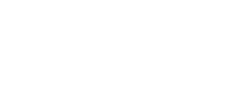
How the modern data stack powers a $45 billion global agribusiness
CHS Inc.’s Senior Manager of IT Engineering Riley Buss shares how Fivetran, Snowflake and dbt are enabling the business to reap real-time analytics on terabytes of data from across the globe to revolutionize its supply chain management.
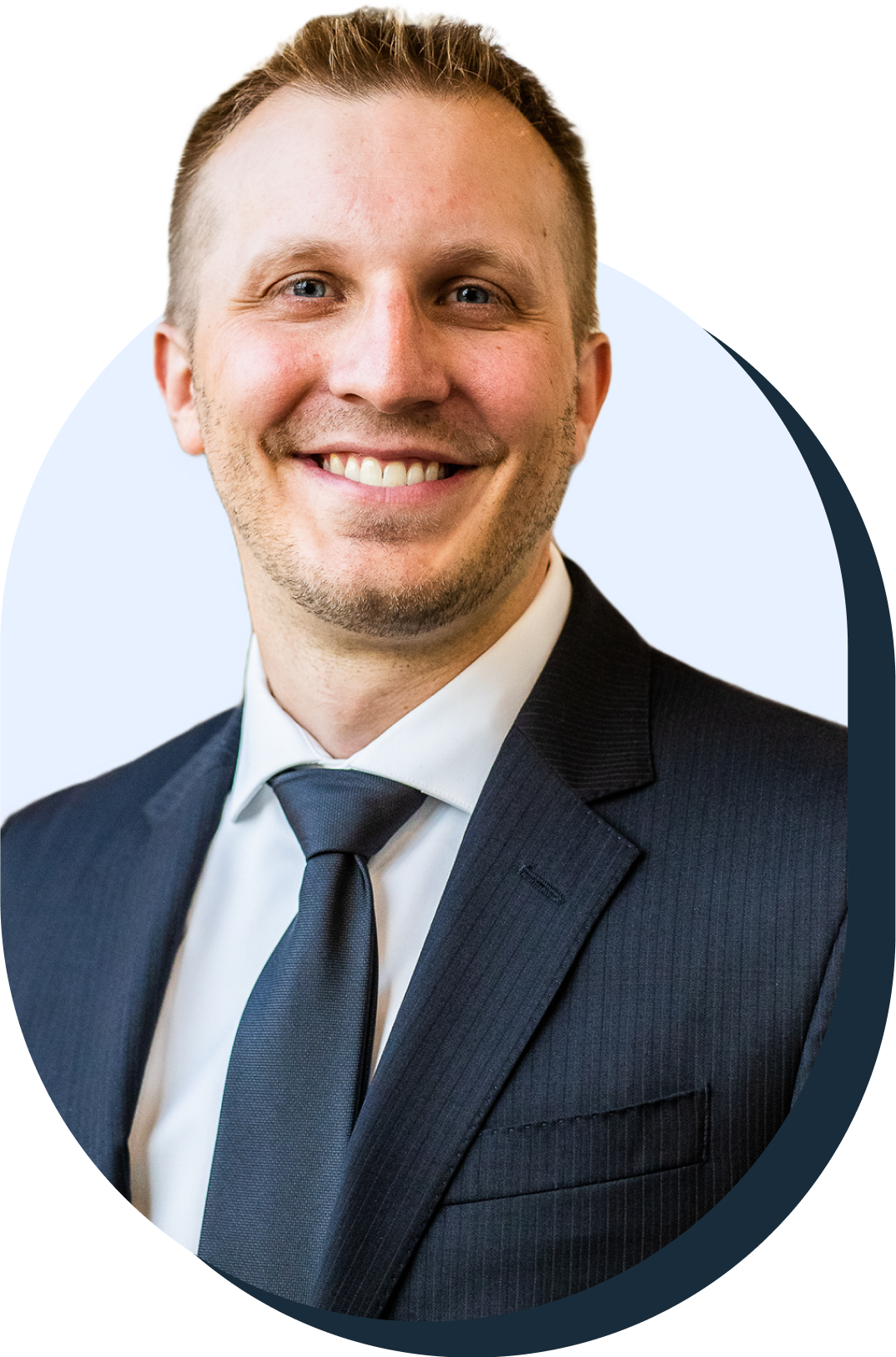
