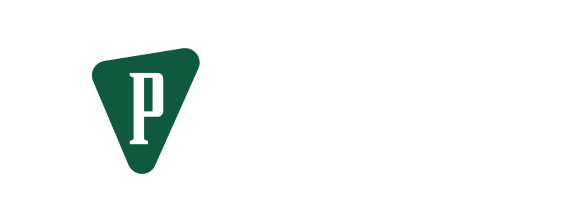
Data democratization and readiness for AI
Ajay Bidani, Data and Insights Manager at Powell Industries, shares his perspective on how a strong, inclusive data culture is fueling the manufacturer’s global success.
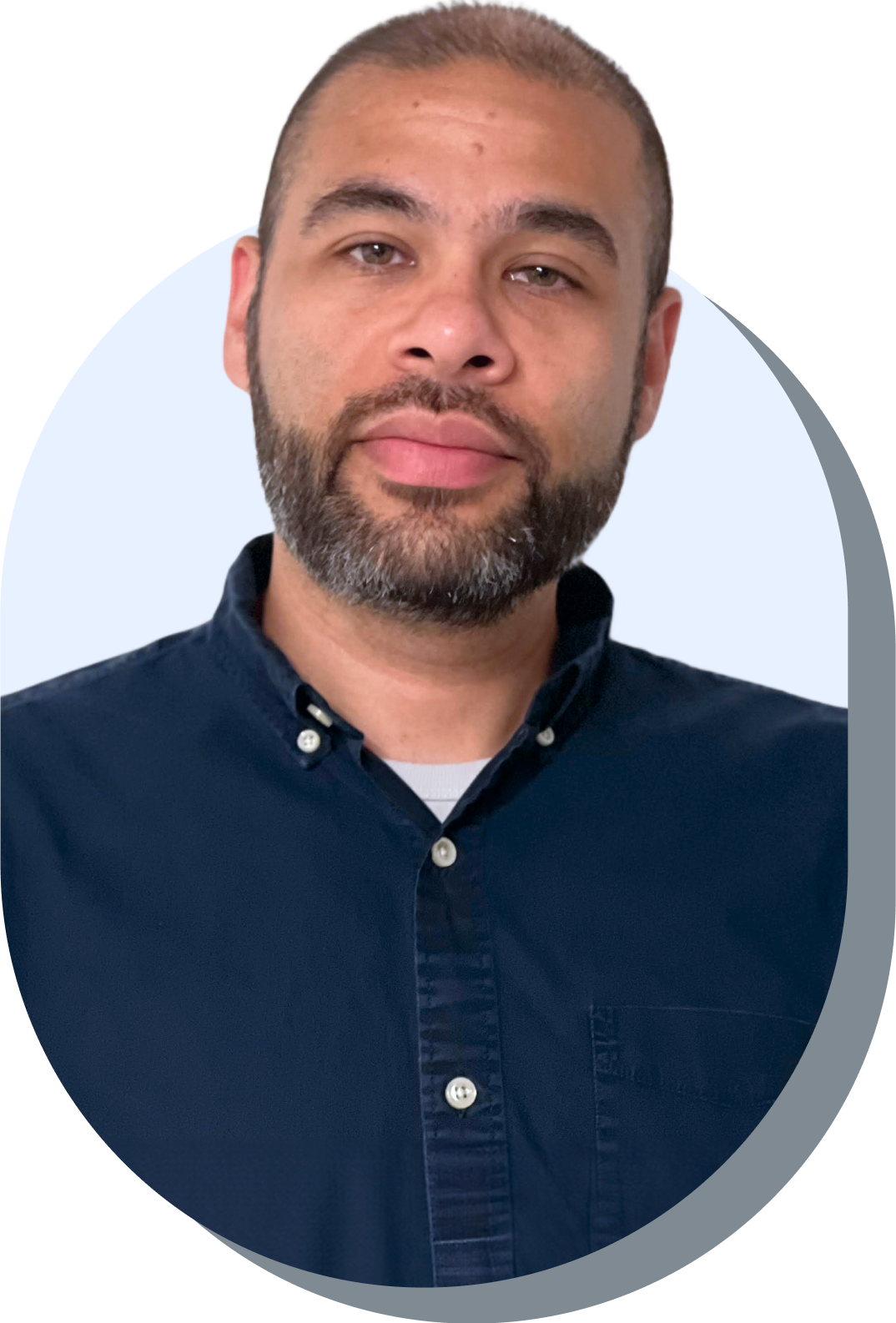
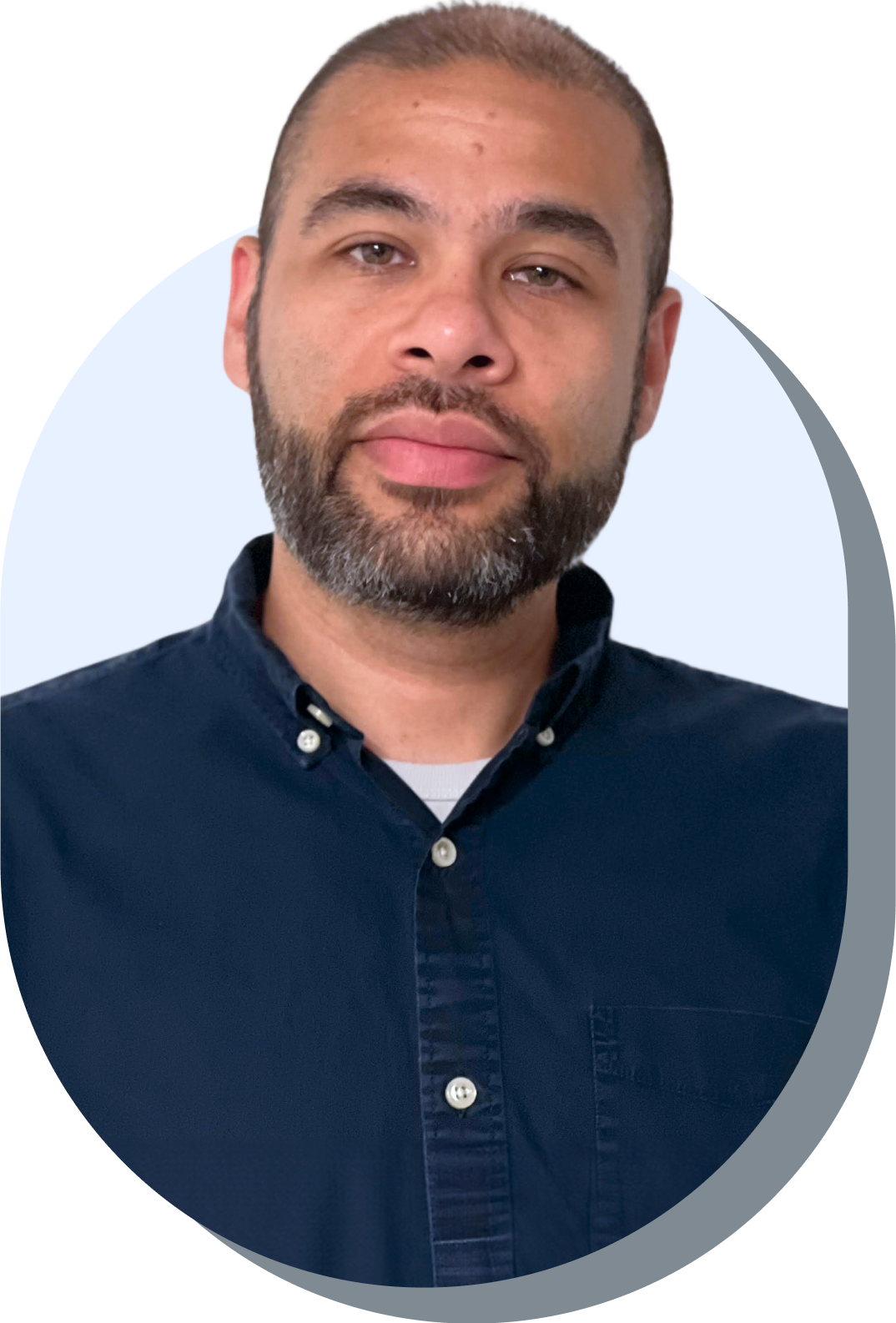
More about the episode
The key to breaking down data silos and fostering innovation goes well beyond having the right technology. It’s the people and processes that truly drive change.
