How Trellix turned data silos into a center of excellence
In this episode of the Fivetran Data Podcast, Frank Carotenuto, Senior Director of Enterprise Data Platforms at Trellix, shares his experience leading the organization’s migration to a single data platform from a data mart architecture.
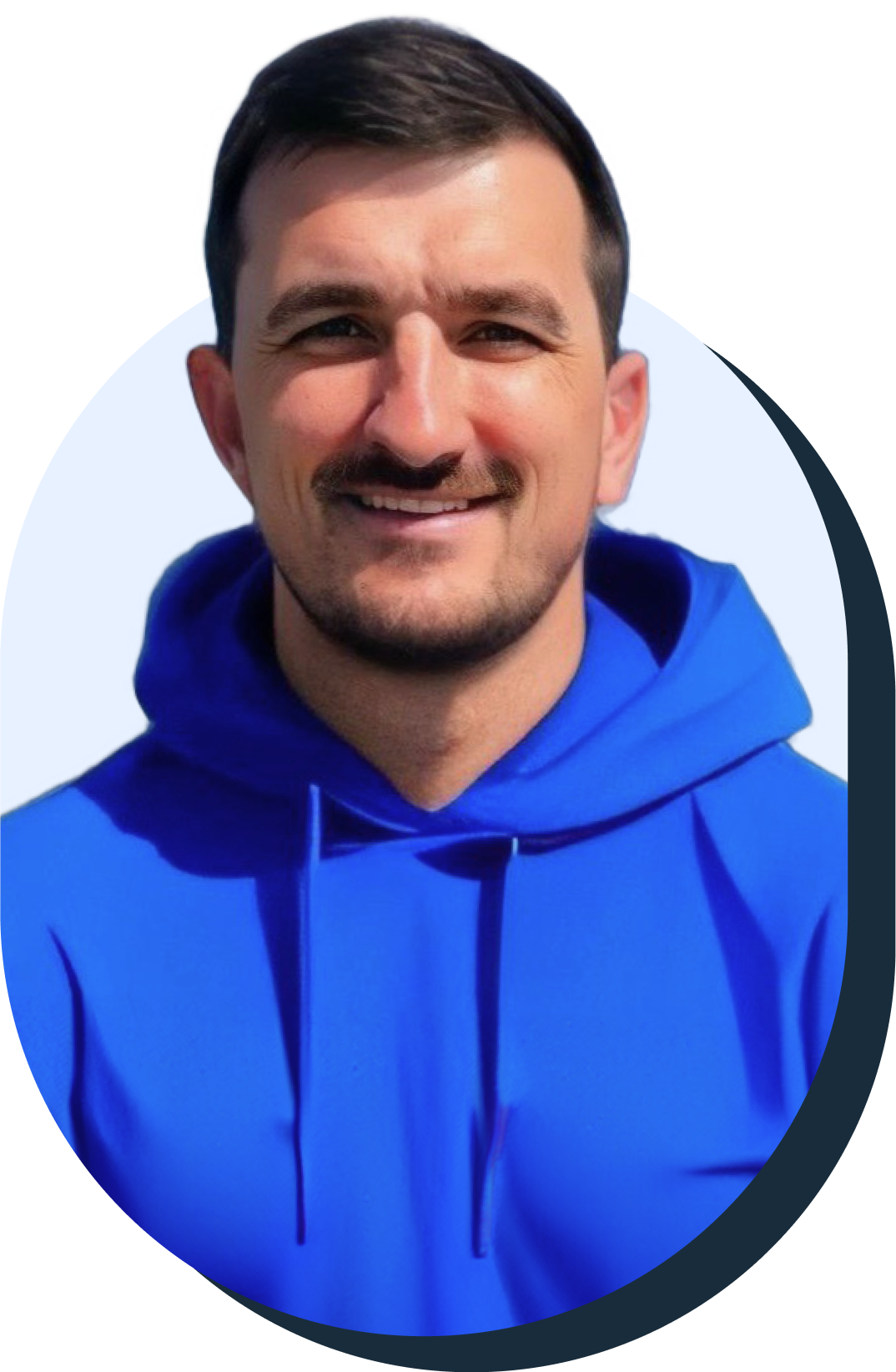