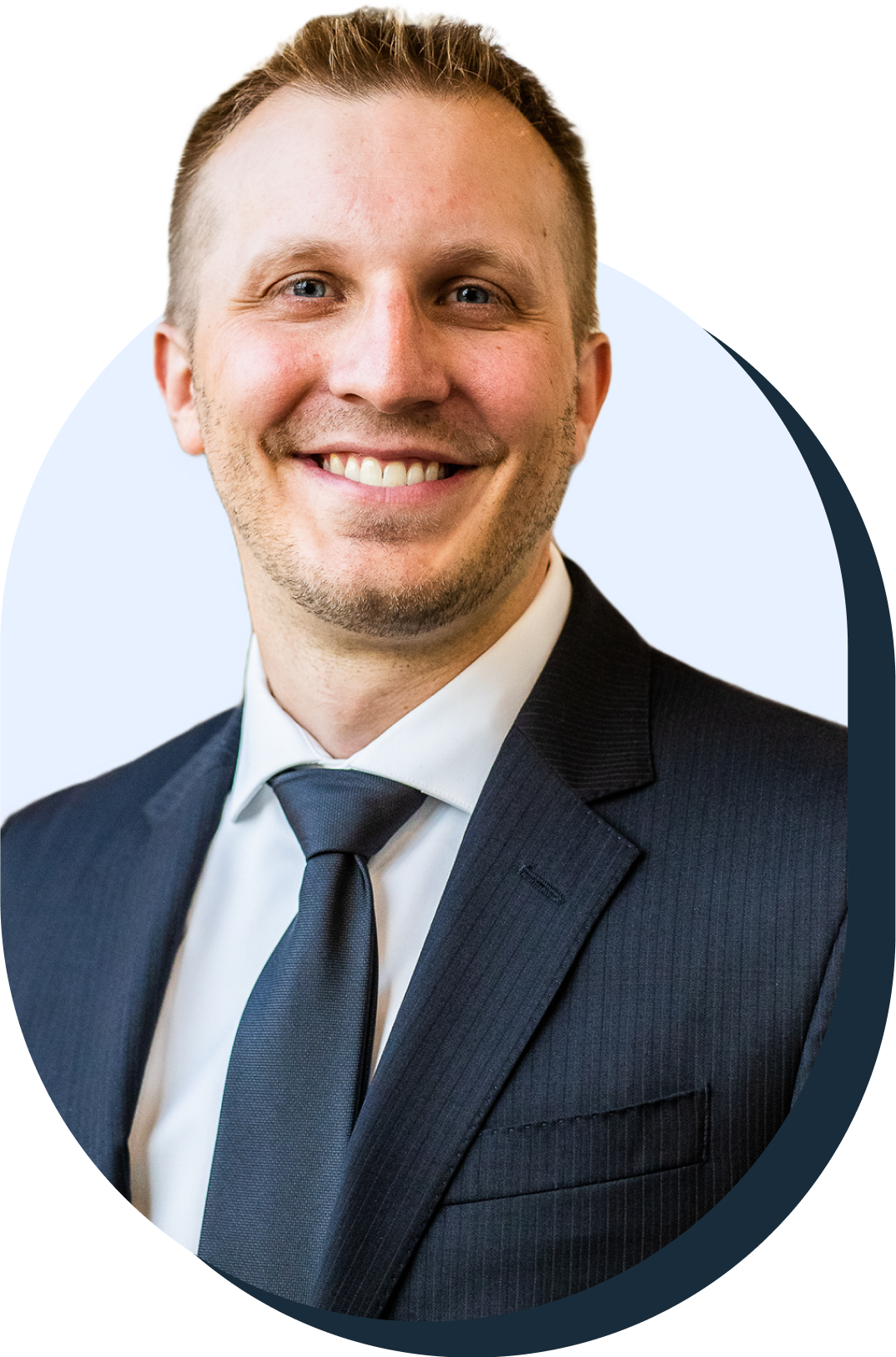
More about the episode
Whether it’s a grain elevator, river terminal or oil refinery, CHS, Inc. is collecting vast amounts of data from countless sensors and devices. The $45 billion agriculture cooperative uses that data to deliver real-time insights to farmers and supply chain managers with the goal of bringing the highest quality and most sustainable food to the table.