“In our journey to an inclusive data culture, we realized it’s more than just data access; it’s about embedding a data mindset across all levels,” adds Bidani. “This shift not only enhances our efficiency but also transforms our organizational culture towards data-driven decision-making.”
In addition, it’s not simply the adoption of GenAI, ML or any particular technology, it’s the deep understanding of the problems and the data that underpins Powell’s systems.
“The thing that being in manufacturing teaches you the most is that most problems with AI will always start with a data problem. Do you have enough data?” With the right data in place, teams can develop a deeper understanding of the challenges (and how to solve them) — a major theme for Bidani’s profession and strategic outlooks for 2024 and beyond.
Dive into the conversation for insightful takeaways on:
- Challenges and triumphs of data democratization and how it influences data readiness for AI
- The importance of problem-solving and critical thinking skills in data management
- Finding the right balance between specialist vs. generalist roles on data teams
Watch the episode
Transcript
Kelly Kohlleffel (00:06)
Hi folks, welcome to the Fivetran Data Podcast. I'm Kelly Kohlleffel, your host. Every other week, we'll bring you insightful interviews with some of the brightest minds across the data community. We'll cover topics such as AI and ML, enterprise data and analytics, data culture and a lot more. Today, I'm really pleased to be joined by Ajay Bidani. He's the Data and Insights Manager at Powell Industries.
Powell designs and manufactures electrical systems for large industrial customers, such as oil and gas producers, refineries and more around the world. Ajay has spent the last 17 years at Powell, managing, developing and procuring enterprise applications. He's got a ton of experience here. More recently, his emphasis has been on performing technical alignment for time-saving automation and optimization and creating scalable architectures.
Ajay, it's great to see you again. Welcome to the show.
Ajay Bidani (00:58)
Thanks, Kelly, for having me. It's been a while.
Kelly Kohlleffel (01:01)
It has, it seems like we're going back. We've done this a couple of times together.
Ajay Bidani (01:06)
Yes, we have.
Kelly Kohlleffel (01:08)
I tell you what, for folks who haven't heard us before, why don't you start with a quick overview? I talked about Powell just a little bit, but maybe a little more detail on Powell Industries, what you guys do and then your current role at the company.
Ajay Bidani (01:21)
Sure. So we're a global company. We have three major locations in the US, Canada and the UK. We do manufacturing in all three. We have equipment all over the world. We recently shipped equipment all the way to the other side of the world and had to work through a project in which we actually had to do the construction there. So the description is pretty apt as far as what we do, but the ways in which you have to do it and the people you have to work with to do it have definitely changed over time. Trying to keep up with technology and trying to be competitive is not easy.
Kelly Kohlleffel (02:00)
Yeah, I've been around the manufacturing oil and gas industry a long time as well. Certainly, in those cycles that we go through in this industry, the need for data does not diminish during a down cycle. It certainly doesn't during an upcycle. You need it regardless of where the industry is overall.
I'd love to spend the majority of this time really digging in a little bit on – with your experience Ajay – building data teams, building the right data team as you go through migration and certainly modernization to do as much with your data as you can for Powell. Maybe to kick things off — how have you organized your data team? How's it organized today? Where have you come from when you started to where you are today?
Ajay Bidani (02:51)
Sure. When it comes to staffing, I'd say just having an experience with the business is a lot of what we tried to focus on.
In whatever way you were supporting the business, having that to bring to the table, so that way, the business sees you already as someone they can trust with the questions they have. And you're not finding that gap of being someone who's overly technical, who doesn't know how to talk to them about the things that they're facing. So, as we've gone forward, we were very much kind of a few people doing a lot and doing probably too much. And now, we've really tried to say, what are the core things that my team needs to be good at to make the business successful when it comes to data? And what are those capabilities that the business needs to be empowered to do more? Because they can do it better than us and they can get to answers faster, if we're really good at enabling them and they're really good at telling us what they're looking for. And we get that kind of collaboration process better than just going after it simply from a tools perspective.