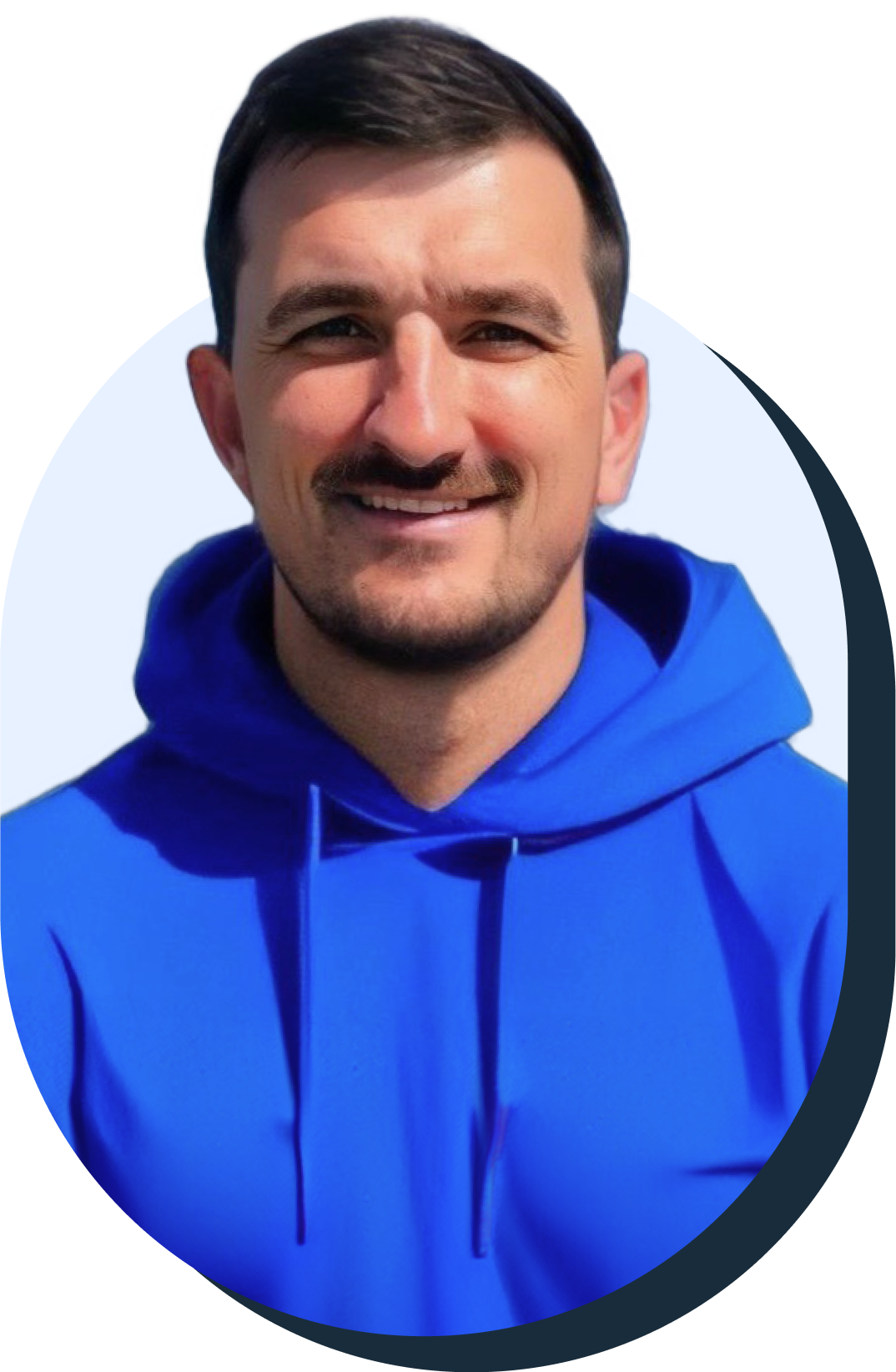
More about the episode
There’s only one thing that can stop your enterprise from building data silos: an enterprise data platform built with a modern tech stack. But the technology alone won’t tear down those silos — it’s the people and new processes that make all the difference.
