because we knew that if we were going to build data products at scale, we had to get our basics right. We've been tackling problems like, how do we get quick and easy access to a new data source, for example.
How do we make our data pipelines, data preparation and transformation quicker and more efficient? How do we reduce the time to market for sourcing data from a data source and making that available to end users? Those questions have been a big focus for us over the last year or so. Looking forward, we will likely take that a step further and say, “How do we democratize that data across more people in the company? How do we build more apps and products from the data that we have centralized? How do we leverage AI capabilities?”
Kelly Kohlleffel (12:10)
You mentioned using S3 and a data lake approach, there are so many benefits to going down that path. Can you talk about the diversity of data workloads and applications and the ways that you're trying to deliver value around data products? What are you most focused on right now? What's important to you as you're building and delivering these data products and services?
Lakshmi Ramesh (12:36)
For me, from a data outcome perspective, I think the big focus needs to be on the end user. It's easy to get caught up in new and shiny tools out there. Or that engineering mindset and be focused too much on the how, instead of the why.
It's also easy to get caught up in thinking you know what your audience wants, but then only to build it and find out that's not what they wanted. We should all start with the users and consumers in mind and focus on how to build better products that can enhance their day-to-day workflows, bringing new value to them with the data that they're leveraging.
Kelly Kohlleffel (13:30)
How do you stay grounded in that “why”?
Lakshmi Ramesh (13:33)
Yeah, it is a challenge. Especially at our scale, we've got 12 to 15 different channel and media teams, cross-channel strategy and analytics teams that we work with. We have a very diverse set of user base and the end clients to consider too. It’s important to keep a pulse and be on top of what their day-to-day workflows are and their struggles are.
We've been doing a lot of forums where users can share how they manage a particular account. What are the data points that they leverage? What is the impact that helps them derive? Getting a deep understanding of their workspace and putting yourselves in their shoes, is the first step to being able to draw up a roadmap and solve some of those problems.
Kelly Kohlleffel (14:36)
I love that. Could you talk to me a little bit about how you're incorporating a data lake approach into that overall data delivery?
Lakshmi Ramesh (14:42)
The biggest advantage that it provides is allowing you to build specific workflows for your known use cases and allows you access to data for the use cases that you've yet to discover. That’s kind of how I think about a data lake essentially. At Tinuiti, we bring all the data that we possibly can, store that in our data lake, build specific workflows for the known use cases and then allow users to interface with the other data and perform exploratory analysis so we can uncover new use cases and product ideas.
Kelly Kohlleffel (15:28)
Very nice. I know you talked a lot about the AI and ML space. Is there anything new you’d like to discuss whether it’s a recent development or a vision you have for the upcoming year?
Lakshmi Ramesh (15:44)
The big one I’m really excited to research and experiment with is more on the BI and the insights side. The ability for us to take data and bring efficiency through an SQL copilot, for example. The ability to leverage large language models towards insights generated and do that in a more automated fashion. A more natural language way of asking questions for analysis. These are the areas that I'm most excited about digging deep into in 2024.
Kelly Kohlleffel (16:24)
Very nice. You've led a number of data teams and you're leading one now. For those listening out there and wanting to move into this area, what qualities are most important to have when you're leading a data team?
Lakshmi Ramesh (16:37)
It's important to stay ahead in this space. You have to constantly, like I said earlier, keep a pulse on your audience and what their struggles are because that's what's going to help you define a vision, roadmap and priorities for your data team. It's also important to be able to constantly learn and respond to change.
We talked about how quickly the data landscape has changed over the last few years, and we have better capabilities now, and that's only going to keep evolving. Learning about what's out there and how can I incorporate that to generate value, I think is going to be huge. In addition to that, I feel like data teams often struggle with the difficulty of tying outcomes that your data team is generating to general company metrics like revenue or margins, etc.
While you can leverage some metrics like driving operational efficiency for the teams by automating certain things, or reducing churn and things like that, I think it's important to build a narrative for your data teams around how data is helping the company advance and providing value to clients at the end of the day. And tying your vision to that narrative and constantly reminding your teams of that. I think that is huge.
Kelly Kohlleffel (18:10)
I love that. I think it goes upstream as well. That same narrative that a data team rallies around is the vision. If you've done it right and tied it to the company overall, then that can go up to the executive team as well. I would imagine that the story stays the same as you're sharing it both ways.
Lakshmi Ramesh (18:31)
Exactly.
Kelly Kohlleffel (18:32)
You talk about staying ahead and learning, honestly, I’m struggling with this. It seems like every day I’m falling behind, there’s always something new. What do you do to stay ahead?
Lakshmi Ramesh (18:46)
It's hard. There's a deluge of information nowadays so it’s hard to keep up. I think Tinuiti does a fantastic job at corralling resources across the entire agency and making them available. Whether it's case studies or webinars, there's a ton of material that's available out there.
We have a lot of marketing partners that do a good job with this – Google, Facebook, etc. – they have really good learning content out there. There are also several blogs I follow, and several Youtubers I keep up with. And of course I listen to data podcasts like this. It keeps me inspired. Yes, it provides a learning avenue, but it mostly keeps me motivated and inspired.
Kelly Kohlleffel (19:39)
Yeah, I agree. If you look back over your career, is there a leadership lesson that stands out to you? One that is unique to you being a female leader in the data space?
Lakshmi Ramesh (19:52)
The biggest lesson I've probably learned personally is just embracing the fact that I'm a female leader. Embracing that feminine side. Part of being a female leader is that certain unique skills may come naturally. I don't want to generalize here, but I do think that there are unique skills that female leaders bring to the table.
For example, their approach to negotiation, conflict resolution and bringing empathy to the workplace. Embracing that, knowing that you're bringing that to the table and leveraging that towards making better teams. At the end of the day, regardless of gender, all of our jobs as leaders is to find that complementary skill set that we can put together in a team and make all the jigsaw puzzle pieces fit together because that's how you have the biggest impact.
Kelly Kohlleffel (20:57)
A lot of times I default to, “Hey, I’ve got to learn some new technology”, but you’re right. There are so many things that as leaders, regardless of your background, you can learn. When you answered that question, you mentioned empathy. I think everybody can do better by being more empathetic. Those are great learnings over the years, certainly, something we can all take and use.
This has been a lot of fun. I have enjoyed this Lakshmi. I appreciate you joining the Data Drip and I look forward to keeping up with everything you're doing at Tinuiti.
Lakshmi Ramesh (21:42)
Thank you so much, I'm grateful to have been a part of this.
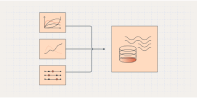
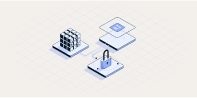
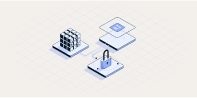