Kelly Kohlleffel (03:47)
You've got your team within Powell, but how much is that notion of democratizing kind of spreading out? We've almost got these virtual team members across the business. I’m really interested in that from an organizational standpoint as well.
Ajay Bidani (04:00)
Right. I mean, most of us grew up on things like Excel and we were used to plugging the gaps for ourselves using Excel when it came to questions. So there are a lot of people who are already familiar with the idea of working with data.
It's just that those efforts are so isolated from each other, at times. I mean, some people are really great at it, but oftentimes they're very isolated. So there is a lot of duplicate work. There are a lot of duplicate questions being answered and there's not necessarily alignment by default, because two people aren't talking to each other to see if they feel the same way about things. We're just trying to improve the baseline for everyone when it comes to the tasks that they already do and the time that they spend. That really allows the cultural aspect of it to start to be more of the conversation, which is the, are we actually already data-oriented? We're just not as organized as we'd like to be in how we tackle a lot of those challenges.
And I think that that's really where that whole idea of getting more folks involved in the business starts, is just understanding what they might already be doing, but you just don't have the visibility to talk about it across the company the way you would if it was all coordinated from the center.
Kelly Kohlleffel (05:05)
Over the last four or five years, have you seen major shifts in how you think about data teams, how you think about data teams being democratized, even some of the roles that you have major shifts that you've seen in Powell Industries?
Ajay Bidani (05:25)
Definitely. You always start with the idea of who's going to be your partner in delivering something, especially if there's a question that needs answering. And you start by asking, trying to kind of narrow down, “who in the business is going to be really good at being responsive and going to kind of actively look to work with me to deliver this?” versus ask me to do something and kind of walk away until I've done it.
Kelly Kohlleffel (05:45)
Very nice. And your direct team, Ajay, what are some of the roles that you have today? I ask that question because a lot of times when I look at a team like yours, I'll see architects, engineers, analysts. It's kind of a group of different skill sets. How do you organize that today and what are those roles?
Ajay Bidani (06:02)
So for a while, I'd say we definitely started by saying, we want folks to just get their head around what it takes to be successful with data when you're kind of starting in this newer stack, where it was kind of fresh for everyone. But what you find is that with everyone trying to get a handle on too many things, no one has room to kind of grow within a certain set of capabilities. So what you find, and I mean, we found certainly more recently — that giving people room to grow in more kinds of finite spaces really does help, and it helps them better collaborate with other folks on the team. So, especially in more recent times, that's where we've kind of moved, is to better define what these roles are, and what their accountability is to what we're trying to do.
So, I mean, some of the names you described, analysts, engineers, but more just explaining where those lines are and what their objectives are a bit better. So, that way, it's like, well, “here's the thing we're trying to accomplish for the business”, but then, “here's your piece of that puzzle”, so that everyone kind of knows, we're not trying to compete with each other over the same things. We're trying to understand how we can each add the most value to getting towards that delivery. So, that focuses a bit on people, I don't want to say, narrowing their skills. That's not really the word I would use. It's more focusing on the things that they can do really well. So, that way, they can give feedback on whether this would help them do that job better.
So, to give you a good example, this has certainly been a more common topic in recent times. The idea of developer experience. Of course, we've got a terminology around a DevEx, right? But, developer experience, when it comes to data, has always been a thing, right? Because we've always been talking about those things that, for example, permeated DevOps and how we bring them into the data space. But in some ways, it's very true when it comes to the actual effort associated with developing things in the data space and figuring out what reduces the time it takes to put something together because you have those pieces together. Are you jumping between tools too much? Do you need to consolidate the experience some? And allowing people to spend more time doing some of those specific activities.
But, if they're hopping between activities and it's like multiple weeks in between, they kind of lose the ability to tell you what's actually working really well and what's not. So that's definitely something we focus on, giving people room to focus on those tasks more narrowly, versus trying to understand the whole picture all the time.
Kelly Kohlleffel (08:16)
I think for the individual too, if you can develop that level of specialization around this focus that you're talking about, but you also talked about room to grow if they have an interest in another area. As part of that too, I would imagine that they're able to, like if I'm a data engineer working with a data analyst team or working with an architect or working with a business analyst, understanding what they go through, I can develop a level of empathy for their role too, that hopefully helps me in my role.