In this episode of the Fivetran Data Podcast, Frank Carotenuto, Senior Director of Enterprise Data Platforms at Trellix, shares his experience leading the organization’s migration to a single data platform from a data mart architecture.
Carotenuto took the role shortly after McAfee and FireEye merged to form Trellix. He saw the opportunity to unite and guide data teams toward a new future where data was a first-class enterprise asset. His efforts resulted in a new team structure, modern data architecture and a cross-functional center of excellence (CoE) called the Data Council.
“When we ended up consolidating our data teams into a single CoE, they didn't lose the ability to individually prioritize. What we gained is the ability to reduce duplicative effort because we're able to align across business units.”
Here are the key highlights from the conversation:
- Managing people and rolling out new processes while building an enterprise data platform
- How to form and operate a cross-functional center of excellence built around data
- Shifting to a mindset where data is a first-class enterprise asset
Watch the episode
Transcript
Kelly Kohlleffel (00:06)
Hi folks. Welcome to the Fivetran Data Podcast. I'm Kelly Kohlleffel, your host. Every other week, we'll bring you insightful interviews with some of the brightest minds across the data community. We will be covering topics such as AI and ML, enterprise data and analytics, cloud migrations, data culture a whole lot more. Today, I'm really pleased to be joined by Frank Carotenuto. He is the Senior Director of Enterprise Data Platforms at Trellix. Frank has more than 10 years of experience in data engineering. He began his career at Nielsen and advanced to leadership roles at Footlocker and Iron Mountain. Now at Trellix, he leads data platform architecture and strategy. He's currently overseeing a pivotal cloud data platform migration. His expertise is in spearheading migrations to cloud-based solutions, and then seamlessly integrating the cloud services and technologies like Azure, Databricks, Apache Arrow and Google Cloud. Frank, it is an absolute pleasure to have you on the show today. Welcome in.
Frank Carotenuto (01:05)
Likewise. Excited to be here.
Kelly Kohlleffel (01:07)
Absolutely. Spend a couple of seconds and review Trellix for us, and then also your role at Trellix.
Frank Carotenuto (03:18)
We have about 40,000 customers and about $1.7 billion in revenue. That is because we're actually the merger of two other entities, so you might be more familiar with McAfee or FireEye.
I joined Trellix back in 2022 to lead our enterprise data platform. And as you mentioned, we immediately embarked on a pretty sizable roadmap to modernize our data stack. There are three primary teams that make up our enterprise data platform. We have two data engineering teams, one focused almost exclusively on master data management and data quality, specific to our account base and our customer base. Another data engineering team focused on data acquisition, ETL, dimensional data modeling and then a BI team that's focused on building out explorable data sets and dashboards for our end users.
Kelly Kohlleffel (02:17)
When you joined, were the teams formed up, or have you kind of crafted them over the last couple of years as you've been there?
Frank Carotenuto(02:23)
They're under one umbrella, but they are three separate functions. I ended up splitting the data analytics services team into a team that's focused on data warehousing and a team that's focused on business intelligence. And the teams that we formed together ended up growing over time. Throughout last year and the year prior, as we started to build a data platform and move away from more of a hub and spoke, or we were a hub or a distributor of data to multiple data marts throughout the organization to an enterprise data platform, we started to acquire more teams, which ended up growing our existing ones.
Kelly Kohlleffel (02:56)
I think of something like the data mesh concept where I'm driving down accountability and responsibility around a particular data product or set of products in the business. How do you think about that at Trellix today?
Frank Carotenuto (03:09)
Treating data as an enterprise asset is really foundational. Ensuring the accuracy of your data, having data quality checks in place, having well-governed data sets and ensuring your data is compliant with regulations. Really, only once you have this foundation, you can start to treat your data as a product, because if your foundational data is flawed, your products are gonna inherit those flaws. So by focusing on data as an asset first, you create a stronger foundation for building data products.