CHS Inc.’s Senior Manager of IT Engineering - Analytic & Automation Riley Buss shares novel insights into how the Fortune 500 secondary cooperative is leveraging modern data practices and technologies to drive sustainability and efficiency into the global food supply. He discusses the challenges of real-time data collection across 50 business applications and 14 different ERP systems, and highlights some innovative use cases for drones, cameras and computer vision for replacing hazardous human labor.
“We leverage Fivetran to do CDC off all our data, stream it into [Snowflake] and provide real-time analytics back to our business. That was one of the game changers when we moved from Cloudera to our new data stack.”
Dig into this insightful conversation to learn how:
- The modern data stack provides real-time insights that impact efficiency and sustainability in agriculture
- Fivetran HVR enables a move to CDC-based ingestion, improving data quality on vast datasets
- Servant leadership encourages collaboration between data experts and business users to drive innovation
Watch the episode
Transcript
Kelly Kohlleffel (00:06)
Hi folks, welcome to the Fivetran Data Podcast. I'm Kelly Kohlleffel, your host. Every other week we'll bring you insightful interviews with some of the brightest minds across the data community. We're going to cover topics such as AI and ML, GenAI, enterprise data and analytics, various data workloads and use cases, data culture and a lot more. Today, I am exceptionally pleased to be joined by Riley Bus. Riley is the Senior Manager of IT Engineering at CHS.
You don't know CHS, they are a diversified global agribusiness cooperative. They're owned by farmers and local co-ops across the U.S. Riley in his current role manages multiple teams consisting of data engineers, data scientists and RPA developers. In 2021, he led the data platform migration from an on-prem, cloud-era platform to a cloud-based platform consisting of best-of-breed tool sets from Fivetran, Snowflake, dbt, Dataiku and AWS.
Since moving to the new platform, his teams have brought new insights and capabilities to the organization's key digital transformation initiatives. Riley, it is great to have you on the show. Welcome in.
Riley Buss (01:13)
Yeah, thanks, Kelly. Glad to be here today.
Kelly Kohlleffel (01:15)
Absolutely. Well, I previewed a little bit of CHS. A lot of us may not be as familiar, its a really interesting company with a huge breadth and depth of products and services, energy, crop nutrients, grain processing services, all types of things, even insurance and financial services. Give us a highlight — dig in a little bit more on CHS. What does the company do? And then a little bit about your current role as well.
Riley Buss (01:41)
Yeah, for sure. So yeah, CHS is what makes it also unique is that it's cooperative. So our owners are also our customers. So it's all centered around providing value back to them, the farmers, the cooperatives that really own us. So when we look at the services we provide, centered around the farmer, we do everything from providing them the kind of the crop inputs that they need to be successful, from seeds to crop nutrients, to crop protection, providing services through the crops while they are growing. And then, on the energy side, we're providing them with diesel fuel, gasoline and lubricants. If people are familiar with the Cenex brand, that is our flagship brand on the energy side. That's probably a little bit more known to folks, but really it's all providing value back to the farmers. So then in the fall, after the crops have grown and they're ready to harvest them, we do the grain merchandising for them and really take their grain and make it accessible to the global markets and give them that outreach.
Kelly Kohlleffel (02:34)
I was thinking you mentioned after harvest, what happens after harvest leading up to? Do the services that CHS focuses on shift a little bit or how does that work?
Riley Buss (02:45)
Yeah, so being as diversified as we are across all the agronomy crop inputs, grain merchandising on the outputs and the energy that kind of surfaces the breath of it. Really, it's a very large supply chain company. We're basically moving logistics of all their goods and services that they need. And that's all around the calendar year and all around the globe.
Kelly Kohlleffel (03:05)
Yeah, so you really don't get to take a little breather after a particular time of year, it’s just constant.
Riley Buss (03:12)
No, nope, the sun never sets on CHS and it's always busy.
Kelly Kohlleffel (03:15)
Yeah, okay, in your role, give a little background, kind of what also interested you about CHS and then what are you doing right now?