In a business unit, is there generally one or two people there that are really attuned to working with your team that are easy to work with that don't, you know, sort of create waves, or is it more broad across those business units?
Ajay Bidani (09:03)
I know we'd all love to just simply train everyone perfectly for everything. It just really doesn't always work that way. So you have to find just kind of more like pockets where you can engage people more. And that's what we're trying to do with those business folks is give them ways that they can actually engage other people in their functions, so that they can kind of nurture that a little bit on their own versus us doing the direct nurturing of those people – if that makes sense. So we're trying to enable, again, even them to do that with other people.
Kelly Kohlleffel (09:27)
If you have a new team member joining your team, what are the tips that you would give them for being able to facilitate that with the business team? Like what are the two or three things that I really should make sure I'm doing? Conversely, what are the two or three things to never do with a business unit as part of the data team?
Ajay Bidani (09:45)
I think the hardest part about doing data, and I mean, I got this advice pretty often at the beginning of my journey with data, was this idea that if you've been used to supporting applications, which I've got a lot of experience with, you're used to thinking that your job is to keep everyone productive all the time, which I mean, is true. But when you're doing data, one of the biggest traps about data is that everyone in theory has some data problem. If you try to go into this thinking you're going to solve every single one. It's somewhat impractical.
In data, you really – just because of the way data works, the way the costs of those things work – you really do have to find the right places to start. And you will, if you gather enough momentum, you'll get to those things. If they're important, you will get to them. But if you start by approaching things from the perspective of, you're going to get to everything, just like you do if you're just supporting people day to day, that approach is just going to split you too thin.
And so, you're not even going to become really good at solving a problem, because you're just looking to kind of give everyone something. Does that make sense? It's kind of the difference between doing it as a hobby and doing it as your actual job. And I'd say that that's kind of one of the things I would probably impress upon at the beginning, is understanding the difference between just supporting people in their job, vs. supporting people with data, and how to actually facilitate that interaction better.
Kelly Kohlleffel (11:03)
I love that. As you were talking, I was thinking that, I think we tend to do that even on the architecture side. “Let me build this perfect architecture that's going to handle every use case, every scenario.” And to your point, those never practically end up getting built or working or really even being used, ever either. So no, I think that's, it's very valid. I have to ask you too, skill sets when you look at going into this year, what do you want to have on your data team? What are those one or two skills or maybe languages or things you need to know that kind of transverse across the team?
Ajay Bidani (11:45)
When it comes to 2024, I feel like open-mindedness about how you achieve what you're trying to achieve and that the language or being an expert in the language may not be the thing that's really essential. It's understanding how to design with it.
And how, for example, generative AI may help you in your journey in a way that just being an expert in that language doesn't by default help you solve. Does that make sense? It's kind of like, is the goal to learn the language or is the goal to be successful with the language?
Kelly Kohlleffel (12:19)
Solve a problem. Let's say I'm, I'm on Ajay's team. I'm not an expert in Python. Maybe I know SQL really well, but I've got to do something within the context of Python, that's going to help with the outcome I'm trying to deliver. Do you have team members who are starting to use GenAI in that sense to say, “Hey, help me out with this Python problem I've got, because it is ultimately going to help the company.”
Ajay Bidani (12:42)
Quite frankly, that's something that even when it came to one of my more recent hires, was one of the questions that previously I would say I didn't focus as much on. And that was something I was more interested in was, how much interest this person has to grow more in that space already. I mean, not just, “hey, if I come to your team, I want to”, it's more something I already have some interest in. And “if you give me an opportunity to explore that, I'm interested to explore that further”. Because for me, that's more, you already have some basic interest in it, regardless of what our needs are. But then, the question is, “can I expose you to more things that make you understand a path where you could be successful with it?”, and like they say, kind of crawl into it a bit? So, a lot of what I do, I confess, I'm sure others are different when it comes to this, if you have bigger teams, a lot of what my team does are things that – typically – are jobs that I am willing to do first, to try to figure out “what's the default experience need to be for doing it”. So, I'll freely admit, a lot of the questions that when you ask about GenAI are typically even to me,,“Where can I accelerate something for myself and actually solve this problem?”