We shifted our focus last year to focus on building a data platform. One of the key things that we implemented to create self-service was to build out an enterprise semantic layer.
We chose Looker. We have our data warehouse team, our master data management team and then that BI team. One of the core responsibilities that they have is building out those Looker explorers, those reusable data sets for all of our business to consume. What that enterprise semantic layer – I'll demystify that a little bit. In lay terms, you know, that just pre-defines all of your joint conditions across all of your data sets in your data warehouse or data lake. Essentially what that's doing is doing all the heavy lifting for your end user. You know, they don't have to know how a measure is calculated because the definition is baked into your enterprise semantic layer. You don't need to know how to join data sets across different systems or even centralize the data. It's already done. Same with data quality checks and things like that. It's all in place.
Kelly Kohlleffel (04:53)
Where are you going as it relates to cloud migration and cloud modernization?
Frank Carotenuto (04:59)
Yeah, absolutely.
I think most cloud migrations, if your motives are well, if you understand why you're going to the cloud, I think that's what's the foundation of a successful migration.
So, we focused on all aspects of our data stack. Everything from data acquisition, to how we process our data, to how we present it to our customers, ultimately our stakeholders.
Frank Carotenuto (05:30)
So the first thing was that we made a bit of a paradigm shift, right? So we went from an ETL-based solution with Informatica, where Informatica did all the data acquisition and did all the data processing, and then it persisted just what was then used as the output, right? To an ELT model where using solutions – Fivetran specifically – to synchronize all of our data to our data platform on BigQuery. And then instead of doing the transformations outside of the warehouse, we did the transformations within the warehouse, using dbt. Now, a lot of value comes out of this, right? So for one, we can increase the cadence at which we acquire the data. It could be, you know, with Fivetran every couple of minutes. Being able to synchronize full data sets to our data platform offered us agility as well.
In my experience, a “lift and shift” is the most effective way to truly migrate. You know when you're talking about migrating a data analytics platform that is built over – I mean, not lift and shift from like, just like taking your Informatica appliance, throw it in the cloud, but like, you know, in terms of all of your ETL pipelines, your dimensional data model – taking that in its entirety, is not a bad move. But in terms of the reports that you have to recreate on top of that, then I think that's where you can start to chip away at, you know, “is this dashboard still relevant? Are people using it? Are they using this for the right purposes?”
Kelly Kohlleffel (06:59)
Yeah, I mean, if you take a lift and shift approach, usually I think about that as having a little bit less risk obviously than, you know, let's just blow everything up and redo it. Less risk. And usually a shorter time. What I like about that too, like if you have a new, let's say a business unit comes up with a new requirement that's not part of the current architecture or platform or solution, “Hey, start piecing that in under the approach you eventually want to get to once that lift and shift has been completely modernized.”
Frank Carotenuto (07:29)
Yeah, absolutely. As you're going through your migration, you're gonna have new demand come in. You can't put the pen down for six to nine months as you're going through migration. You have to continue to deliver. So it is certainly important to have your architectural patterns in place and establish first and then migrate towards those. Because what you don't wanna do is just rebuild every solution. Right? You don't wanna start with your end products and try to reproduce them. From my experience, what you'll end up with, is you'll have your cloud environment, your net-new data platform, but then you'll still have your legacy, maybe on-prem data platform that's still running the business that's still essential to the business. And now you have two separate teams doing two separate things, and you never have the ability to consolidate. So like the lift and shift is critical, but it's also really critical to have those architectural patterns in place so you can support those new use cases.
Kelly Kohlleffel (08:25)
How do you keep continued alignment with the organizational objectives that you have, your data program objectives and then the ongoing objectives and business KPIs that Trellix has?