Riley Buss (03:34)
Yes, what really attracted me about CHS is I actually grew up in agriculture. I grew up on a dairy farm in a small town in Wisconsin. I went to school and I ended up with a criminal justice major and an MIS minor. But I really had a passion for IT and then dove into IT. Basically, CHS is my second job out of college. I started off more on \the business analyst side. And then I had a love for data and then grew up through the development ranks to leading a few different teams and then got to the position where I'm at right now.
Kelly Kohlleffel (03:55)
Really good. You know when you think about agriculture. I mean – to me – in every industry, if you're not able to use data in innovative ways and new ways every day, you're kind of falling behind. Provide some examples if you can on maybe just two or three of those where CHS is leveraging data and technology to drive innovation for the cooperatives and the farmers that you support.
Riley Buss (04:22)
Yeah, for sure. So a lot of people might think of data and agriculture and they might jump to Precision Ag. Precision Ag is all about maximizing the yield per acre, using all kinds of cool technologies and data to do that, but it really surfaces a much broader use case than that. So when you look at CHS, we're a very asset-heavy company. We have grain elevators, we have river terminals, we have gateway ports, we have processed food ingredients, which has soybean crushing facilities and we have ethanol refineries.
We have refined field refineries. So all those assets are littered with IOT devices, PLCs, GMIs that all are kicking data off of it and all that can be used. We also have – which is also one of our challenges – we have 14 different ERP systems that we also need to collectively get one view of the whole of.
Kelly Kohlleffel (05:12)
Wait, wait a minute. 14, how do you deal with 14 ERPs? That's crazy.
Riley Buss (05:18)
It creates job security, that's for sure. No, it's very challenging. One of those actually has 36 instances of itself distributed, which is its unique challenge. But yeah, we've been on an SAP journey since 2015, and it's been a fun journey. We're making progress there. Still got a little ways to go, but in the meantime, we're still getting all the use we can out of some of the legacy ERPs.
Kelly Kohlleffel (05:41)
Are you going to make it to a single global instance of SAP during your tenure at CHS?
Riley Buss (05:46)
I think the visions and the future are there for us. We'll end up on two different instances of SAP, just because our Ag lineup and our energy lineup are a little bit unique, especially for how SAP services the Ag side with our agricultural commodity module. So we'll have an Ag and an energy instance.
Kelly Kohlleffel (06:04)
I think you mentioned precision agriculture. I think of – and tell me if I'm wrong – efficiency and effectiveness and you know, how do I manage my resources best? Am I on the right track there? And if so, you know, where are you seeing precision agriculture mainly being used?
Riley Buss (06:25)
Yes, so definitely it also ties into sustainability too. We want to be very mindful of what kind of chemicals or seeds we’re using to make sure that they're the best kind of fit they need to get the maximum yield, without kind of over using any type of ingredients over each other. We're going to be very mindful of that. So making sure we can still get the most yield per acre, using the latest and greatest technology like drones to do things like that too.
What's fun about drones is we, you might think of that as, “Oh, I fly it over a field. I can see different dead spots or maybe different types of plant health.” But it also goes back into surfaces that need us. But because we have a lot of different lines of businesses. We also have, you know, many assets. We actually use them for asset inspections as well. So we have a lot of grain elevators. They're made out of concrete and steel. We'll check them for cracks and we'll use some computer vision to track that. Up in Superior we actually ran a fun use case where it is an attachment for a drone that made it look like a big light bulb. And we're able to fly that inside the grain elevator and inspect the inside, which is something that is very dangerous to do if we're doing it the old way of having a person go in there. But now we can also do it much more frequently. So we're able to keep the plants and everything much safer and much quicker without putting humans at risk.
Kelly Kohlleffel (07:45)
Oh my gosh, that is incredible. I'd love to be that — eh, maybe I wouldn't want to be that drone operator. And I got to imagine the data being churned off is pretty substantial.
Riley Buss (07:56)
Yeah, there are terabytes of data that make it fun to figure out just the strategy for how do you do, you know, ML at the edge? Where are you moving your data? What frequency? What value are you trying to get out of moving it? Because every time you move data, it takes processing to do it. So you have to be mindful of cost from that perspective. And also every time you move data, it's never going to really increase in quality. So you want to make sure that you have the right process to put in place for it, based on your use case and what you're trying to solve.