And start using that to build some momentum in my team and interest within my team, because of how it helped me. Not taking it from the perspective of “it was easy because I'm an expert in this language and everything else.” It's like, “no, this is what I did know and here's what I didn't. And this set of steps helped me bridge that gap.”
Because, ultimately, GenAI is not going to be a “flip-the-switch and everything's fixed” type of thing. You have to find where it fits into your workflow, effectively. And there are lots of individual spots in the workflow where it helps. It's just a question of how do you make that experience fit that person's job without it feeling like, “I got to stop doing something I'm good at, try to do something totally different and when it doesn't work, I don't have a way to merge back into what I was trying to accomplish.”
Because I think for a lot of people, that's more of the part where they feel depressed by that idea, that could happen. I'm like, no, the idea is let's find a way that you can integrate it into how you're doing something. And if it works out great, if not, you can still kind of work through, for example, the idea of building the code yourself. But let's just find a spot where you could ask a question and see what kind of answer you start with.
Kelly Kohlleffel (14:58)
I think we could spend the rest of the show just on what you just talked about. There are a couple of things that stood out to me. Number one, I loved what you said because I'm a big proponent of this as well. I don't think you and I have ever really talked about this, but not asking your team to do something that you're not willing to do yourself or at least willing to try, right? You may not have the skills to do it, but give it a try. So, that really stood out to me when you said that. I think if I was on your team, I would very much appreciate that. I say, “look, Ajay's in there with us. He's in the trenches getting this done.”
Let me transition a little bit. When you're leading a data team, what are the key qualities that someone needs to have? Maybe it's somebody who's looking to move into a leadership role, move into a management role. What do you think based on your experience?
Ajay Bidani (15:42)
I mean, this sounds kind of silly, but it's true in a lot of spots, is that always be willing to listen, because sometimes the hardest part of trying to solve problems is that you can get so tied into the way you're trying to solve it, that you're just missing more about the problem than you might be realizing, or the ways that the problem could be solved than you realize. So, honestly, I would say, just being, always willing to listen.
Not because you might be totally off, but because there might be nuances you were missing about what it could take to solve something. And I think the idea is always to be willing to go a little bit deeper, to make sure that you're not just going at it like, “Oh, I listened, I mean, he said this.” I'm like, right, but almost like, see if you can reframe the question the way you heard it. I used to be really bad about that because I'm not someone who likes hearing things repeated, but sometimes I feel like just forcing myself to repeat it allows me to see if I actually got on top of it.
With everyone I work with, that's probably the hardest thing, is you get so tied into solving something that sometimes you forget that you might have missed some things and tied yourself down to a particular way of doing something that you didn't have to tie yourself down to.
Kelly Kohlleffel (16:54)
That's outstanding guidance, Ajay. I think for data leaders, individual contributors as well within, not only data organizations but any organization. I know we're starting to get to come up at the end of time, but I wanted to ask you. So Powell Industries, manufacturing, there's a lot of different types of manufacturing out there. I wanted to get your thoughts before we end, are there any particular challenges when you look at the manufacturing industry? From a data standpoint, uniqueness is there that you've got to address or that you need to be aware of.
Ajay Bidani (17:28)
You mentioned we're an electro-manufacturer. We don't mass produce a single design over and over as much per se. Like we do have some common designs, but we ultimately are kind of engineered to order, per a requirement.
So, what that means then is that, for any manufacturer, you're trying to figure out what's the most effective way to deliver. And for some, that means, okay, well, we'll do more of the steps in-house. Well, since we do not just, let's say, do the final assembly of some of the equipment, we also do the wiring of the equipment. We also do the procurement ourselves of the products we need. We fabricate the parts.
It's kind of like treating each one of things as its own ecosystem of data. And the struggle, sometimes, is thinking in terms of, what's the metric overall? There's a metric overall for the people who need to have the equipment produced. But, within that world of producing it, there are a lot of subsets of collecting data that you don't even realize people are doing in their own minds as a part of making decisions. So, you're not even realizing that there's more to drill down into. And unless you're actually watching them do it, and you ask them about it, you don't realize how much they might just be doing themselves, as a part of doing it. And there are ways that you could say, well,” are these data points we could actually bring to bear a slightly different way?”