Frank Carotenuto (08:41)
When we started our enterprise data platform initiative and centralized many of the analytics teams, we didn't want to silo-off that communication with our business units. We ended up forming an adjacent team to our enterprise data platform, and we call it the data council. And the uniqueness of this approach really lies in the composition of the team. We selectively sought a representation from every business unit, someone who might have been a previous leader of the analytics team that was focused exclusively on, maybe customer success or finance or sales operations. We appointed them as a data product owner, and they would effectively continue with their role and be immersed in their day-to-day, but they would also serve as the conduit to funnel new demand and prioritization to our team.
You know, in their day-to-day they'll stay immersed in their business unit. But they would also be responsible for understanding what their needs are, translating that into a data product and then breaking that down into our functional teams. So an example, if new demand were to come in, they would assess whether or not that would require new data acquisition and they would funnel certain demand into the data warehousing team to go get that data. If all data is available, they would just focus, they would just work with the BI team to build out a new dashboard or some reverse ETL pipeline to funnel data into an application or something like that.
Kelly Kohlleffel (10:18)
Yeah. That makes a lot of sense. And, that domain expertise obviously will vary from function to function. I would think that maybe a common thread on that data council is that pretty much everybody's gotta be pretty passionate about what data can do for their individual business unit as well.
Frank Carotenuto (10:38)
Absolutely. You'd be surprised by how much of the data needs across different organizations align with the needs of sales, product and finance. They have a lot of the same needs. So, when we ended up consolidating our data teams into a single CoE into a single enterprise data platform team, they didn't lose the ability to individually prioritize. What we gained is the ability to reduce duplicative effort because we're able to align across those business units.
Kelly Kohlleffel (11:11)
Yeah, that makes a lot of sense. When you think about data council, but even outside of that, what skills for you, what's most valuable for building a data team? What do you look for this year in 2024, as you're hiring or growing or just trying to help your individual team improve as it stands right now?
Frank Carotenuto (11:33)
There are different avenues in which people enter the data engineering space, and there's no bad one. I typically like to see somebody with an alternative programming background. They don't have to have it, but it's a very valuable skill. Or any programming language really. Having a CS background is pretty advantageous. Specifically because, you know, like for data warehousing, for example, our data warehousing team integrations team, you might need to build a bespoke API solution, either for another team to consume data off the platform or to retrieve data from another service. And having that skill in-house in the team creates that autonomy for us. You know, a solid foundation in programming obviously, SQL skills, but sometimes I even like to see networking skills. I like that because you'd be surprised how often that's used on a team, you know, if specifically with building data integrations, being able to troubleshoot connectivity to a server.
I always say that establishing connectivity to an application is like 80% of integration, specifically with solutions like Fivetran, you know, it, you really boil it down to establishing a connection.
And then lastly, what's really evolved quite a bit is a lot of the more modern data applications, like you juxtapose Informatica versus Airflow and dbt. You know, in Looker, a lot of these are a structured code base. So having DevOps skills, CICD deployment automation, and version control skills, are pretty important when we're looking.
Kelly Kohlleffel (13:30)
So translating, I'll call it professional software development skills into that data space is something that you really value.
Frank Carotenuto (13:35)
Absolutely.
Kelly Kohlleffel (13:36)
Cool. What about somebody looking to move into a data leadership role? In your experience, what's most important to have from a quality standpoint when you lead a data team?
Frank Carotenuto (13:48)
You really have to constantly check that the metrics you produce and the measures you produce are still relevant to a business need. You have to constantly keep yourself in check that the measure that you have still has a strong correlation to the outcome that you're using that metric for. Because, you can reduce a lot of wasted effort.
Kelly Kohlleffel (14:12)
Well, I really appreciate this. This has been outstanding, Frank. Thank you so much for joining the show and I'm looking forward to keeping up with everything that you're doing at Trellix.
Frank Carotenuto (14:21)
Awesome. Great chatting with you.
Kelly Kohlleffel (14:24)
Yeah, fantastic. And for everybody who listened in today, thank you so much. We appreciate each one of you. Take care.
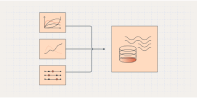
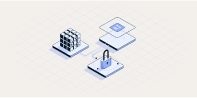
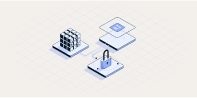