Kelly Kohlleffel (08:22)
And you mentioned some of the edge processing going on. I mean, a lot of times your constituent base, the farmers and even the cooperatives may not be sitting smack dab in the middle of a big city with perfect internet service, right? Do you find that that's a challenge when you start talking about different types of data capture via a sensor at the edge, if you will?
Riley Buss (08:51)
Yeah, that is a challenge. I would say we're in a much better state today though, with those challenges than we were eight years ago when I started. When I started eight years ago, we still had some sites that were on old T1s that had very slow bandwidth. Some of their ERPs even ran on Citrix, so that way they didn't have to have a local client of that app installed that was communicating traffic over the web. That has improved, or there's been a good push towards rural broadband, and that's made a lot of what we do easier, but still, we have areas that we need to be very mindful of, you know, low bandwidth, low connectivity. “How are we capturing that data? How are we transmitting it?” and so on.
Kelly Kohlleffel (09:27)
Let's talk a little bit about some of the technologies that you use, would love to dig in a little bit more on your data tech stack and how you deliver data products and data services today. But then also, where are you going with that tech stack and the way you deliver data services and products?
Riley Buss (09:45)
Yeah, so when we look at our data landscape, we have, like I said, 14 different ERP systems. One of them has 36 different instances of itself. We have over 50 lines of business applications. We also have a bunch of SaaS apps and external data that we want to bring in to enrich our data. So it creates a nice, fun data engineering challenge. If it's a database that we have either on our on-prem data centers or in our public cloud, that's where we're going to leverage Fivetran HVR to do CDC (Change Data Capture) off that data, to stream it into our platform and provide real-time analytics back to our business. That was one of the game changers that we did when we moved from Cloudera to our new tech stack. On Cloudera, we were leveraging either Spark or Scoop to do those ingestions. They were more time-consuming, more resource intensive. Being able to do ingestions off of Change Data Capture logs really reduced the amount that we were hitting our source.
So our DBAs were much happier when we moved to CDC. And then also our end customers were happier just because we're providing them more real-time access to data.
Kelly Kohlleffel (10:51)
Yeah, I spent a pretty considerable time in the Hadoop world as well. And I think that the thing that was toughest was “how do I get predictability and standardization when I am building, you know, Sqoop pipelines or Java Scala Spark pipelines?” And again, trying to get to this level of “let's build this once, use it many times” and it sounds like you're starting to get there. Where you build something, you know it's going to work and it's going to be very predictable for those downstream users that you have.
Riley Buss (11:21)
Very predictable. And then we also need to make sure it's accurate information too, right? With, especially back when it was, you know, Sqoop and Spark and you're doing more batch style ingestions, it's easier to manage data quality just because you're able to do kind of point in time, you know, are we matching your source? When you move to CDC and you're streaming that information through into, you know, we land that data directly in Snowflake, but it could land in S3 or wherever.
It creates a more unique challenge of, “how are you ensuring that you're capturing hard and soft deletes of the database correctly.” And that's one where Fivetran really stood out, because it has the compare and repair functionality. So, we can schedule on our HVR hub to have a compare done. We can have it iterate out like, “Hey, here's a statement that you would need to make that data whole again.” But it also ensures that when we bring the data into our data lake, and data warehouse platform with Snowflake, we know that it's matching the source and we're starting with that good integrity.
We do have, kind of a process layer that will make sure that we're deduping anything happening, like handling those deletes effectively. Then that's when we jump into data products. Typically how we build data products is leveraging dbt. We're leveraging their cloud solutions, so dbt Cloud, and making sure that we adhere and make sure that we meet their data quality.
Concerns as we continue to add business logic and application logic to the data products we build, we do try to take advantage of the dbt tests. So leveraging their unit tests and automating those to ensure every time we want to run a dbt test run, we can do that in an automated fashion to help us have a scalable, sustainable future. So really kind of starting with that test-driven first approach to development.
Kelly Kohlleffel (13:02)
When you're building and delivering these data products, I heard scalable just then. What does become most important to you? Because there's a speed aspect of being able to deliver, but you've got to have the quality, you've got to have the scalability. What do you take into consideration at CHS most?
