Think back to the last time you returned an item you bought online: Maybe that colorful pair of sneakers didn’t quite fit, or that splurge-worthy jacket arrived missing a button. When you sent it back for an exchange or a refund, that item’s complicated return voyage through warehouses and supply chains generated a wealth of data points. Multiply that across the countless items returned every day as more and more of us shop from home, and you’ll quickly realize there’s an ocean of data out there to manage.
That’s the issue that excites the team at Optoro, a Washington, DC–based technology company whose platform connects returned items to their next best home — ultimately saving customers money and helping the environment by reducing waste.
At our recent Data Engineer Appreciation Day conference, Optoro Lead Data Engineer Patrick Campbell noted that as returned inventory is routed through various systems, it generates large amounts of operational data. That data is mission-critical for Optoro, but until recently the company had little visibility into its quality, and struggled to build trust with the end users who most needed it.
“We lacked insight into the quality of our data, plain and simple,” Campbell said. “We didn’t have a good method for understanding when data might be missing, when it might go stale, or if the data wasn’t what we had expected.”
As the Optoro team began sketching out solutions, they realized that automating data observability could solve the problem.
What is data observability and why does it matter?
Just like software engineers rely on observability solutions to measure the health of web applications, data engineers think of data observability as a way to monitor data pipelines and alert for issues. Although standard testing and checks have always been a best practice, new automated solutions use machine learning to learn a company’s unique data patterns over time and better detect anomalies. By monitoring a company’s entire data ecosystem, this new generation of solutions can detect problems and notify users right away.
This proactive approach to quality assurance can have a meaningful impact on a company’s bottom line, given how devastating downtime can be. In a recent survey from Dimensional Research, two-thirds of data engineers said pipeline breaks reduce operational efficiency, while 59% said they delay important decisions that can lead to lost opportunities or competitive disadvantage. Nearly 40% of companies say customer satisfaction suffers when data goes down.
Optoro’s Campbell also noted how issues like these further deteriorate trust among internal stakeholders. “If we have downtime, it’s a pretty major cost to our clients, which isn’t sustainable.” When things go wrong, he said, “this produces mistrust in our data and mistrust in our ability to deliver reliable information about the inventory our software manages.”
A modern data stack with built-in observability
Optoro found its solution in a modern data stack using automated, cloud-based tools that reliably gather data from a multitude of sources:
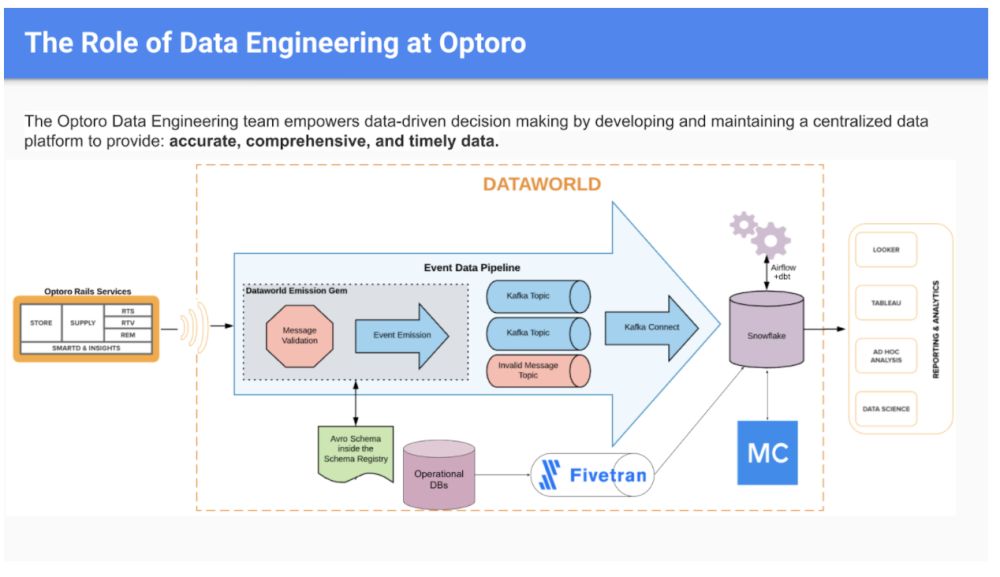
A Fivetran pipeline connects data from operational databases to a Snowflake warehouse, with data clients using Looker, Tableau and ad hoc analysis tools to visualize and generate insights. Monte Carlo, an automated data observability tool, is there to learn Optoro’s data patterns and alert the right people when things break.
Campbell says his team especially appreciates their newfound ability to trace lineage. In other words, when something breaks, they understand at a glance which databases, reports and users are affected downstream. They can also quickly see which upstream sources could have contributed to the problem. “We can get a visual on the affected data sources, from data marts all the way down to Looker reports that can be client-facing,” he said.
And it makes a data engineer’s job that much easier, he added: “Not only is this a win for data engineering in terms of being able to track down needle-in-the-haystack issues, but being able to enable other data teams to help us keep trust in our data.”
Since prioritizing data observability, the Optoro team has saved about four hours of work per data engineer per week. Most importantly, they continue to build trust throughout the company and gain confidence in their data. As Campbell put it, “Data integrity really should be self-service, and your data engineers will thank you.”