And I'll go ahead and say this just in the bigger picture. The hardest part about AI, for many of us, is trying to understand what's the actual reach of it. Like, what of our problems are actually solvable with AI is sometimes a hard thing. I'd say that being in manufacturing teaches you the most is, most problems associated with AI still will always start with a data problem. Do you have enough data to start to answer this question? If you were a person trying to answer it, do you have enough data to answer this? If you don't even know how you'd explain what things might affect something in a basic way, you can try to, let's say, data science your way to a solution. But the only way you'll be able to articulate how the pieces fit is to understand, if you have enough data about it, to really understand why it works the way it does.
I would say in our area, that's probably the thing that I've learned is, there's a lot more data to be collected than we may realize, and it is going to take a little bit of that drill down in each area to spot what things we are not collecting, still, that we need to be collecting, even to have a chance at eventually realizing what AI might be able to bring to bear.
Kelly Kohlleffel (20:04)
Regardless of the data workload, whether it's AI or something else, I'm hearing you say, “I got to have that solid data foundation in place to deliver the data to help solve that.” That is outstanding. Hey, last thing, Ajay, we've talked about a lot here, but are there other technologies or, I don't know, approaches that you're thinking about in 2024 that you haven't tested, maybe you haven't tested out previously? Anything else that we haven't talked about that you'd like to mention?
Ajay Bidani (20:33)
The only thing I would probably say, and I'll say it's a combination of technologies. So one of the things you mentioned at the open as far as what I've been focused on is where there's room for consolidation, where there's room for things to kind of evolve. And I feel like in the area when it comes to being successful with AI, one of the things we always talk about is the idea of you're starting, for example, with an LLM and you have to do the fine-tuning, you have to do the training. And if you wanted to understand your organization, what does that take? Well, I feel like for years, one of the things, as part of my journey, was the understanding of where modeling really fits into that. And I will say that where we are is being able to articulate how all the modeling that you may have put off doing when it comes to your organization, AI is not going to be forgiving about the fact that you weren't willing to do that part. AI, actually, will deliver faster if you recognize the fact that you're literally trying to train someone. If you're trying to train a person to be really successful in your business, how would you do that? Right?
If you feel like you're going to get to AI without being able to understand how to train it, like really train it on what your business does and what things it would take to do that. So, I would say that, especially when it comes to – and I look at the field of enterprise architecture – that knowledge about how your business works is something that you need to be successful in operating your business anyway. If you can kind of connect that up to the long-term objective of how you can use that to train an AI, I want to further explore what that could mean, because I'd say the one part about that's not clear to me is, “how are you storing what you've captured in the enterprise architecture and in that model to make it useful for AI?” I don't know exactly what that needs to look like, from a modeling perspective, but understanding that, I think, is probably, at least for us, and maybe for several others, one of the key things to understand is, “what does your data journey need to include, if you ultimately want to see long-term success with AI?” As we were talking about, there are a lot of smaller places you can be with things like generative AI, but there are a lot of other AI opportunities. And this is one that I feel has been critical in the data community for a long time. It's understanding why it's important. And I feel like maybe AI will help remind us why it's important when it comes to that trajectory that most companies end up going on to be successful.
Kelly Kohlleffel (23:00)
Love it. Ajay, you’re insightful as always. It is always a pleasure to speak with you. Thank you so much. I really appreciate you joining the show today.
Ajay Bidani (23:10)
It was great to get a chance to talk about this stuff. Honestly, so much happens, right? So this has been great. Thank you so much.
Kelly Kohlleffel (23:14)
I know. Yeah. I look forward to keeping up with all of that new stuff that you talked about going on at Powell as well. And thanks everybody for listening in. We really appreciate each one of you.
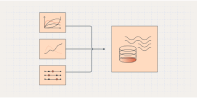
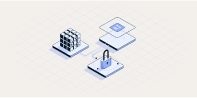
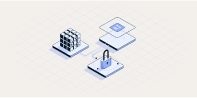