Riley Buss (13:21)
You need to take a broad consideration. So one of the things that you might think about that or might not think about is, we're streaming this data in with CDC, we can provide real-time analytics, but our business processes might not be real-time to enable some of those real-time analytics. For example, maybe a back office accounting or finance team does a business process once a week, once a month or once a day, and that won't be articulated in those ERP systems until those business processes happen.
So just because we're ingesting data in real-time, we need to be mindful when we're gathering requirements to the end stakeholder that, how the business actually functions on those processes is taken into account, in the timeliness and the KPIs that they want to be able to see and ultimately make decisions off.
Kelly Kohlleffel (14:07)
I really like that. What you just described flows down into how you may define success for a particular data product or service that you're delivering at CHS.
Riley Buss (14:17)
Yeah, so how I determine success for any data product that we deliver is, “is it implemented in the business process?” and “how is it implemented?” Is it something that is improving what they had before? Are they spending reduced time doing their old process or are they getting more information and able to do it better? But, ultimately, how is it being embedded in the business process and not just being, “Oh, I have this report out here that I can use sometimes.” It's no, that report needs to be embedded in that business process to make sure that we're getting the value out of delivering it.
Every data product we build is another data product we also have to support. So we want to make sure that it's being leveraged to its full extent and is providing its value, meeting what it was designed to do.
Kelly Kohlleffel (21:52.982)
Yeah. And is that an evergreen process to a degree, Riley, where you, you know, you go along for a couple of quarters or maybe a year and you want to revisit? If so, is this something, it's kind of built into the process of revisiting these success metrics and ensuring that you've got this continued alignment with the business?
Riley Buss (15:17)
It is, and right now we kind of do it manually and ad-hoc, but right now we're actually implementing a data catalog. And that data catalog is going to be key to enabling a sustainable path for that process. Because essentially we'll have basically broken down by our value stream, workstream at CHS, which data products have been used by which customers. We have the data catalog, we'll get better visibility into “how are they actually using it?” And “We delivered it, are they using it?” And we'll see the usage reports.
Are they actually querying the information? Are they using that Power BI report or that embedded analytics? And that will be conversations that we have with our product owners on the business side. When we meet with them, it'll be reviewing that saying, like, “Hey, we built this for you, spent a lot of time and money on data engineering to do that. We're not seeing that you're not using it. Is there some additional enhancement that we need to be doing there? Or should we look at decommissioning because it didn't hit the mark or maybe the business priorities have changed and that's no longer as valuable as it once was?”
Kelly Kohlleffel (16:17)
Yeah. And you know, those shifts can happen in, like you said, unless you've, even if you're doing it more on an ad-hoc basis, unless you're having those conversations, it's easy to go along and spend some cycles, and not necessarily hit the mark. So I love the fact that you're coming back and talking.
Are there areas that you're primarily focused on right now, data workload-wise that maybe we really have not taken advantage of before?
Riley Buss (16:48)
Right now I have five different capital projects running on my team. So that's a lot that is currently underway in active development.
What's been unique is what Snowflake has given us is we have two of those, which are actually working with other partners of ours and actually doing a direct Snowflake share. So because we have that very lovely problem of 14 different ERP systems, over 50 line of business applications that we try and all centralized in Snowflake. That means we do have singular pipelines that are high quality. And then the data is in Snowflake, still of high quality. We're managing it effectively. So that's a central place now when we reach and have conversations with external parties that we might be looking at their solutions for , ots “are they a Snowflake customer? Can we do a direct Snowflake data share with you?” Cause we already have the data there. Most likely we already got a normalized to some degree and it'd be quicker for us just to create a derivative off of what we've already done and share it with you directly via Snowflake, rather than having to do some type of reverse ETL off the platform, configure an API interface and go through additional hoops that there's going to be additional points of failure.
Only quality can degrade, and it was ultimately costing us more computing and processing to add to that.
Kelly Kohlleffel (17:58)
Really interesting. You mentioned Snowflake and the data share collaboration, are you seeing in the agribusiness space, more opportunities with organizations? Maybe it's trading partners of CHS that are starting to come to the table, whether it's within the Snowflake marketplace or as a private data share offering those up, you mentioned there's four or five right now. Do you see this expanding significantly over the next, say 12 to 24?
Riley Buss (18:25)
Current signs are that they are going to be expanding. If we look at a year ago, we had one that we were kind of having discussions with, and now that's ballooned up to five. So that's been really kind of nice to see, just because it shows that the investment in that space is paying off. We're able to kind of create a unique relationship with some of our B2B counterparts to ensure that we can deliver on use cases quicker, faster and more scalable.
Kelly Kohlleffel (18:50)
You're removing a lot of friction from the process as well, which is really nice. Give me faster time to whatever that value-based thing is that I'm trying to deliver or develop.
If you look back where you are right now, you say, “Hey, here's some advice.” If you're looking, whether you're in the agriculture industry or not, but someone looking to modernize their approach to delivering data services, data outcomes and data products, the best advice that you could give to that person or people.
Riley Buss (19:17)
Yeah, so for us, it's kind of defining, like how are you defining value and success for the products you build? And making sure that you're adhering to that as nicely as possible. And a couple of different ways to do that, especially with some companies, there's still a lot of folks that are dealing with some legacy data platforms, and are working on migrating towards more cloud-based ones. What really kind of made it easy for us to migrate and how to do that successfully was we focused on enhancing our processes.
So on Cloudera. We had, there's a lot of learnings that we had from, you know, working one with on-prem storage, but two, it was also the first time that we had a data lake at CHS. So there's a lot of learning of “how do we best handle this.” So what we wanted to do was create a better avenue for our citizen developers, for our actual data engineers, to make sure that there was a benefit for them moving to the cloud. So if you can make it, for the people that need to do kind of their core work for doing that migration, if you can make it a benefit for them doing it, that was very successful for us.
Kelly Kohlleffel (20:12)
What qualities for you, Riley, are most important to have when you're leading a data team and building a data team?
Riley Buss (20:19)
Yeah, I'm very big on servant leadership. I came up through the development ranks. So it's very easy for me to kind of think of, well, this is how I would do it. So that's how we should create the standard to do it. That doesn't lead to the best kind of team morale or the best kind of collaborative thinking to make sure that we're thinking out of the box and thinking more futuristic as well. It's nice jumping into those discussions, empowering the data engineers, data scientists and business users to really kind of collaborate and come up with what's going to be best for us and also what are we gonna enjoy living in that we all can agree upon. So kind of fostering that collaborative culture is the biggest thing that I try to do from a leadership perspective.
Kelly Kohlleffel (20:59)
I love that. If you're, if you're hiring somebody onto your data team, anything that you want to comment on, like is there one or two characteristics or qualities that you look for and you go, “Ooh, that could be an ideal CHS addition to the data team.”
Riley Buss (21:14)
Yeah, definitely looking at kind of just aptitude, drive, ability to communicate and partner with the business. The technical side, we can kind of coach up and skill up on that, but the ability just to kind of lean into working directly with business users, understanding their process, the challenges that they're facing and then partnering with them on technical solutions, that's really where we see the best kind of mix of both worlds there.
Kelly Kohlleffel (21:40)
Do I have to have an agriculture background or can that be taught as well? What do you generally look for?
Riley Buss (21:47)
No, that can be taught as well. That's the kind of fun beauty of it too, is just having the more of the inquisitive nature of wanting to learn what you're building, not just building it for the sake of building it. That's really the differentiator.
Kelly Kohlleffel (21:58)
I love it. Riley, thank you so much. This has been a lot of fun. I think I could easily spend a couple of hours on some of these topics. Thank you so much for joining the show today.
Riley Buss (22:08)
Yeah, thanks, Kelly. Appreciate you having me.
Kelly Kohlleffel (22:10)
Absolutely. Look forward to keeping up with everything that's going on at CHS. Really, cool stuff. Hey, a huge thank you to everyone who listened in. We really appreciate each one of you.
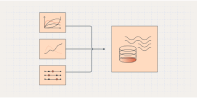
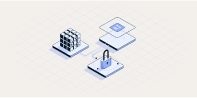
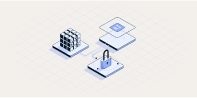